Abstract 1120: Dissecting tumor cell programs through group biology estimation in clinical single-cell transcriptomics
Cancer Research(2023)
Abstract
Abstract Translational oncology studies increasingly emphasize deriving hypotheses from single-cell datasets by evaluating groups of patients (e.g., treated vs. untreated, responders vs. non-responders, early vs. late-stage tumors) for differences in gene signatures/pathways to guide subsequent investigations. However, when evaluating these questions using tumor single-cell data, current statistical methods for this task are limited in two major aspects: (i) They are not representative of the hierarchical nature of tumor single-cell datasets (where cells are more similar within the patient than between patients); and (ii) They often do not contextualize statistical significance (p-values) in the context of variability that is expected biologically between patients. This can lead to molecular hypotheses that are skewed by patient-specific biology with high false positive rates. To address these challenges, we developed a nonparametric statistical method, BEANIE (group Biology EstimAtioN in sIngle cEll), for estimating group biology in single-cell transcriptomic datasets. BEANIE uses monte carlo simulations to estimate the p-value distribution for the gene signature of interest and normalizes with respect to a background distribution of the expected patient-to-patient variability. The method then performs leave-one-out cross-validation to infer robustness of the gene signatures to patient exclusion. We applied our method to public cancer single-cell datasets to evaluate candidate differences in tumor cell populations between known groups. When comparing tumor cell programs derived from treatment-naive vs. chemotherapy-treated Triple-Negative Breast Cancer (TNBC) patients, BEANIE identified upregulation of hallmark gene signatures for fatty acid metabolism and hypoxia to be statistically significant and robust in the treatment-naive group, indicative of response to chemotherapy treatment. We also compared BEANIE to conventional methods like Mann-Whitney U (MWU) test followed by Benjamini-Hochberg (BH) correction and Generalized Linear Models (GLM). MWU test and GLM also identified these signatures to be upregulated, but they additionally identified other immune cell gene signatures like T-cell markers, B-cell markers and NK cell markers, to also be upregulated, which was not expected since we were comparing between tumor cells. Similarly, BEANIE identified candidate tumor cell intrinsic programs relevant to hypotheses in lung cancer patients (early vs. late-stage) and melanoma patients (Immune Checkpoint Blockade (ICB)-naive vs. ICB-treated). Therefore, BEANIE greatly increased the specificity of analysis, and reduced false positive rates. This method is publicly available and may overcome existing methodological limitations to guide tumor-intrinsic hypothesis generation in rapidly growing clinical cancer single-cell cohorts. Citation Format: Shreya Johri, Kevin Bi, Breanna M. Titchen, Jingxin Fu, Jake Conway, Jett Crowdis, Natalie I. Volkes, Zenghua Fan, Lawrence Fong, Jihye Park, David Liu, Meng Xiao He, Eliezer M. Van Allen. Dissecting tumor cell programs through group biology estimation in clinical single-cell transcriptomics [abstract]. In: Proceedings of the American Association for Cancer Research Annual Meeting 2023; Part 1 (Regular and Invited Abstracts); 2023 Apr 14-19; Orlando, FL. Philadelphia (PA): AACR; Cancer Res 2023;83(7_Suppl):Abstract nr 1120.
MoreTranslated text
Key words
tumor cell programs,group biology estimation,transcriptomics,single-cell cell,tumor cell
AI Read Science
Must-Reading Tree
Example
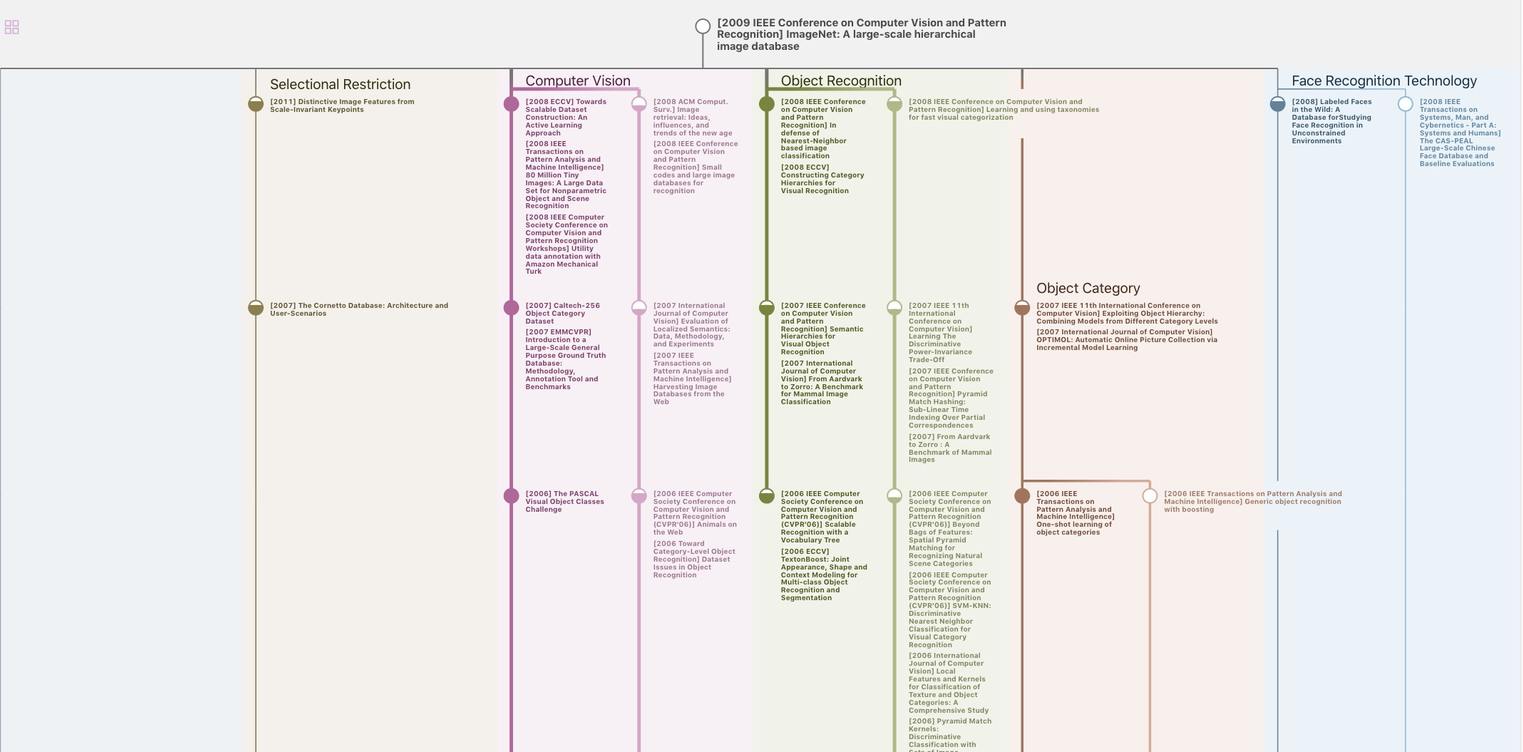
Generate MRT to find the research sequence of this paper
Chat Paper
Summary is being generated by the instructions you defined