Deep learning‐based classification and spatial prognosis risk score on whole‐slide images of lung adenocarcinoma
Histopathology(2023)
摘要
Classification of histological patterns in lung adenocarcinoma (LUAD) is critical for clinical decision-making, especially in the early stage. However, the inter- and intraobserver subjectivity of pathologists make the quantification of histological patterns varied and inconsistent. Moreover, the spatial information of histological patterns is not evident to the naked eye of pathologists.We establish the LUAD-subtype deep learning model (LSDLM) with optimal ResNet34 followed by a four-layer Neural Network classifier, based on 40 000 well-annotated path-level tiles. The LSDLM shows robust performance for the identification of histopathological subtypes on the whole-slide level, with an area under the curve (AUC) value of 0.93, 0.96 and 0.85 across one internal and two external validation data sets. The LSDLM is capable of accurately distinguishing different LUAD subtypes through confusion matrices, albeit with a bias for high-risk subtypes. It possesses mixed histology pattern recognition on a par with senior pathologists. Combining the LSDLM-based risk score with the spatial K score (K-RS) shows great capacity for stratifying patients. Furthermore, we found the corresponding gene-level signature (AI-SRSS) to be an independent risk factor correlated with prognosis.Leveraging state-of-the-art deep learning models, the LSDLM shows capacity to assist pathologists in classifying histological patterns and prognosis stratification of LUAD patients.
更多查看译文
关键词
lung adenocarcinoma,spatial prognosis risk score,deep learning‐based
AI 理解论文
溯源树
样例
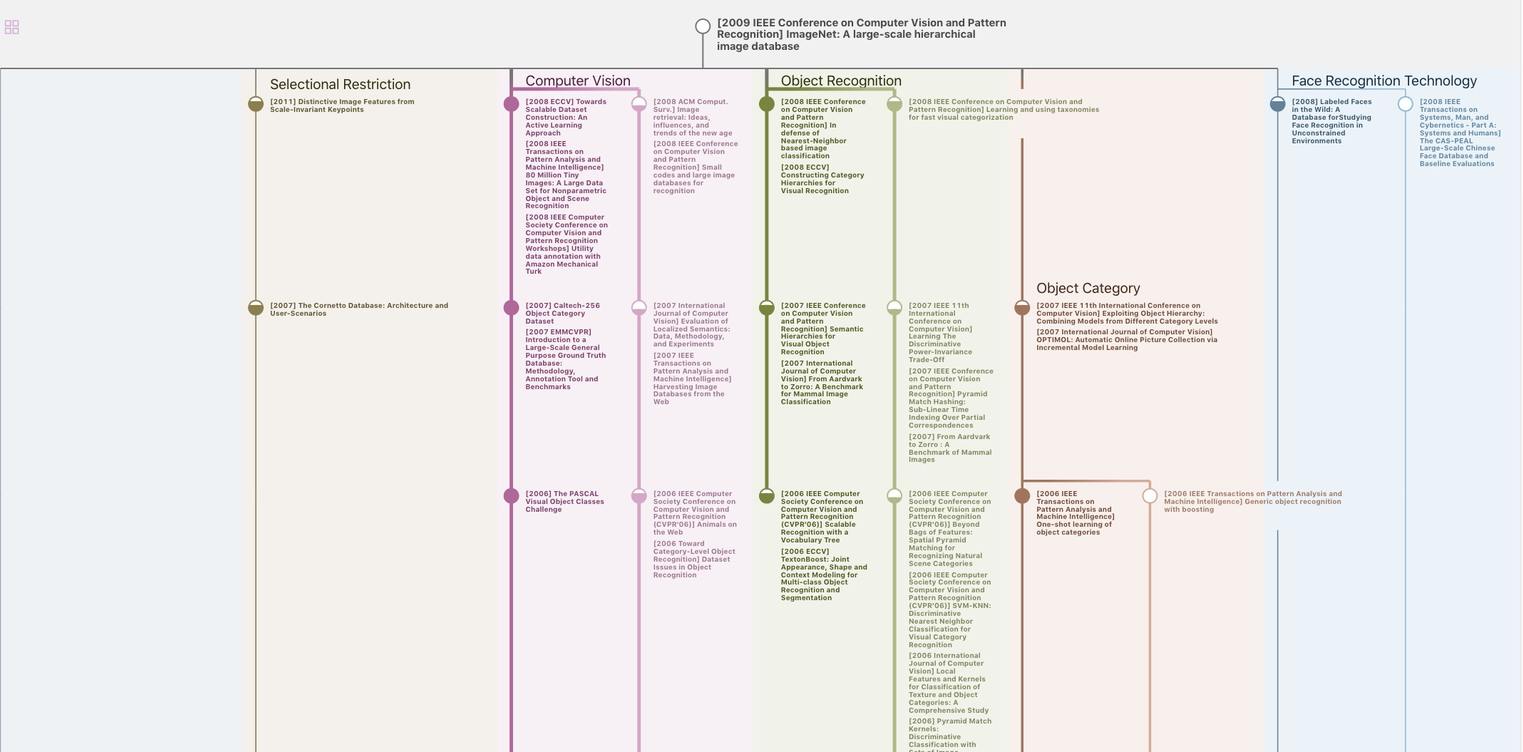
生成溯源树,研究论文发展脉络
Chat Paper
正在生成论文摘要