Utilizing machine learning and lipidomics to distinguish primary lateral sclerosis from amyotrophic lateral sclerosis
Muscle & Nerve(2023)
摘要
There are currently no imaging or blood diagnostic biomarkers that can differentiate amyotrophic lateral sclerosis (ALS) from primary lateral sclerosis (PLS) patients early in their disease courses. Our objective is to examine whether patients with PLS can be differentiated from ALS reliably by using plasma lipidome profile and supervised machine learning.40 ALS and 28 PLS patients derived from the Multicenter Cohort study of Oxidative Stress (COSMOS) and 28 healthy control volunteers (CTR) were included. ALS, PLS, and CTR were matched by age and sex. Plasma samples were obtained after overnight fasting. Lipids were extracted from the plasma samples and analyzed using liquid chromatography/mass spectrometry to obtain relative concentrations of 392 lipid species. The lipid data were partitioned into training and testing datasets randomly. An elastic net algorithm was trained using cross-validation to classify PLS vs ALS and PLS vs CTR. Final accuracy was evaluated in the testing dataset.The elastic net model trained with labeled PLS and ALS training lipid dataset demonstrated accuracy (number classified correctly/total number), sensitivity, and specificity of 100% in classifying PLS vs ALS in the unlabeled testing lipid dataset. Similarly, the elastic net model trained with labeled PLS and CTR training lipid datasets demonstrated accuracy, sensitivity, and specificity of 88% in classifying PLS vs CTR in the unlabeled testing lipid dataset.Our study suggests PLS patients can be accurately distinguished from ALS and CTR by combining lipidome profile and supervised machine learning without clinical information.
更多查看译文
关键词
primary lateral sclerosis,machine learning,lipidomics
AI 理解论文
溯源树
样例
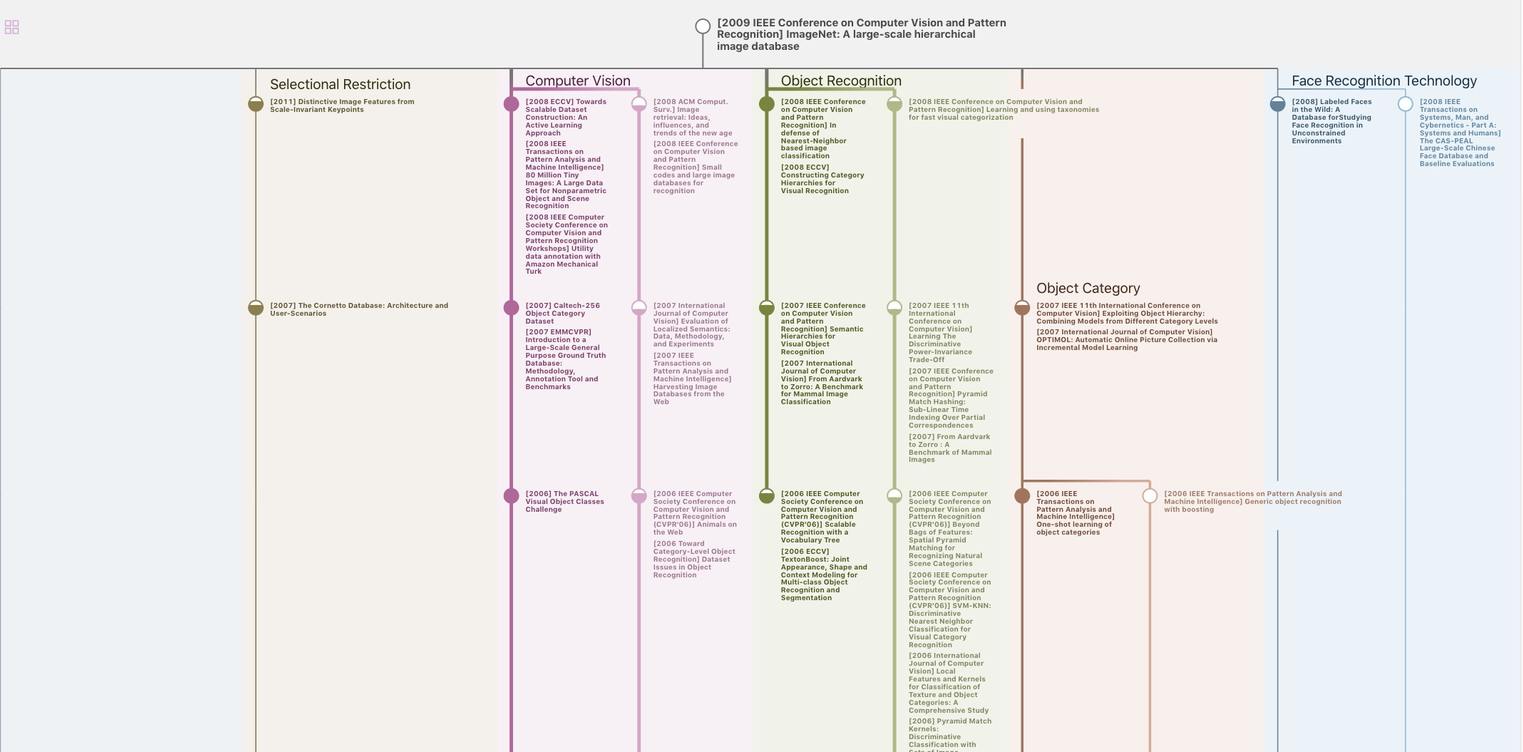
生成溯源树,研究论文发展脉络
Chat Paper
正在生成论文摘要