A review of mechanistic learning in mathematical oncology
arxiv(2023)
摘要
Mechanistic learning, the synergistic combination of knowledge-driven and data-driven modeling, is an emerging field. In particular, in mathematical oncology, the application of mathematical modeling to cancer biology and oncology, the use of mechanistic learning is growing. This review aims to capture the current state of the field and provide a perspective on how mechanistic learning may further progress in mathematical oncology. We highlight the synergistic potential of knowledge-driven mechanistic mathematical modeling and data-driven modeling, such as machine and deep learning. We point out similarities and differences regarding model complexity, data requirements, outputs generated, and interpretability of the algorithms and their results. Then, organizing combinations of knowledge- and data-driven modeling into four categories (sequential, parallel, intrinsic, and extrinsic mechanistic learning), we summarize a variety of approaches at the interface between purely data- and knowledge-driven models. Using examples predominantly from oncology, we discuss a range of techniques including physics-informed neural networks, surrogate model learning, and digital twins. We see that mechanistic learning, with its intentional leveraging of the strengths of both knowledge and data-driven modeling, can greatly impact the complex problems of oncology. Given the increasing ubiquity and impact of machine learning, it is critical to incorporate it into the study of mathematical oncology with mechanistic learning providing a path to that end. As the field of mechanistic learning advances, we aim for this review and proposed categorization framework to foster additional collaboration between the data- and knowledge-driven modeling fields. Further collaboration will help address difficult issues in oncology such as limited data availability, requirements of model transparency, and complex input data
更多查看译文
AI 理解论文
溯源树
样例
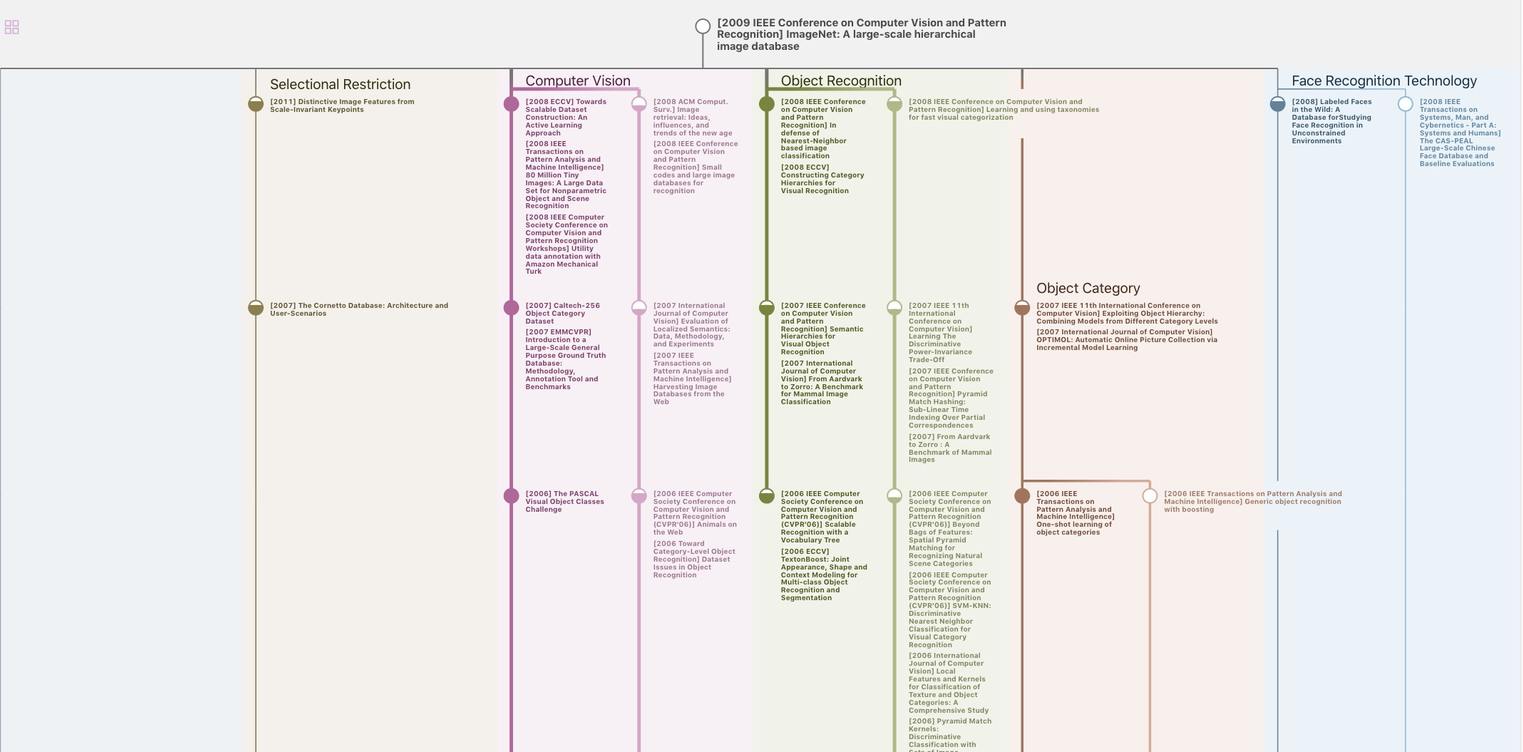
生成溯源树,研究论文发展脉络
Chat Paper
正在生成论文摘要