Differentially Private Federated Learning With Stragglers' Delays in Cross-Silo Settings: An Online Mirror Descent Approach.
IEEE Trans. Cogn. Commun. Netw.(2024)
摘要
Federated learning is a privacy-preserving machine learning paradigm to protect the data of clients against privacy breaches. A lot of work on federated learning considers the cross-device setting where the number of clients is large and the data sample size of each client is low. However, this work focuses on cross-silo settings, where clients are few and have large sample sizes. We consider a fully decentralized setting where clients communicate with their immediate time-varying neighbors without the need for a central aggregator prone to congestion and a single point of failure. Our goal is to address stragglers’ delays in cross-silo settings. Existing algorithms designed to overcome stragglers’ delays work with fixed data distributions. They cannot work in real-time settings, such as wireless communication, characterized by time-varying data distributions. Therefore, this paper proposes two online learning algorithms that work with time-varying data and address stragglers’ delays while guaranteeing differential privacy, strong convergence, and communication efficiency. Using the mirror descent technique, the first proposed algorithm addresses the case where the loss gradient is easily computed while the second proposed algorithm addresses the case where the loss gradient is difficult to compute. Simulation results show the performance of the proposed algorithms.
更多查看译文
关键词
differential privacy,federated learning,mirror descent,online learning,regret
AI 理解论文
溯源树
样例
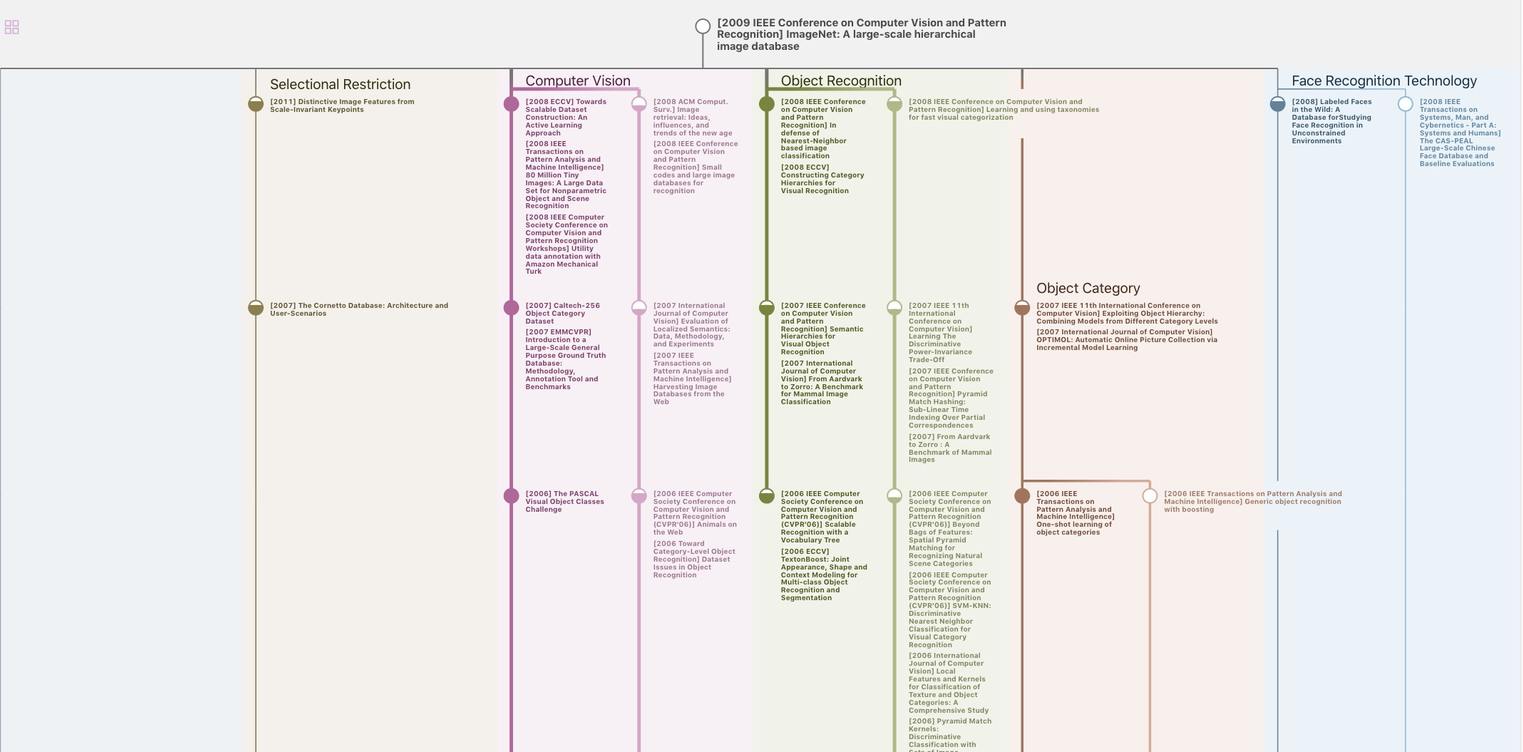
生成溯源树,研究论文发展脉络
Chat Paper
正在生成论文摘要