Evidential Meta-model for Molecular Property Prediction
Bioinformatics(2023)
摘要
Abstract Motivation The usefulness of supervised molecular property prediction (MPP) is well-recognized in many applications. However, the insufficiency and the imbalance of labeled data make the learning problem difficult. Moreover, the reliability of the predictions is also a huddle in the deployment of MPP models in safety-critical fields. Method We propose the Evidential Meta-model for Molecular Property Prediction (EM3P2) method that returns uncertainty estimates along with its predictions. Our EM3P2 trains an evidential graph isomorphism network classifier using multi-task molecular property datasets under the model-agnostic meta-learning (MAML) framework while addressing the problem of data imbalance. Results Our results showed better prediction performances compared to existing meta-MPP models. Furthermore, we showed that the uncertainty estimates returned by our EM3P2 can be used to reject uncertain predictions for applications that require higher confidence. Availability and Implementation Source code available for download at https://github.com/Ajou-DILab/EM3P2. Supplementary information Supplementary Materials are available at Bioinformatics.
更多查看译文
关键词
molecular property prediction,meta-model
AI 理解论文
溯源树
样例
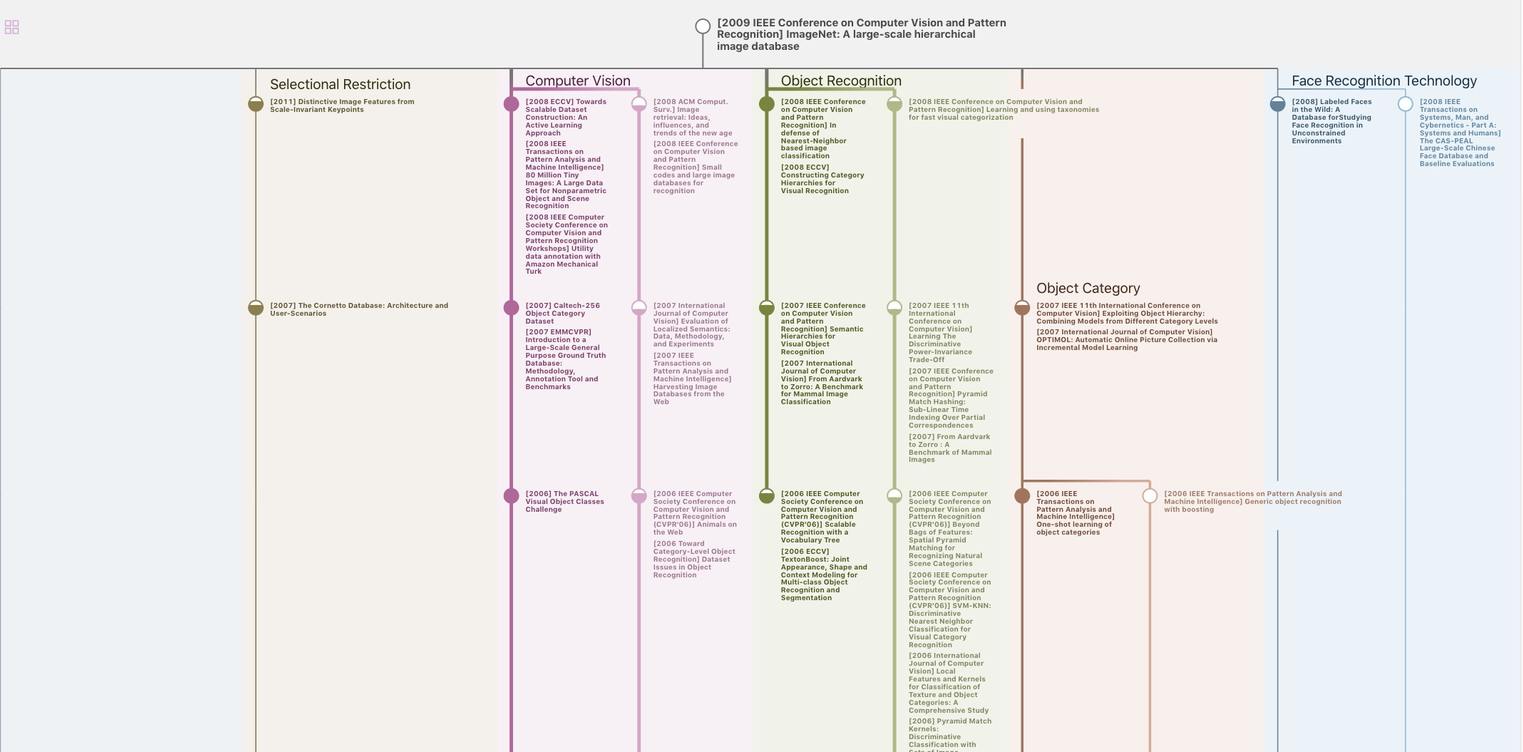
生成溯源树,研究论文发展脉络
Chat Paper
正在生成论文摘要