An interpretable machine learning framework for modelling macromolecular interaction mechanisms with nuclear magnetic resonance
DIGITAL DISCOVERY(2023)
摘要
Macromolecular interactions, such as polymer-protein binding, determine the biological fate of biomaterials. However, in most macromolecular binding systems, underlying interaction mechanisms are unclear, limiting capabilities for in vitro prediction. In particular, the atomic-level structure-activity relationships that drive protein-polymer binding are confounding. To overcome this gap, we developed a machine learning framework that applies interaction data from direct saturation compensated nuclear magnetic resonance (DISCO NMR) to classify polymer proton descriptors to their interactive behaviors with mucin proteins. The framework constructs structure-interaction trends from cross-polymer atomic-level behavior patterns, and identifies "undervalued" inert polymer groups with potential to be engineered towards interaction. Trends are constructed from materials-agnostic interaction descriptors that combine chemical shift fingerprints, molecular weight, and cumulative DISCO effect from saturation transfer buildup, mapping proton chemical, physical, and conformational attributes together. In this work we constructed a fully-trained decision tree classifier to model structure-activity after applying principal component analysis (accuracy = 0.92, F1 = 0.87) and interpreted its decision rules to improve scientific understanding of mucin binding. Several undervalued inert protons identified by the model include: HPC 80 kDa (4.58 ppm), HPMC 120 kDa (4.48 ppm), PVA 105 kDa (1.58 ppm), DEX 150 kDa (5.20 ppm), PVP 55 kDa (3.89 ppm), CMC 90 kDa (4.58 ppm), and PEOZ 50 kDa (3.42 ppm). The model additionally suggested a structure-activity relationship is shared by HPC, CMC, DEX, and HPMC protons in the 80-150 kDa range. More broadly, the framework and its descriptors can be applied for data-driven discovery of new polymer formulations using previously obscure cross-polymer sub-group trends, and is similarly applicable to any receptor-ligand system compatible with DISCO-NMR screening. We use a glass box approach based on decision trees to understand glycoprotein binding with biomedical polymers.
更多查看译文
关键词
macromolecular interaction mechanisms,interpretable machine learning framework,machine learning,nuclear magnetic resonance,magnetic resonance
AI 理解论文
溯源树
样例
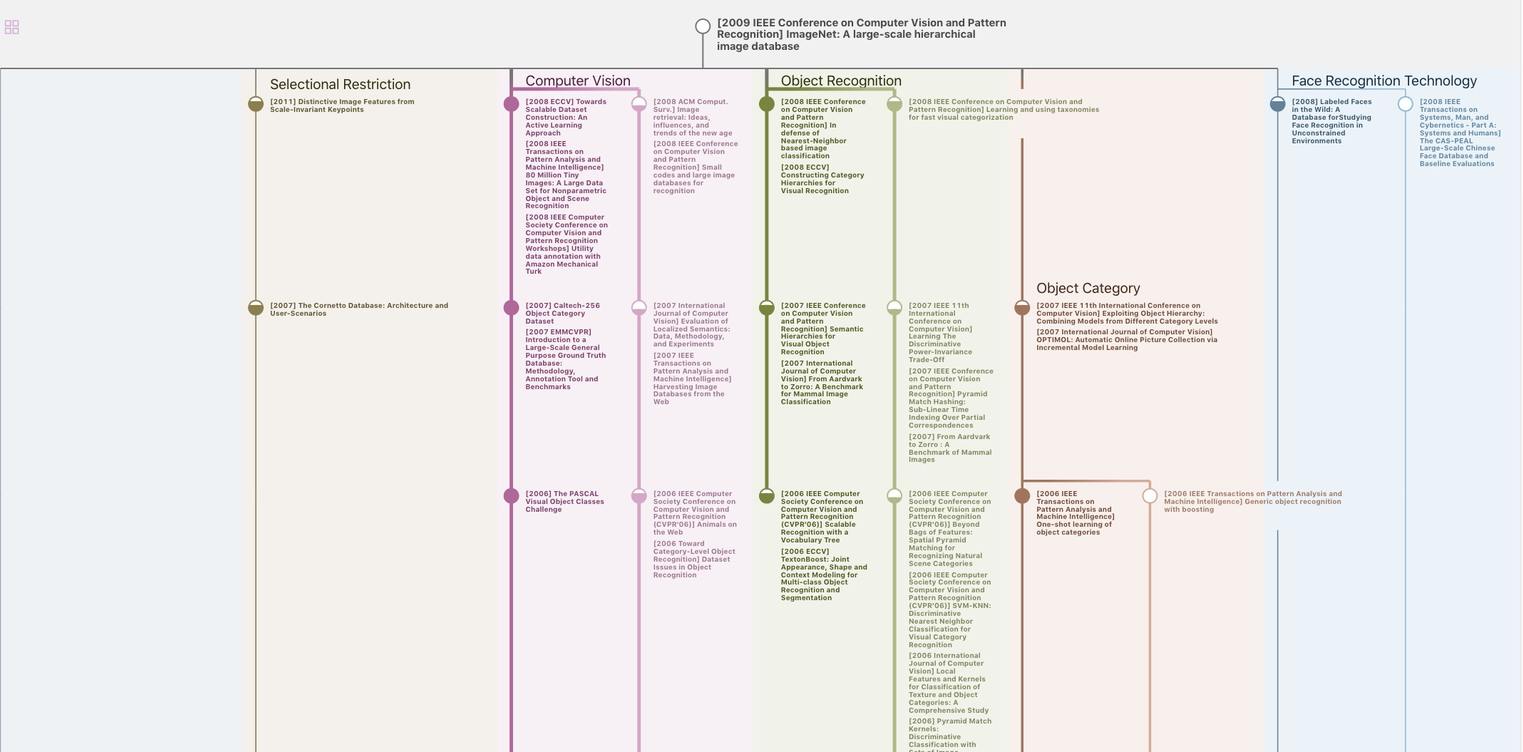
生成溯源树,研究论文发展脉络
Chat Paper
正在生成论文摘要