An AI system (SonoLyst) achieves expert level performance when categorising images for adherence to ISUOG mid-trimester screening guidelines
ULTRASOUND IN OBSTETRICS & GYNECOLOGY(2023)
摘要
To evaluate the performance of SonoLyst (GEHC, Zipf), an Artificial Intelligence (AI) based algorithm, in undertaking “peer review” of images required for complete routine mid-trimester ultrasound scans according to ISUOG guidelines. Multicentre prospective evaluation using real-world images captured from 4 sites during routine anomaly scanning. These were supplemented by non-optimal views extracted from preceding video loops to allow more robust testing. To replicate routine clinical image review, each image was categorised into the relevant ISUOG view, and then quality assessed for protocol adherence. Each image was assessed by AI and 4 clinical experts from a pool of 12, blinded to each other's results. Expert-expert agreement was compared to expert-SonoLyst agreement. Subanalysis by operator experience was undertaken. We assessed 12,476 images from 776 patients. Mean Expert-Expert agreement in categorising views was 79.4% (77.6% to 81.1%) and was indistinguishable to Expert-SonoLyst agreement of 79.6% (77.1% to 82.4%). SonoLyst is indistinguishable from an expert when categorising views of routine mid-trimester scans against ISUOG guidelines. Such software can support sonographers during routine scans to ensure completeness and quality of scanning by “real-time peer review” of captured images.
更多查看译文
AI 理解论文
溯源树
样例
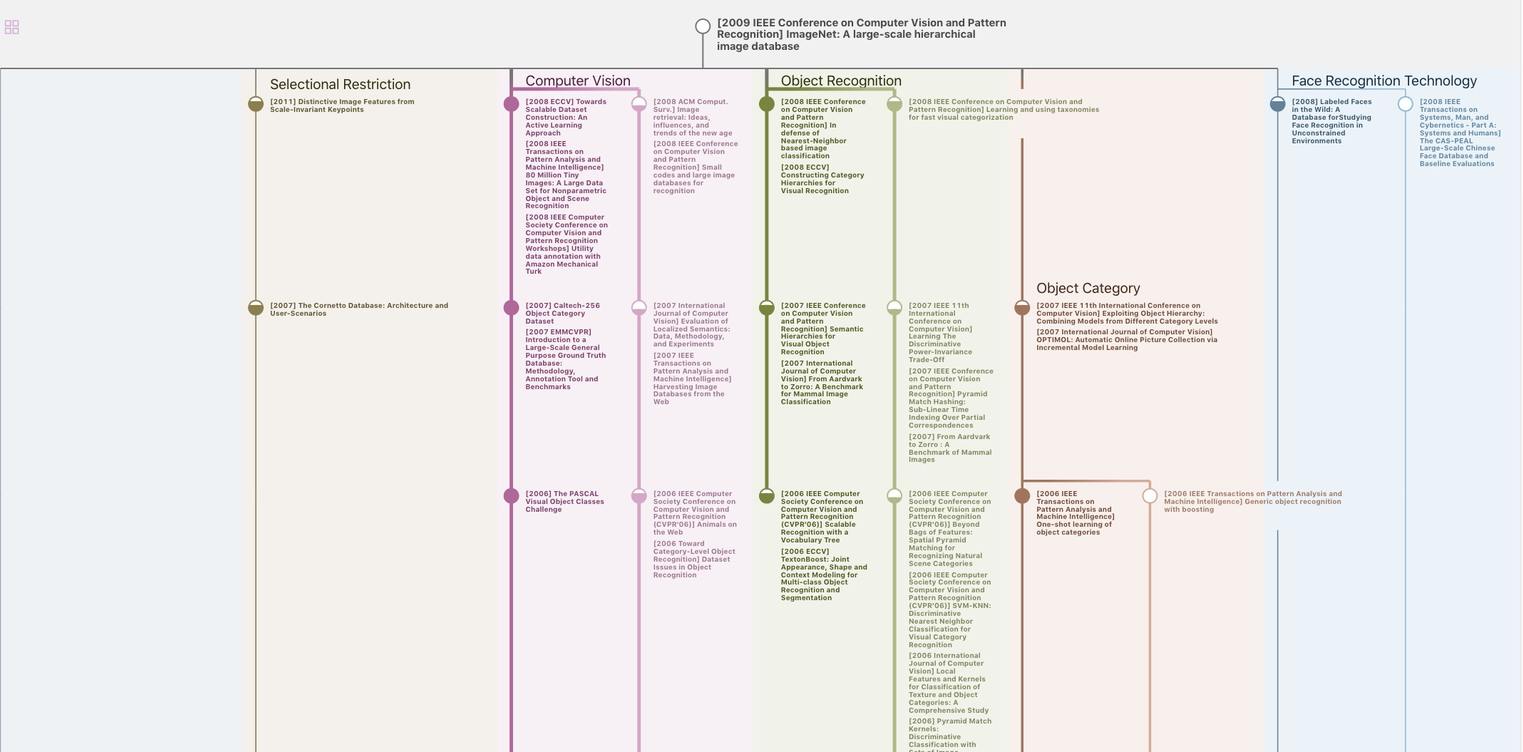
生成溯源树,研究论文发展脉络
Chat Paper
正在生成论文摘要