Dynamic Relation Graph Learning for Time-aware Service Recommendation
IEEE Transactions on Network and Service Management(2023)
摘要
Driven by Service-Oriented Computing, time-aware service recommendation aims to support personalized mashup development, adapting to the rapid shifts of users’ dynamic preferences. Recently, users’ social connections have shown significant benefits to time-aware service recommendation, and graph neural networks have demonstrated great success in learning the pattern of information flow among users. However, the current paradigm always presumes a given social network, which is not necessarily consistent with the similarities of service preferences among users and is expensive to collect for most service platforms. We propose a novel idea to learn the graph structure among historical mashups and make time-aware service recommendation for dynamic mashup creation collectively in a coupled framework. This idea raises two challenges, i.e., scalability and accuracy. To solve both challenges simultaneously, we introduce the Dynamic Relation Graph Learning (DRGL) framework for time-aware service recommendation. For scalability, our framework has a coarse-to-fine recalling strategy to learn the graph structure among the mashups, which enables the exploration of potential links among all historical mashups while maintaining a tractable amount of computation. For accuracy, we leverage recent advances in self-attention mechanisms to the mashup modeling and propose a transformer-based mashup encoder, which considers long-range dependencies in dense mashups for more accurate mashup representations. Extensive experiments show that the DRGL model consistently outperforms the state-of-the-art methods in terms of prediction accuracy for mashup creation.
更多查看译文
关键词
Service recommendation,graph neural networks,social network,time-aware,mashup creation
AI 理解论文
溯源树
样例
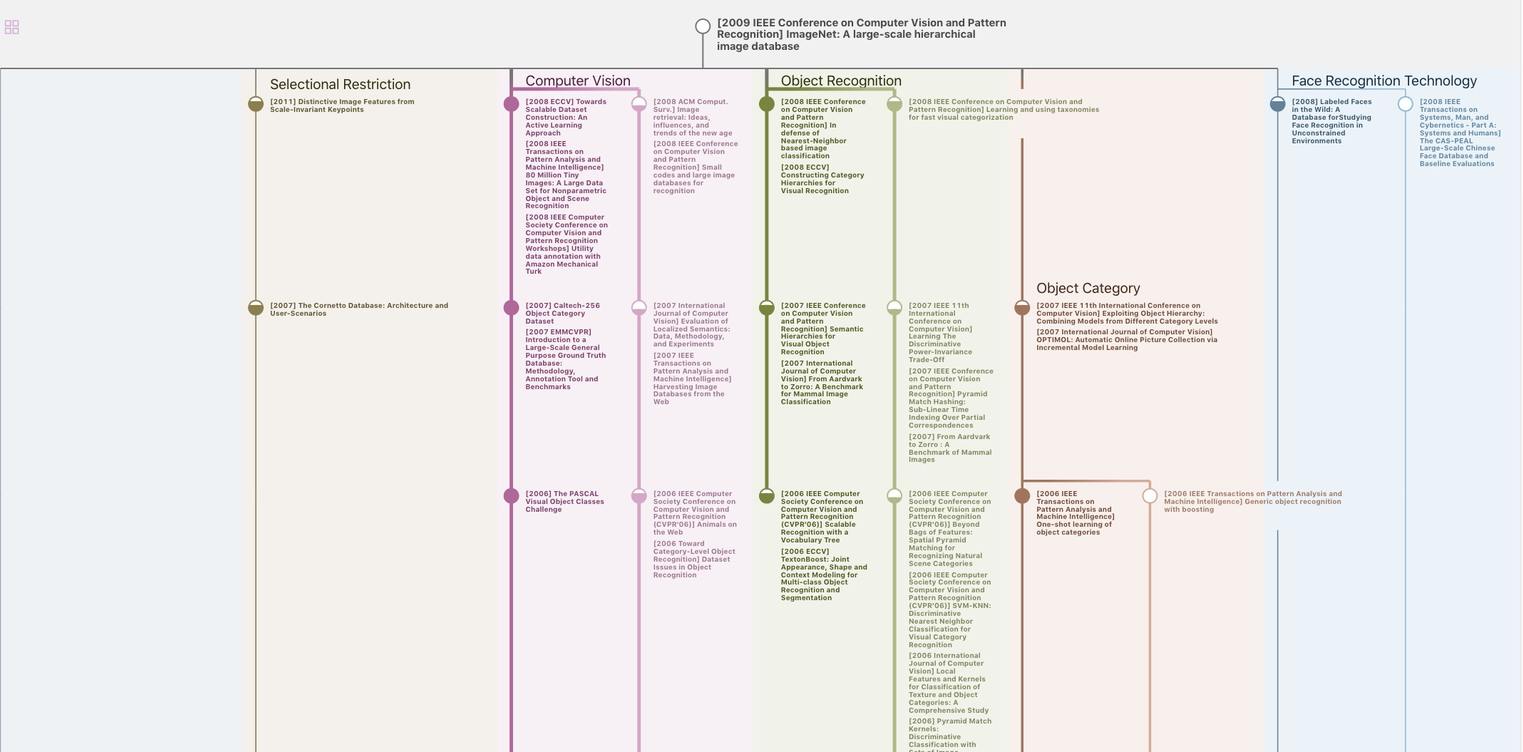
生成溯源树,研究论文发展脉络
Chat Paper
正在生成论文摘要