Identifying the determining factors of detonation properties for linear nitroaliphatics with high-throughput computation and machine learning
Energetic Materials Frontiers(2023)
摘要
In this work, a high-throughput computation (HTC) and machine learning (ML) combined method was applied to identify the determining factors of the detonation velocity (vd) and detonation pressure (pd) of energetic molecules and screen potential high-energy molecules with acceptable stability in a high-throughput way. The HTC was performed based on 1725 sample molecules abstracted from a dataset of over 106 linear nitroaliphatics with 1- to 6-membered C backbones and three types of substituents, namely single nitro group (-NO2), nitroamine (-NNO2), and nitrate ester (-ONO2). ML models were established based on the HTC results to screen high-energy molecules and to identify the determining factors of vd and pd. Compared with quantum chemistry calculation results, the absolute relative errors of vd and pd obtained using the ML models were less than 3.63% and 5%, respectively. Furthermore, eight molecules with high energy and acceptable stability were selected as potential candidates. This study shows the high efficiency of the combination of HTC and ML in high-throughput screening.
更多查看译文
关键词
Energetic molecule,Molecular design,High-throughput computation,Machine learning,Detonation property
AI 理解论文
溯源树
样例
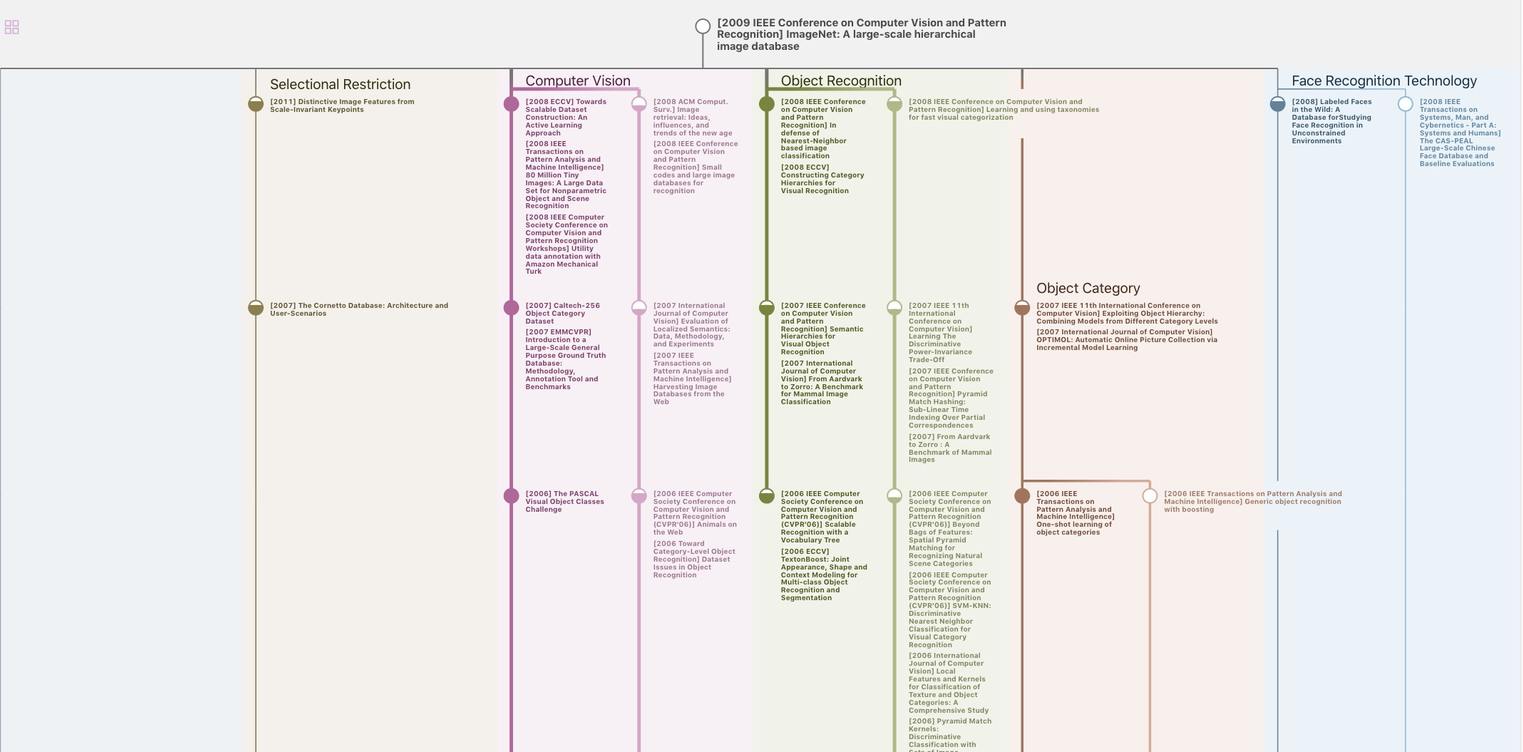
生成溯源树,研究论文发展脉络
Chat Paper
正在生成论文摘要