GLR-SEI: Green and Low Resource Specific Emitter Identification Based on Complex Networks and Fisher Pruning
IEEE transactions on emerging topics in computational intelligence(2023)
Abstract
Better neural networks, more powerful computer hardware and signal Big Data make deep learning increasingly important in Specific Emitter Identification (SEI). However, its implementation uses large amounts of resources and releases CO2. With the requirement of green and low-carbon resource sustainability, deep learning-based SEI faces the great challenge of compressing the model while ensuring its performance. In this article, we propose a novel green and low resource specific emitter identification method using complex networks and Fisher pruning (GLR-SEI). Specifically, we divide the learning process into two stages: knowledge distillation and fisher pruning, which involves transferring knowledge from complex number networks to real number networks. The proposed approach is evaluated using aircraft Automatic Dependent Surveillance Broadcast (ADS-B) data collected in the real world and many pruning experiments are performed. In addition, we add descriptive experiments on Raspberry Pi to provide a more effective data presentation in order to better demonstrate our research results. The experimental results show that the proposed lightweight network in this article can reduce the recognition rate by only 0.7% and the inference time by 10.1% compared to the complex neural network.
MoreTranslated text
Key words
Specific emitter identification,complex networks,knowledge distillation,ADS-B
AI Read Science
Must-Reading Tree
Example
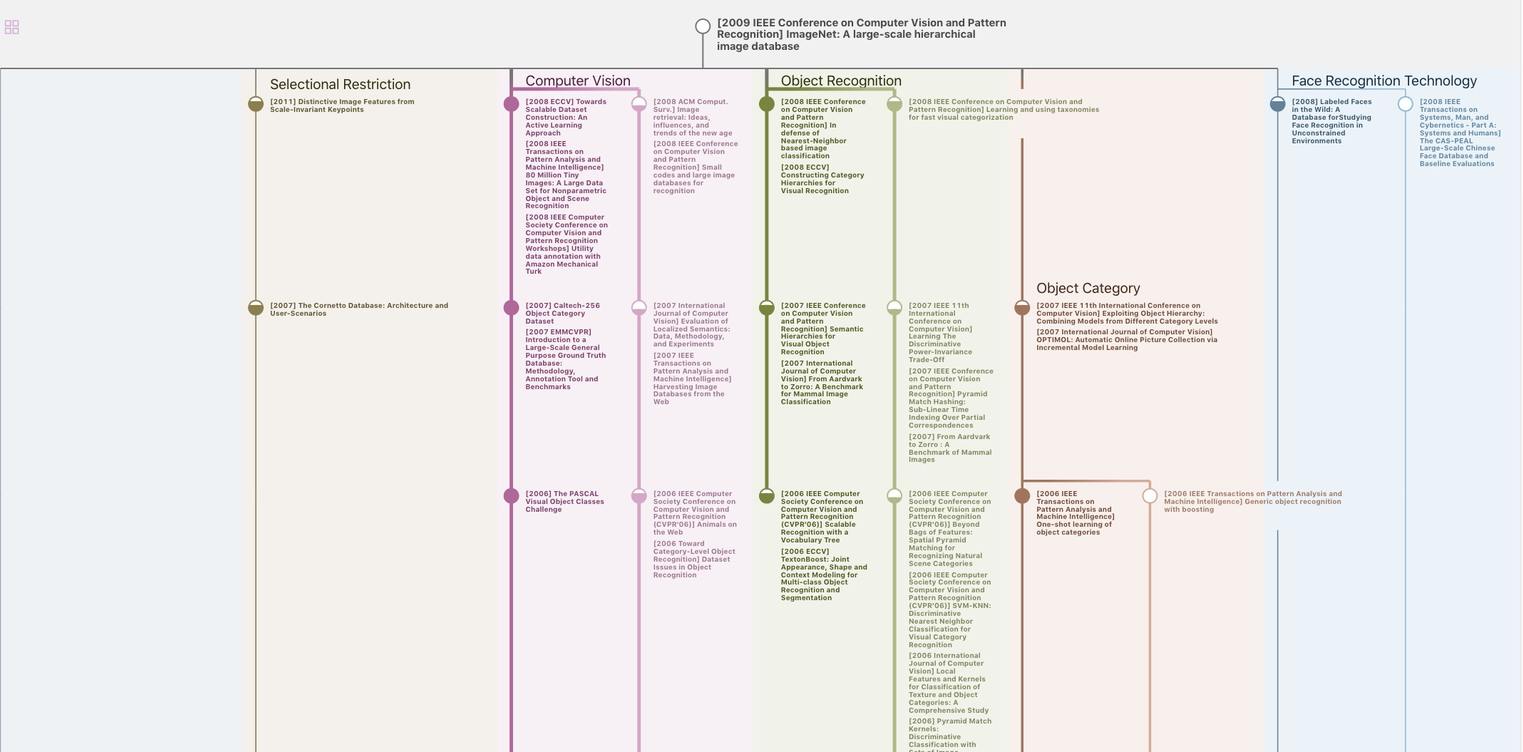
Generate MRT to find the research sequence of this paper
Chat Paper
Summary is being generated by the instructions you defined