Deep Learning Based Modeling of Wireless Communication Channel with Fading
International Conference on Wireless Communications and Mobile Computing(2024)
关键词
Deep Learning,Neural Networks,Wireless Channel,Stochastic Model
AI 理解论文
溯源树
样例
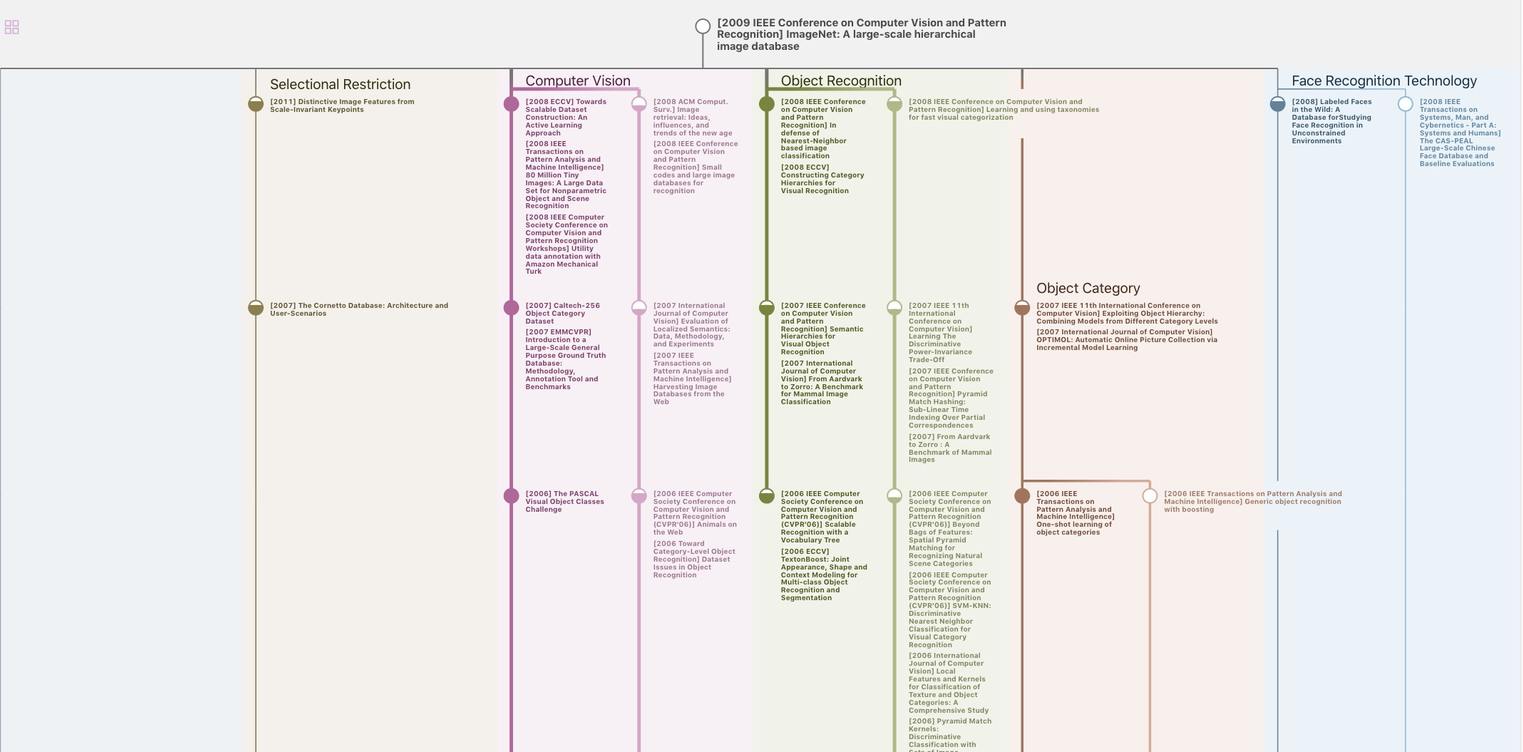
生成溯源树,研究论文发展脉络
Chat Paper
正在生成论文摘要