Quality Regularization based Semisupervised Adversarial Transfer Model with Unlabeled Data for Industrial Soft Sensing
IEEE TRANSACTIONS ON INDUSTRIAL INFORMATICS(2024)
Abstract
Traditional soft sensors typically rely only on labeled data to predict key variables, despite the significant amount of unlabeled data that could provide valuable information. To solve this problem, a quality regularization based semisupervised adversarial transfer model (QR-SATM) is proposed. The idea of transfer learning is used in QR-SATM. QR-SATM comprises a pre-training model and a regression model. The pre-training model is an unsupervised model. And the regression model is a supervised model with a similar structure to the pre-training model, allowing for easy transfer between the two models. Firstly, the pre-training model is trained with unlabeled data to extract features. Then the trained parameters of pre-training model are transferred to the regression model, and the regression model is fine-tuned with labeled data. During fine-tuning the regression model, an improved quality regularization is introduced in order to select useful features and prevent overfitting. QR-SATM is validated by a real industrial dataset of purified terephthalic acid (PTA). The experimental results show the effectiveness of the proposed QR-SATM in accurately predicting key variables.
MoreTranslated text
Key words
Soft sensors,Transfer learning,Adversarial auto-encoder,Industrial processes.
AI Read Science
Must-Reading Tree
Example
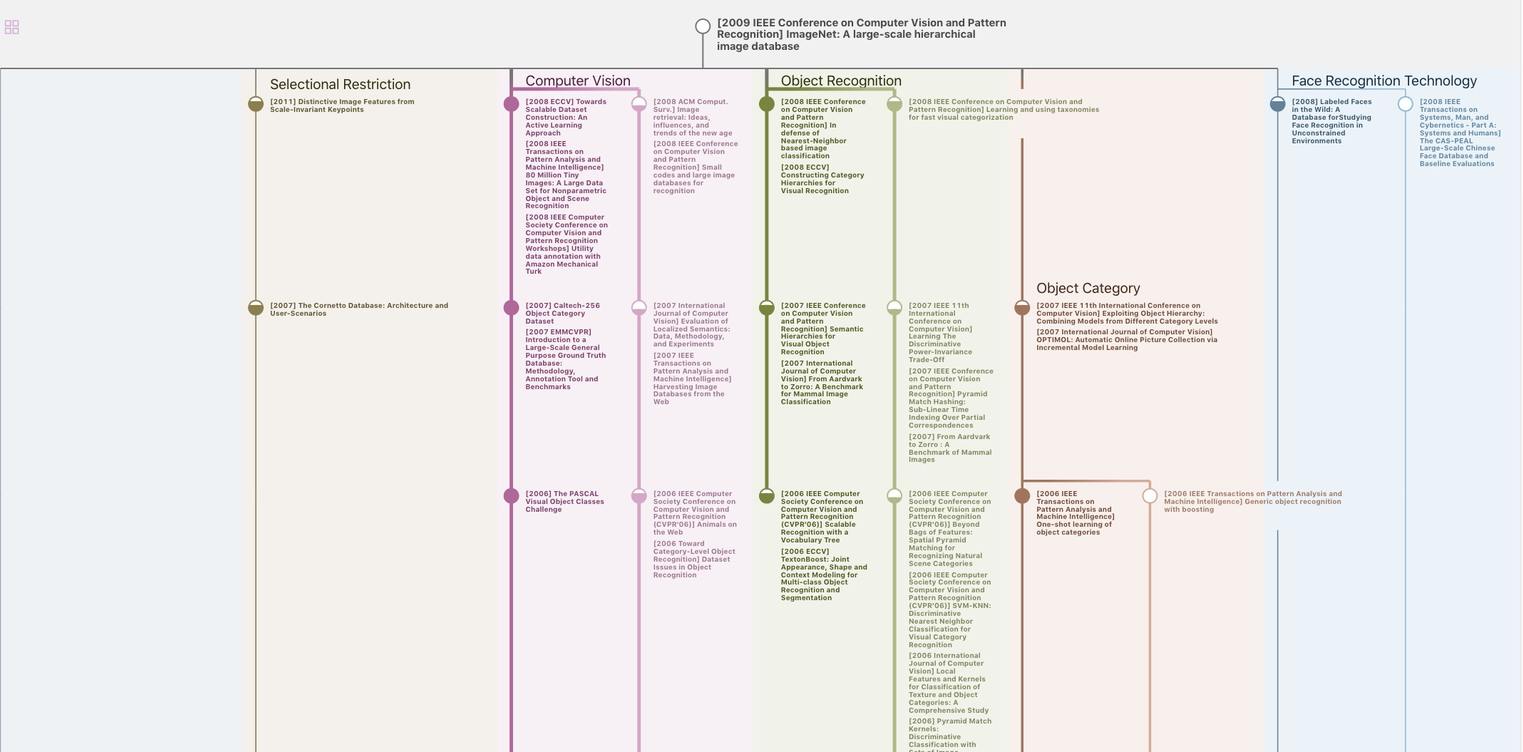
Generate MRT to find the research sequence of this paper
Chat Paper
Summary is being generated by the instructions you defined