FilFL: Client Filtering for Optimized Client Participation in Federated Learning
arXiv (Cornell University)(2023)
摘要
Federated learning is an emerging machine learning paradigm that enables clients to train collaboratively without exchanging local data. The clients participating in the training process have a crucial impact on the convergence rate, learning efficiency, and model generalization. In this work, we propose FilFL, a new approach to optimizing client participation and training by introducing client filtering. FilFL periodically filters the available clients to identify a subset that maximizes a combinatorial objective function using an efficient greedy filtering algorithm. From this filtered-in subset, clients are then selected for the training process. We provide a thorough analysis of FilFL convergence in a heterogeneous setting and evaluate its performance across diverse vision and language tasks and realistic federated scenarios with time-varying client availability. Our empirical results demonstrate several benefits of our approach, including improved learning efficiency, faster convergence, and up to 10 percentage points higher test accuracy compared to scenarios where client filtering is not utilized.
更多查看译文
关键词
client filtering,optimized client participation,federated
AI 理解论文
溯源树
样例
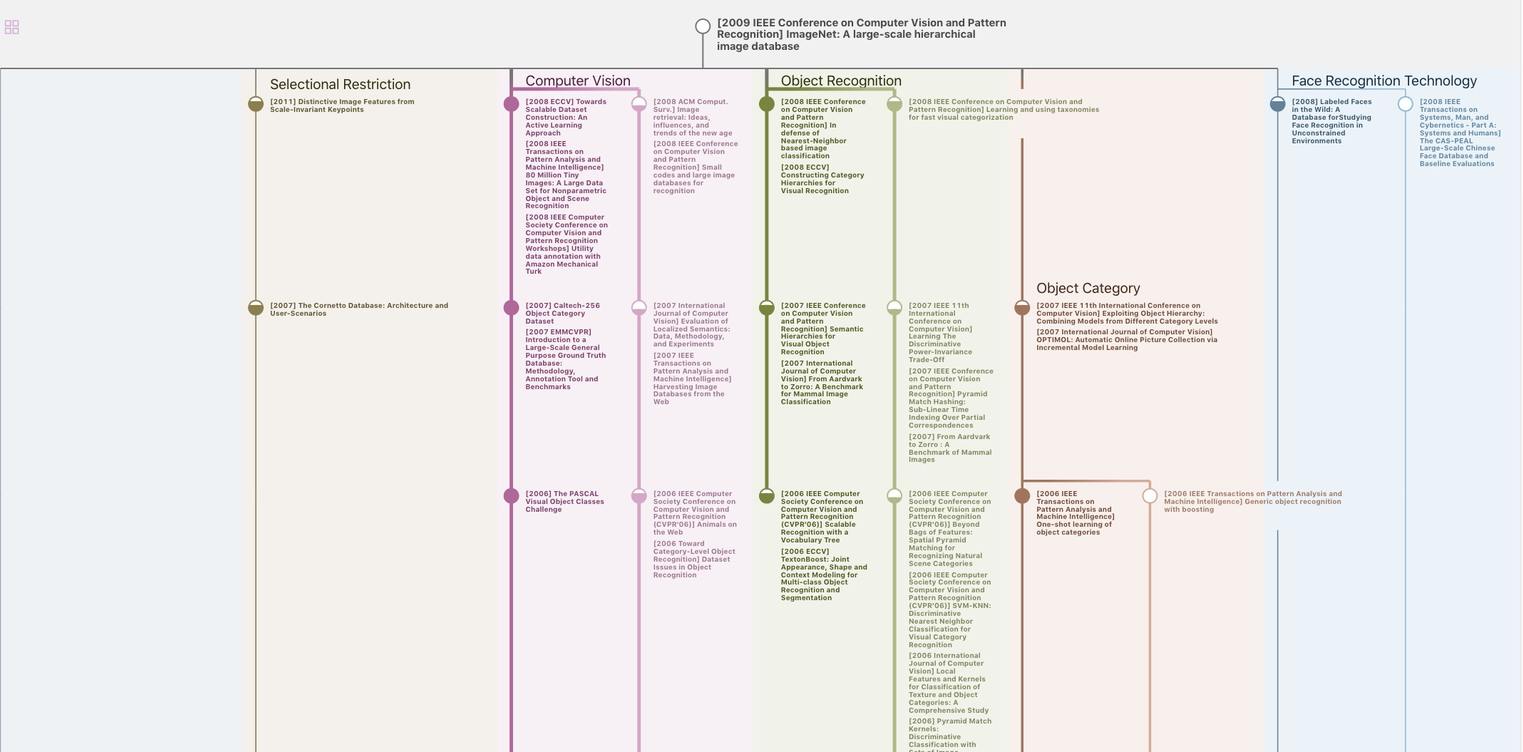
生成溯源树,研究论文发展脉络
Chat Paper
正在生成论文摘要