Learning Vortex Dynamics for Fluid Inference and Prediction
arXiv (Cornell University)(2023)
摘要
We propose a novel differentiable vortex particle (DVP) method to infer and predict fluid dynamics from a single video. Lying at its core is a particle-based latent space to encapsulate the hidden, Lagrangian vortical evolution underpinning the observable, Eulerian flow phenomena. Our differentiable vortex particles are coupled with a learnable, vortex-to-velocity dynamics mapping to effectively capture the complex flow features in a physically-constrained, low-dimensional space. This representation facilitates the learning of a fluid simulator tailored to the input video that can deliver robust, long-term future predictions. The value of our method is twofold: first, our learned simulator enables the inference of hidden physics quantities (e.g., velocity field) purely from visual observation; secondly, it also supports future prediction, constructing the input video's sequel along with its future dynamics evolution. We compare our method with a range of existing methods on both synthetic and real-world videos, demonstrating improved reconstruction quality, visual plausibility, and physical integrity.
更多查看译文
关键词
fluid inference,vortex dynamics,prediction
AI 理解论文
溯源树
样例
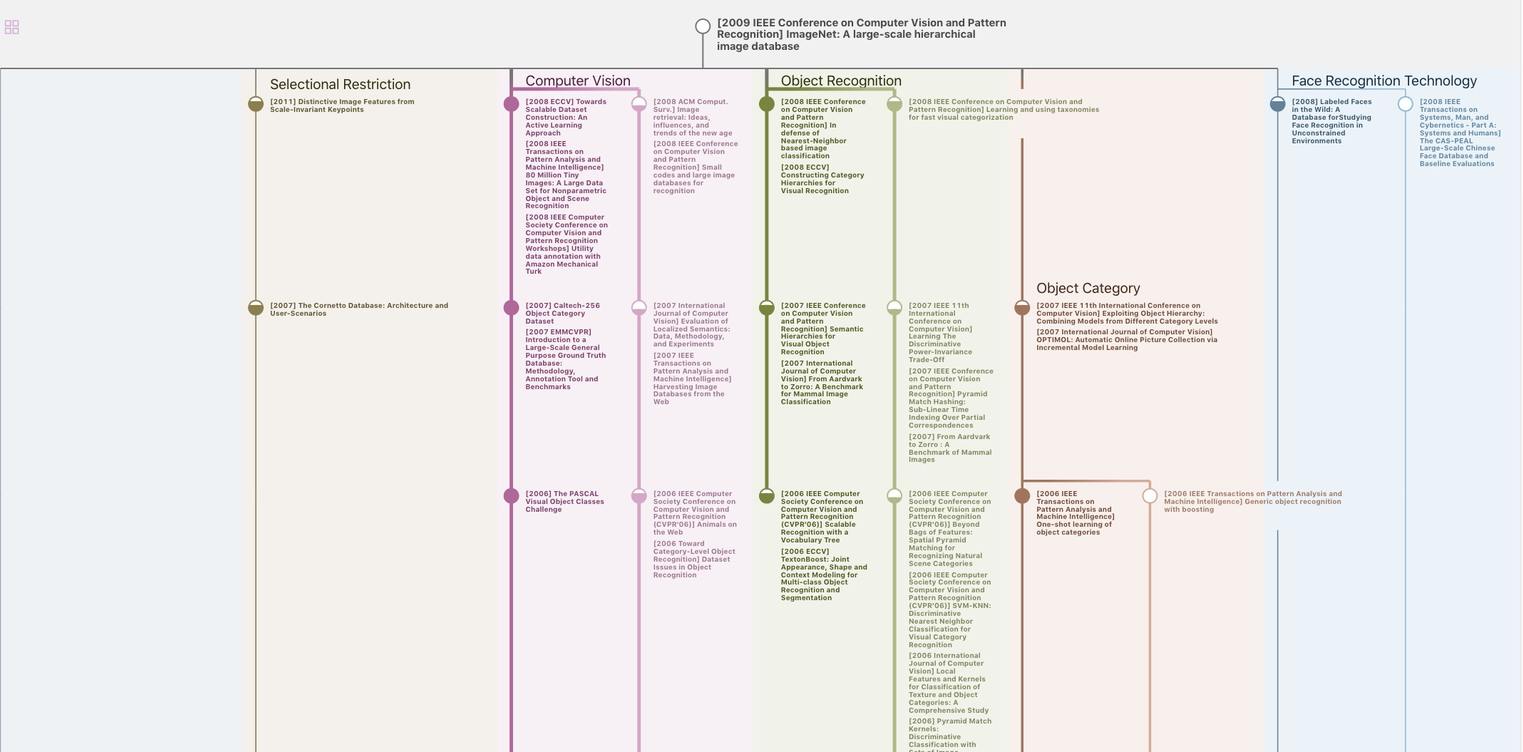
生成溯源树,研究论文发展脉络
Chat Paper
正在生成论文摘要