Spatial-Contextual Discrepancy Information Compensation for GAN Inversion
AAAI 2024(2024)
摘要
Most existing GAN inversion methods either achieve accurate reconstruction but lack editability or offer strong editability at the cost of fidelity. Hence, how to balance the distortion-editability trade-off is a significant challenge for GAN inversion. To address this challenge, we introduce a novel spatial-contextual discrepancy information compensation-based GAN-inversion method (SDIC), which consists of a discrepancy information prediction network (DIPN) and a discrepancy information compensation network (DICN). SDIC follows a ``compensate-and-edit'' paradigm and successfully bridges the gap in image details between the original image and the reconstructed/edited image. On the one hand, DIPN encodes the multi-level spatial-contextual information of the original and initial reconstructed images and then predicts a spatial-contextual guided discrepancy map with two hourglass modules. In this way, a reliable discrepancy map that models the contextual relationship and captures fine-grained image details is learned. On the other hand, DICN incorporates the predicted discrepancy information into both the latent code and the GAN generator with different transformations, generating high-quality reconstructed/edited images. This effectively compensates for the loss of image details during GAN inversion. Both quantitative and qualitative experiments demonstrate that our proposed method achieves the excellent distortion-editability trade-off at a fast inference speed for both image inversion and editing tasks. Our code is available at https://github.com/ZzqLKED/SDIC.
更多查看译文
关键词
CV: Computational Photography, Image & Video Synthesis
AI 理解论文
溯源树
样例
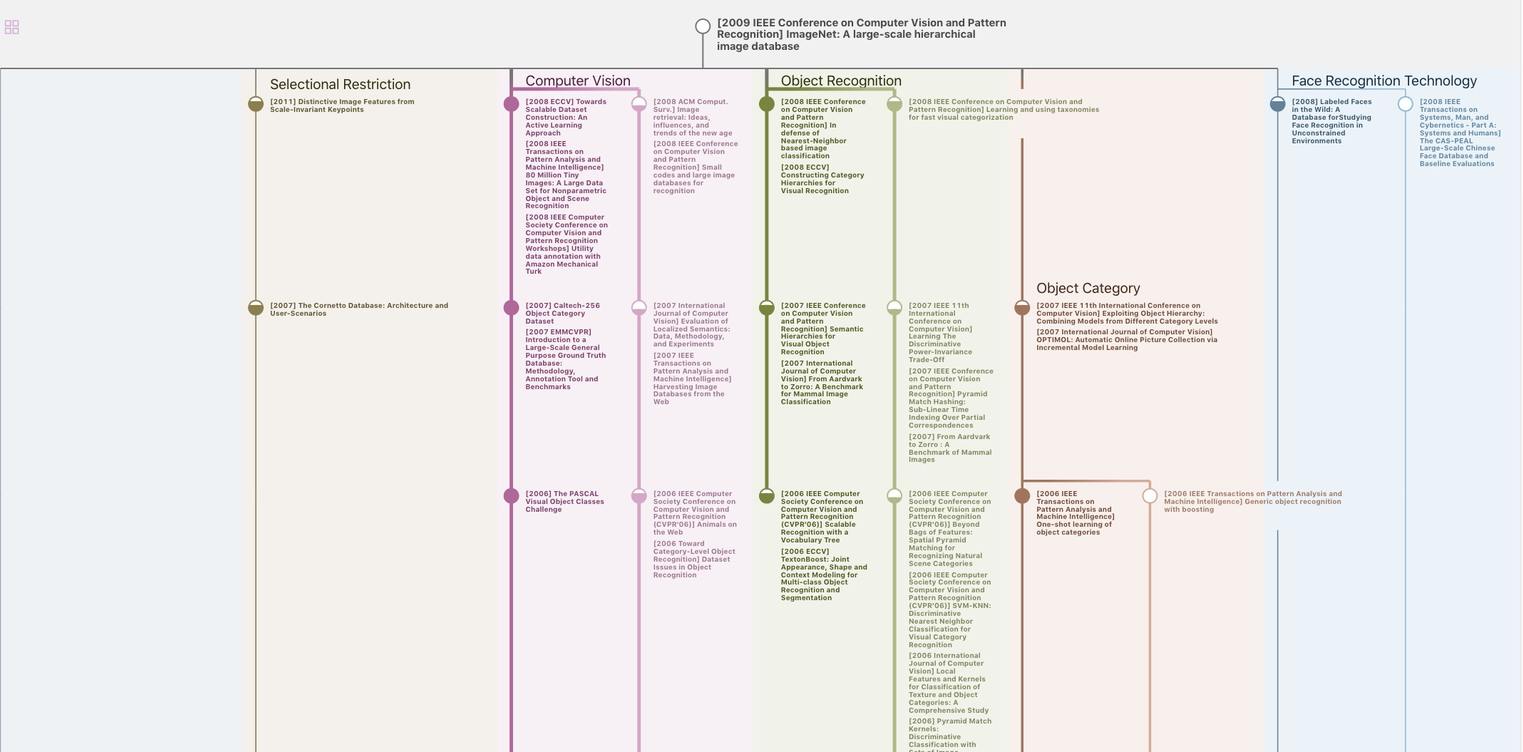
生成溯源树,研究论文发展脉络
Chat Paper
正在生成论文摘要