Stable Knowledge Tracing Using Causal Inference
IEEE TRANSACTIONS ON LEARNING TECHNOLOGIES(2024)
摘要
Knowledge tracing (KT) for evaluating students' knowledge is an essential task in personalized education. More and more researchers have devoted themselves to solving KT tasks, e.g., deep knowledge tracing (DKT), which can capture more sophisticated representations of student knowledge. Nonetheless, these techniques ignore the reconstruction of the observed input information. Therefore, this leads to poor predictions of students' knowledge, even if the student performed well in the past knowledge state. In this article, we first employ causal inference for explanatory analysis of KT, then propose a learning algorithm for stable KT based on the analysis outcomes. The proposed approach aims to achieve stable KT by constructing global balanced weights that facilitate estimating feature influence and assessing causal relationships between individual variables and outcome variables. We have proved the approach has effective in accuracy and interpretability through extensive experimentation on real-world datasets. In conclusion, this article has methodological implications for the stable assessment of students' knowledge and provides a reference for personalization and use of intelligence in the educational teaching process.
更多查看译文
关键词
Analytical models,Education,Predictive models,Prediction algorithms,Inference algorithms,Stability analysis,Robustness,Causal inference,deep knowledge tracing (DKT),learning sciences,stable knowledge tracing (KT)
AI 理解论文
溯源树
样例
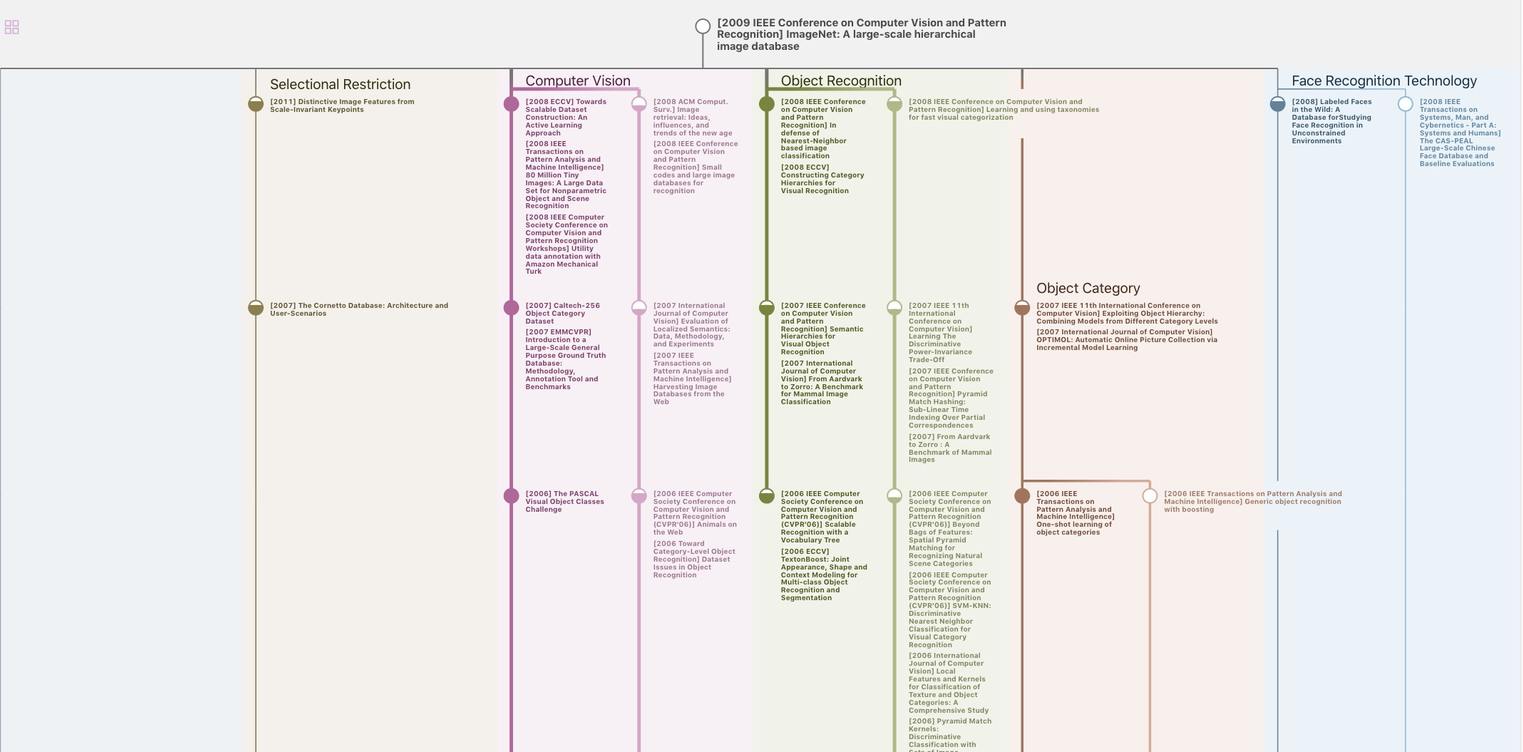
生成溯源树,研究论文发展脉络
Chat Paper
正在生成论文摘要