Dynamic Low-Rank and Sparse Priors Constrained Deep Autoencoders for Hyperspectral Anomaly Detection
IEEE TRANSACTIONS ON INSTRUMENTATION AND MEASUREMENT(2024)
摘要
Linear-based low-rank and sparse models (LRSM) and nonlinear-based deep autoencoder (DAE) models have been proven to be effective for the task of anomaly detection (AD) in hyperspectral images (HSIs). The linear-based LRSM is self-explainable, while it may not characterize the complex scenes well. In contrast, the nonlinear-based DAE is able to extract the discriminative features between the background and anomaly for the complex scenes, whereas it is not self-explainable. To effectively combine the advantages of both, a dynamic low-rank and sparse priors-constrained DAEs (DLRSPs-DAEs) for hyperspectral AD (HAD), in this article, is proposed. In order to utilize the low-rank prior existing in an HSI, a low-rank prior-based DAE (DAE_LR) is designed to generate an excellent background reconstruction effect and terrible anomaly reconstruction performance. Further, to consider the sparsity reflecting the anomalies in the HSI, a DAE that is constrained by the sparse prior obtained by the decomposition of the HSI (DAE_S) is developed. Notably, to make the model more compact, the DAE_LR and DAE_S share a common encoder. To achieve global optimal performance, an end-to-end joint optimization strategy with the consideration of the interaction between the learning of the DAEs and the decomposition of the HSI is proposed. Additionally, to yield better detection performance, a nonlinear fusion strategy is exploited to comprehensively combine the detection results obtained from both the DAE_LR and DAE_S. Extensive experiments conducted on several datasets show that the proposed DLRSPs-DAEs detector achieves tremendous performance with respect to the classical and state-of-the-art detectors.
更多查看译文
关键词
Anomaly detection (AD),deep autoencoder (DAE),joint optimization,low-rank prior,sparse prior
AI 理解论文
溯源树
样例
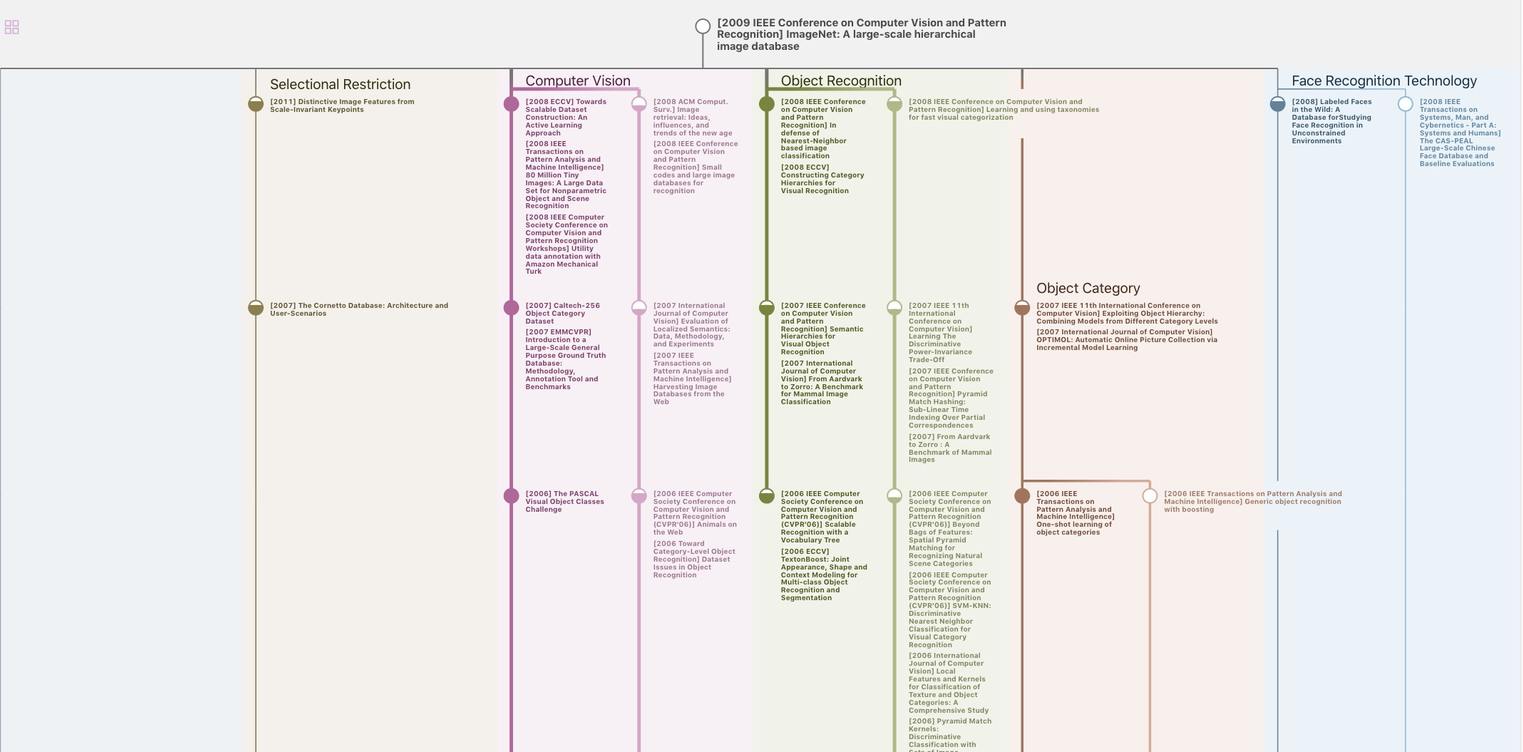
生成溯源树,研究论文发展脉络
Chat Paper
正在生成论文摘要