Quantum Circuit Fidelity Improvement with Long Short-Term Memory Networks
arXiv (Cornell University)(2023)
摘要
Although NISQ computers show great promise in accelerating many tasks that are not practically possible using classical computation, useful quantum computing is still a long way off. One important reason is due to the fragile nature of quantum hardware. As the building blocks of a quantum circuit (QC), quantum gates and qubits are susceptible to external interference, and therefore even a simple QC can produce extremely noisy output. Since it is hard to distinguish whether the output represents meaningful computation or just random noise, it raises the question of how much we can rely on the output of a QC, i.e., the fidelity of the QC. In this paper, we purpose a simple yet intuitive metric to measure the fidelity of a QC. By using this metric, we can observe the evolution of fidelity with time as the QC interacts with its external environment. Consequently, we can frame fidelity prediction as a Time Series Forecasting problem and use Long Short-Term Memory (LSTM) neural networks to better estimate the fidelity of a QC. This gives the user better opportunities to optimize the mapping of qubits into the quantum hardware for larger gains. We introduce the LSTM architecture and present a complete workflow to build the training circuit dataset. The trained LSTM system, Q-fid, can predict the output fidelity of a QC running on a specific quantum processor, without the need for any separate input of hardware calibration data or gate error rates. Evaluated on the QASMbench NISQ benchmark suite, Q-fid's prediction achieves an average RMSE of 0.0515, up to 24.7x more accurate than the default Qiskit transpile tool mapomatic. When used to find the high-fidelity circuit layouts from the available circuit transpilations, Q-fid predicts the fidelity for the top 10% layouts with an average RMSE of 0.0252, up to 32.8x more accurate than mapomatic.
更多查看译文
关键词
quantum,memory
AI 理解论文
溯源树
样例
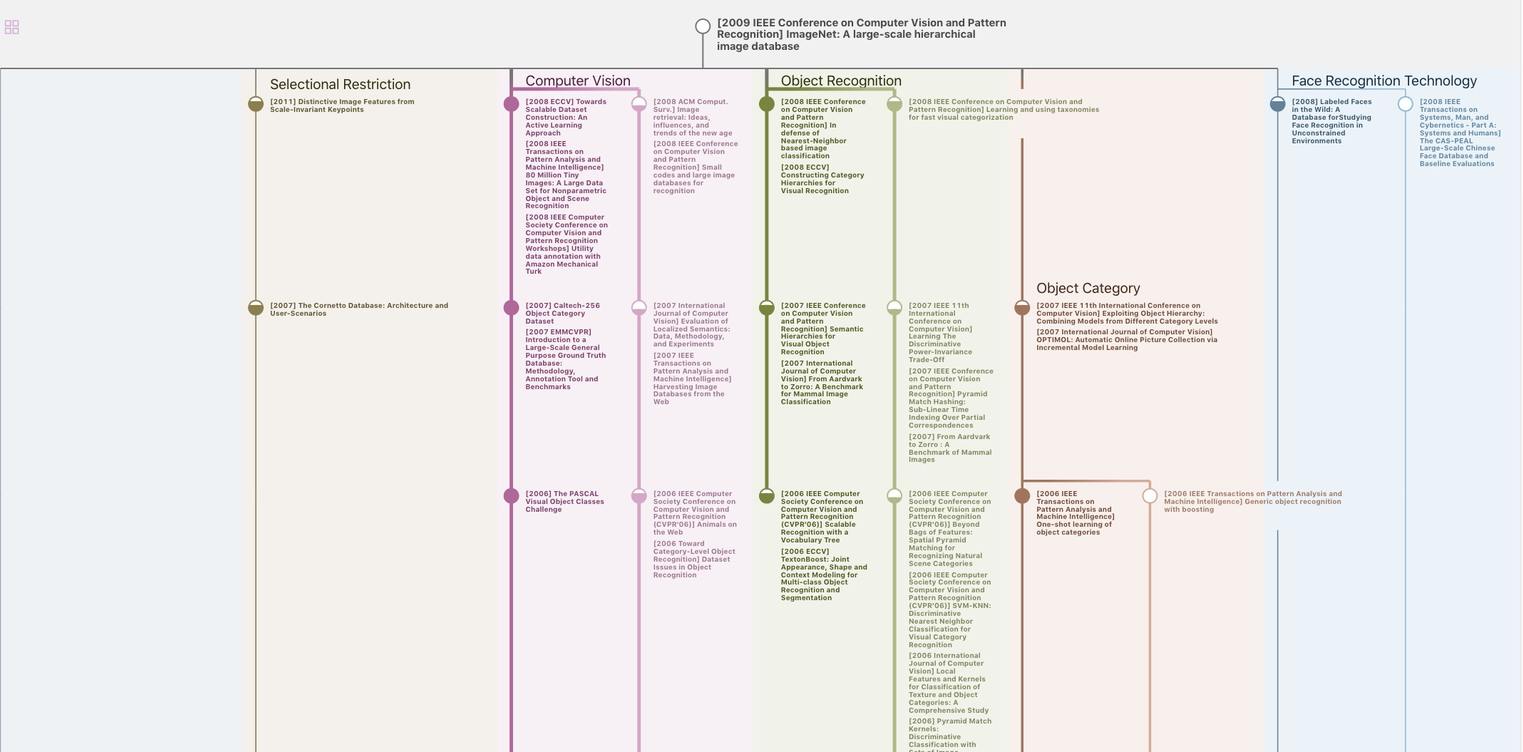
生成溯源树,研究论文发展脉络
Chat Paper
正在生成论文摘要