Statistically Distinct Plans for Multi-Objective Task Assignment
CoRR(2023)
摘要
We study the problem of finding statistically distinct plans for stochastic
planning and task assignment problems such as online multi-robot pickup and
delivery (MRPD) when facing multiple competing objectives. In many real-world
settings robot fleets do not only need to fulfil delivery requests, but also
have to consider auxiliary objectives such as energy efficiency or avoiding
human-centered work spaces. We pose MRPD as a multi-objective optimization
problem where the goal is to find MRPD policies that yield different trade-offs
between given objectives. There are two main challenges: 1) MRPD is
computationally hard, which limits the number of trade-offs that can reasonably
be computed, and 2) due to the random task arrivals, one needs to consider
statistical variance of the objective values in addition to the average. We
present an adaptive sampling algorithm that finds a set of policies which i)
are approximately optimal, ii) approximate the set of all optimal solutions,
and iii) are statistically distinguishable. We prove completeness and adapt a
state-of-the-art MRPD solver to the multi-objective setting for three example
objectives. In a series of simulation experiments we demonstrate the advantages
of the proposed method compared to baseline approaches and show its robustness
in a sensitivity analysis. The approach is general and could be adapted to
other multi-objective task assignment and planning problems under uncertainty.
更多查看译文
关键词
Multi-Robot Task Assignment,Pickup and Delivery,Path Planning for Multiple Mobile Robots,Multi-Objective Optimization
AI 理解论文
溯源树
样例
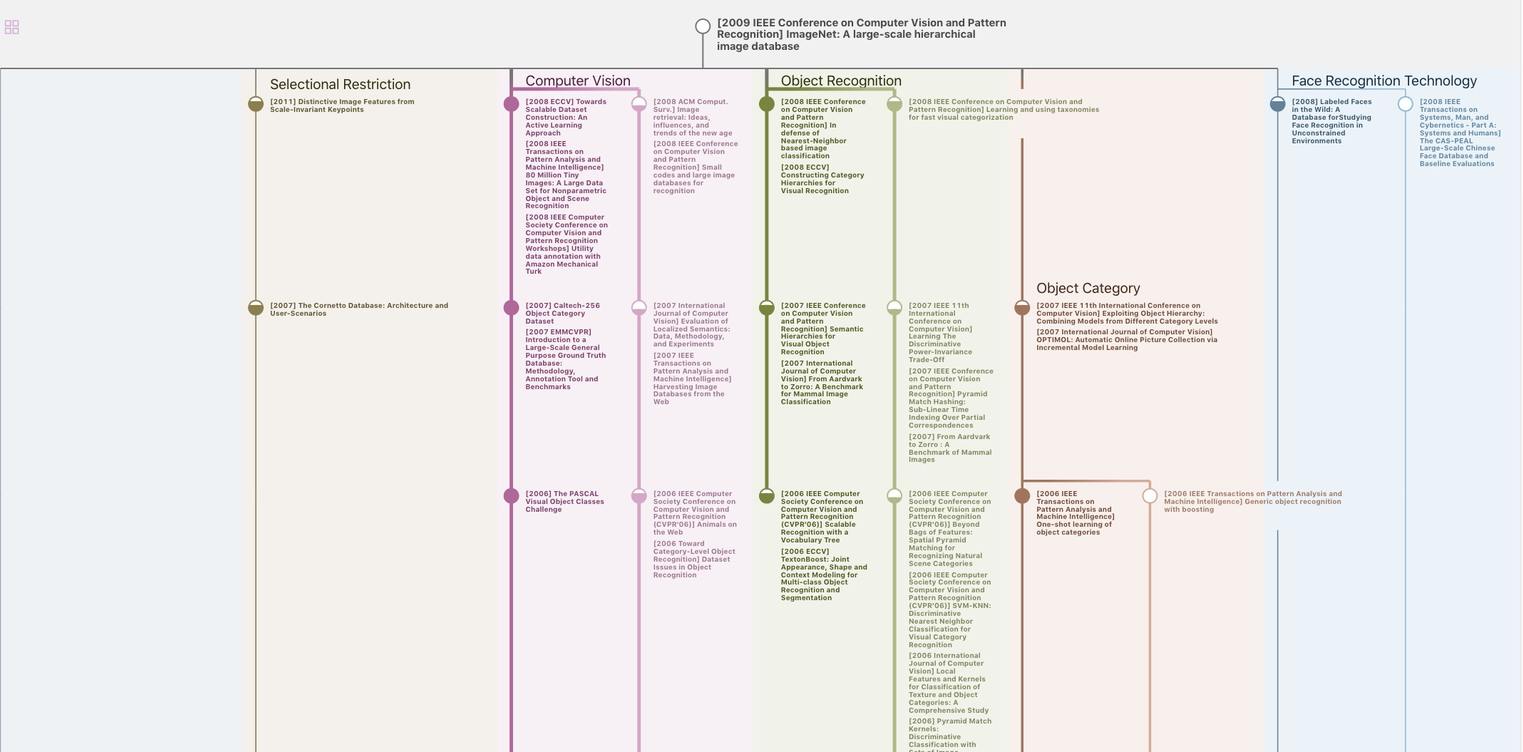
生成溯源树,研究论文发展脉络
Chat Paper
正在生成论文摘要