EgoPlan-Bench: Benchmarking Egocentric Embodied Planning with Multimodal Large Language Models
arxiv(2023)
摘要
Multimodal Large Language Models, combining the remarkable reasoning and
generalization capabilities of Large Language Models (LLMs) with the ability to
comprehend visual inputs, have opened up new avenues for embodied task
planning. Given diverse environmental inputs, including real-time task
progress, visual observations, and open-form language instructions, a
proficient task planner is expected to predict feasible actions, which is a
feat inherently achievable by Multimodal Large Language Models (MLLMs). In this
paper, we aim to quantitatively investigate the potential of MLLMs as embodied
task planners in real-world scenarios by introducing a benchmark with human
annotations named EgoPlan-Bench. Our benchmark is distinguished by realistic
tasks derived from real-world videos, a diverse set of actions involving
interactions with hundreds of different objects, and complex visual
observations from varied scenes. We evaluate a wide range of MLLMs, revealing
that these models have not yet evolved into embodied planning generalists (even
GPT-4V). We further construct an instruction-tuning dataset EgoPlan-IT from
videos with human-object interactions, to facilitate the learning of high-level
task planning in intricate real-world situations. The experiment results
demonstrate that the model tuned on EgoPlan-IT not only significantly improves
performance on our benchmark, but can also be applied as a task planner for
guiding embodied agents in simulations.
更多查看译文
AI 理解论文
溯源树
样例
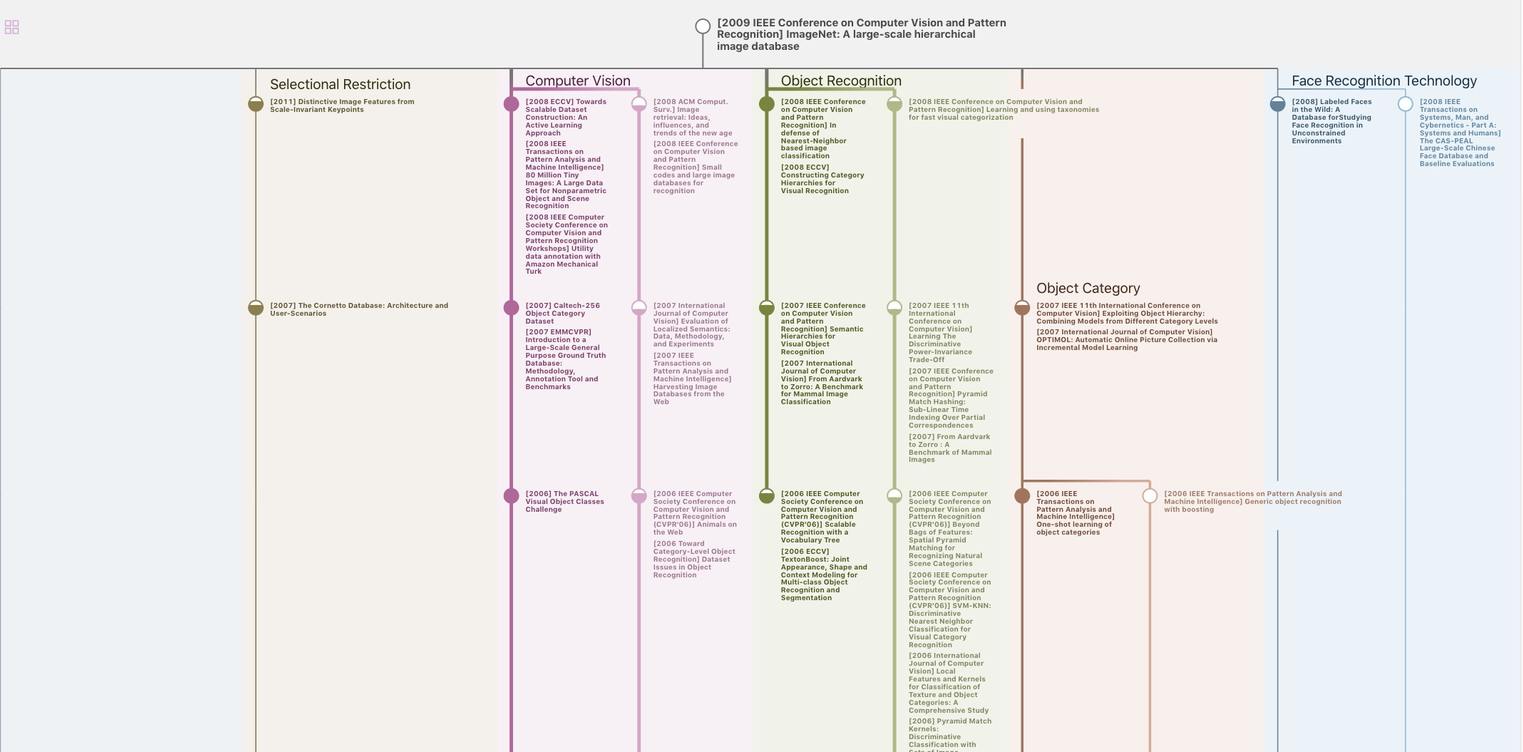
生成溯源树,研究论文发展脉络
Chat Paper
正在生成论文摘要