Search Optimization with Query Likelihood Boosting and Two-Level Approximate Search for Edge Devices
CoRR(2023)
摘要
We present a novel search optimization solution for approximate nearest
neighbor (ANN) search on resource-constrained edge devices. Traditional ANN
approaches fall short in meeting the specific demands of real-world scenarios,
e.g., skewed query likelihood distribution and search on large-scale indices
with a low latency and small footprint. To address these limitations, we
introduce two key components: a Query Likelihood Boosted Tree (QLBT) to
optimize average search latency for frequently used small datasets, and a
two-level approximate search algorithm to enable efficient retrieval with large
datasets on edge devices. We perform thorough evaluation on simulated and real
data and demonstrate QLBT can significantly reduce latency by 15% on real data
and our two-level search algorithm successfully achieve deployable accuracy and
latency on a 10 million dataset for edge devices. In addition, we provide a
comprehensive protocol for configuring and optimizing on-device search
algorithm through extensive empirical studies.
更多查看译文
AI 理解论文
溯源树
样例
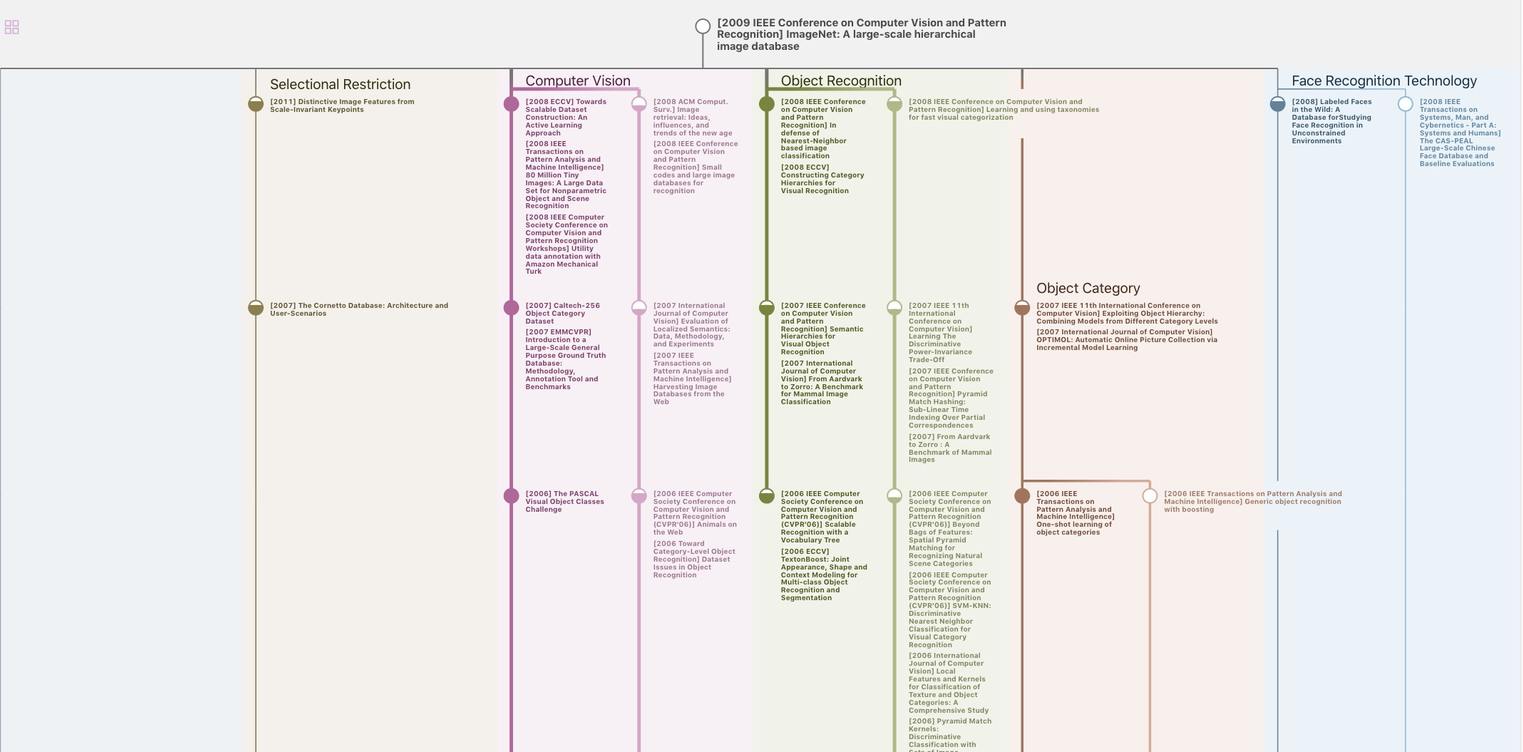
生成溯源树,研究论文发展脉络
Chat Paper
正在生成论文摘要