Spectral State Space Models
CoRR(2023)
摘要
This paper studies sequence modeling for prediction tasks with long range
dependencies. We propose a new formulation for state space models based on
learning linear dynamical systems with the spectral filtering algorithm
[HSZ17]. This gives rise to a novel sequence prediction architecture we call
spectral state space models. The resulting models are evaluated on synthetic
dynamical systems. These evaluations support the theoretical benefits of
spectral filtering for tasks requiring very long range memory.
更多查看译文
AI 理解论文
溯源树
样例
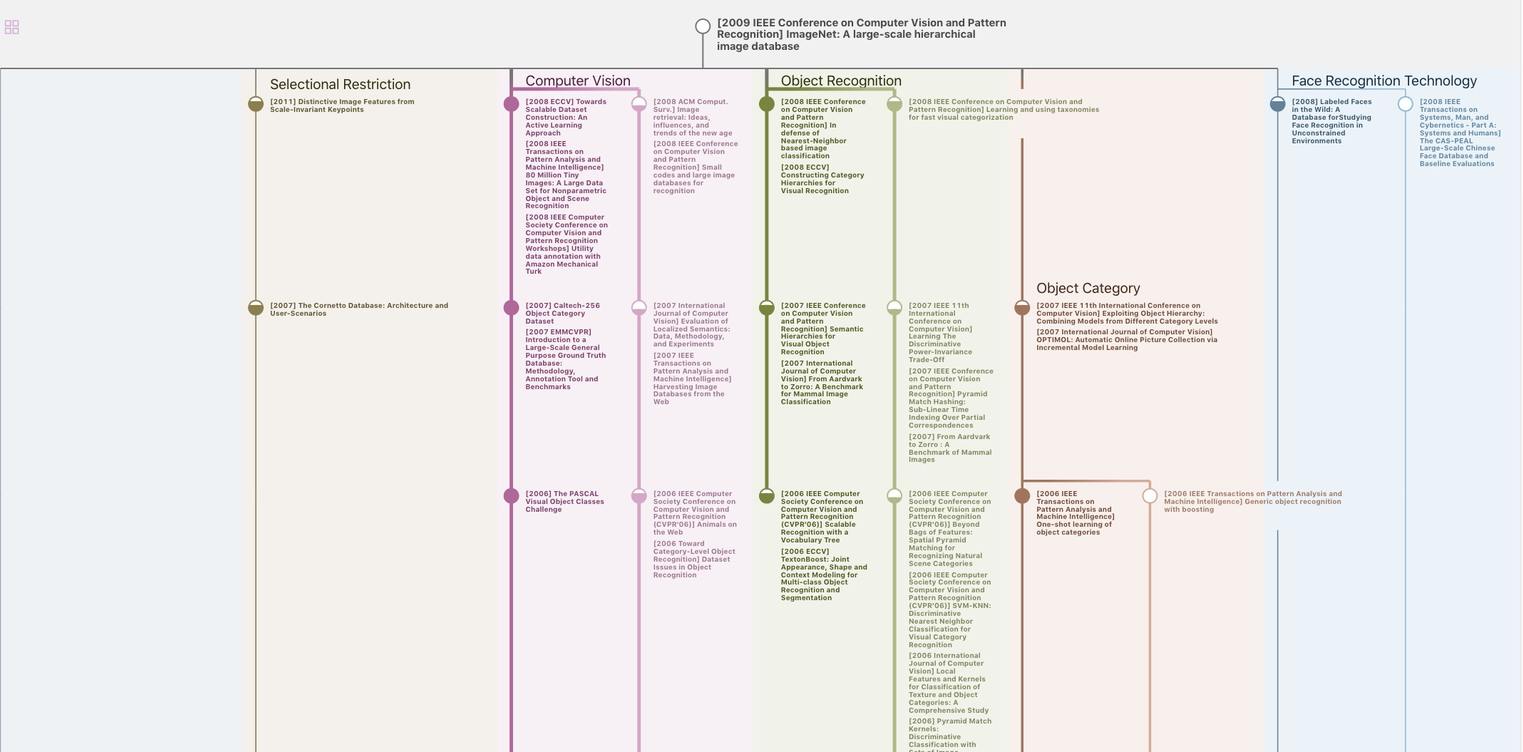
生成溯源树,研究论文发展脉络
Chat Paper
正在生成论文摘要