Using Machine Learning to Forecast Market Direction with Efficient Frontier Coefficients
The journal of financial data science(2023)
摘要
The authors propose a novel method to improve estimation of asset returns for portfolio optimization. This approach first performs a monthly directional market forecast using an online decision tree. The decision tree is trained on a novel set of features engineered from portfolio theory: the efficient frontier functional coefficients. Efficient frontiers can be decomposed to their functional form, a square-root second-order polynomial, and the coefficients of this function capture the information of all the constituents that compose the market in the current time period. To make these forecasts actionable, these directional forecasts are integrated to a portfolio optimization framework using expected returns conditional on the market forecast as an estimate for the return vector. This conditional expectation is calculated using the inverse Mills ratio, and the capital asset pricing model is used to translate the market forecast to individual asset forecasts. This novel method outperforms baseline portfolios, as well as other feature sets including technical indicators and the Fama–French factors. To empirically validate the proposed model, the authors employ a set of market sector exchange-traded funds.
更多查看译文
关键词
forecast market direction,machine learning
AI 理解论文
溯源树
样例
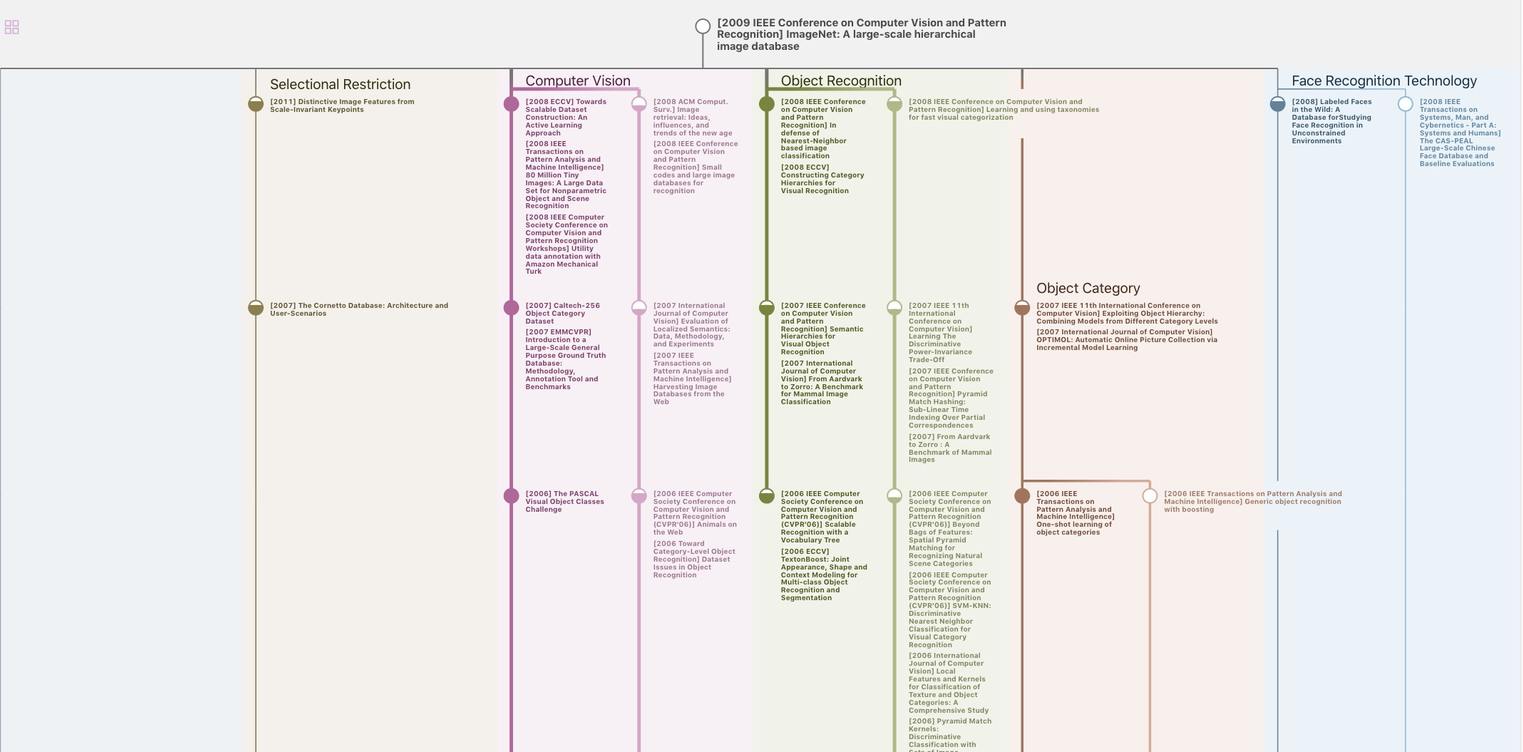
生成溯源树,研究论文发展脉络
Chat Paper
正在生成论文摘要