Adaptive Static Equivalences for Active Distribution Networks With Massive Renewable Energy Integration: A Distributed Deep Reinforcement Learning Approach
IEEE Transactions on Network Science and Engineering(2023)
摘要
Active distribution networks (ADNs) with 100% renewable energy sources (RESs) penetration are essential in the net-zeros emission power sector. As an efficient analysis tool, the equivalent model for ADNs (EMADNs) can be leveraged to expedite the lengthy analysis and preserve data privacy. Nevertheless, volatile RESs, along with their active management techniques, e.g., voltage regulation schemes (VRSs), deteriorate the fidelity of the developed EMADNs derived from historical data. This paper proposes an adaptive EMADN with tunable network scales and adaptive parameters to address the above challenges. A leaves-trimming network topological reduction algorithm is utilized to preserve the radial topology, the nodes of interest, and VRSs. Upon the derived network topology and components, a distributed Proximal Policy Optimization (DPPO)-based deep reinforcement learning agent is employed to adjust the parameters periodically to maintain the fidelity of EMADNs over time. The distributed training scheme extends the ordinary PPO to boost exploration and training efficiency by exploiting the multi-processors in a synchronous way. Case studies on the IEEE-33 bus and IEEE 123-bus ADNs with massive photovoltaic and wind generation demonstrate the superior accuracy and efficiency of the proposed agent-based EMADNs in various scenarios, especially when the production of RESs exceeds the load amount.
更多查看译文
关键词
active distribution networks,deep reinforcement learning,distributed learning,network reduction,voltage regulation schemes
AI 理解论文
溯源树
样例
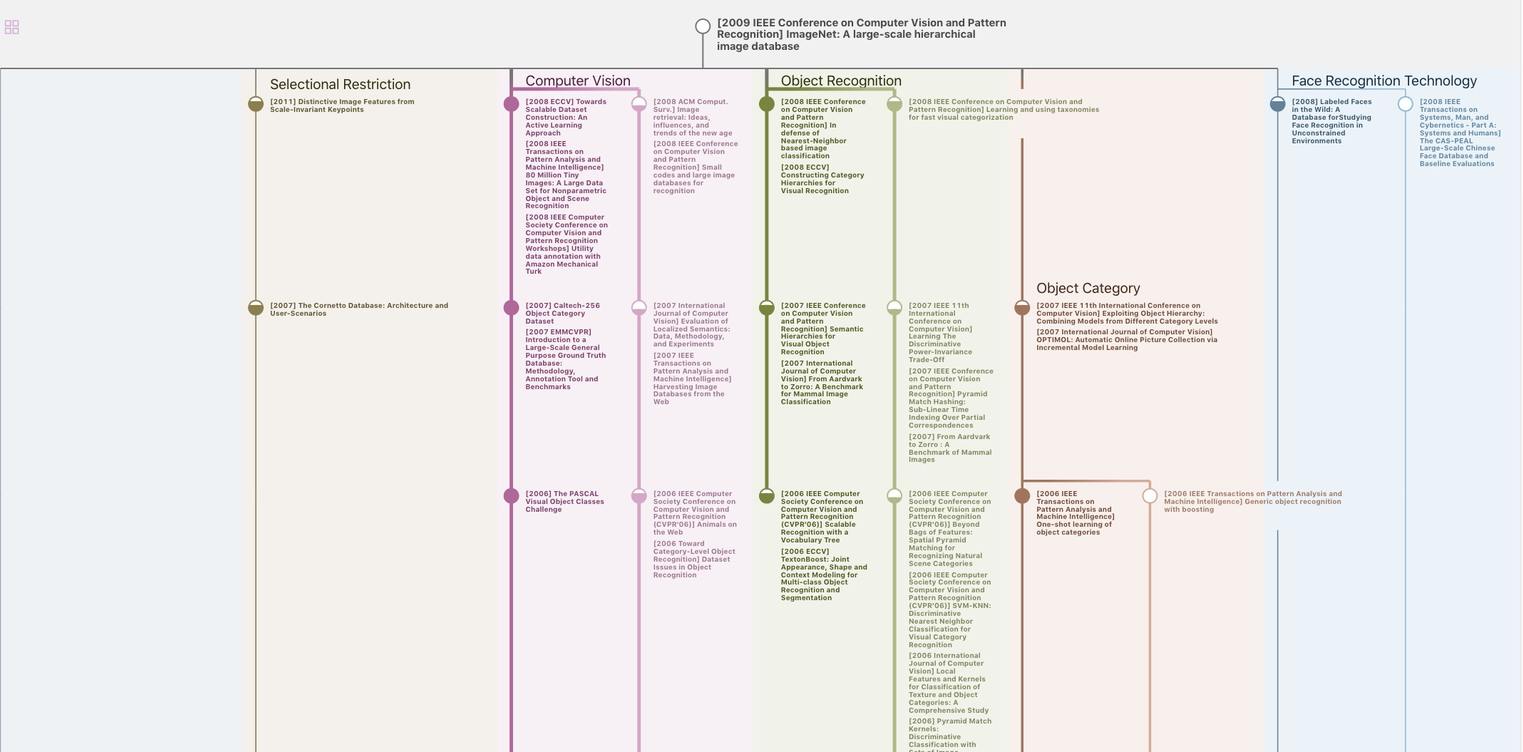
生成溯源树,研究论文发展脉络
Chat Paper
正在生成论文摘要