A Novel Conditional Wasserstein Deep Convolutional Generative Adversarial Network
IEEE transactions on artificial intelligence(2023)
摘要
Generative Adversarial Networks (GAN) and their several variants have not only been used for adversarial purposes but also used for extending the learning coverage of different AI/ML models. Most of these variants are
unconditional
and do not have enough control over their outputs.
Conditional
GANs (CGANs) have the ability to control their outputs by
conditioning
their
generator
and
discriminator
with an auxiliary variable (such as
class label
s, and
text description
s). However, CGANs have several drawbacks such as
unstable training
,
non-convergence
and multiple
mode collapse
s like other
unconditional
basic GANs (where the
discriminator
s are
classifier
s). DCGANs, WGANs, and MMDGANs enforce significant improvements to stabilize the GAN training although have no control over their outputs. We developed a novel
conditional
Wasserstein GAN model, called CWGAN (
a.k.a
RD-GAN
named after the initials of the authors' surnames
) that stabilizes GAN training by replacing
relatively unstable
JS divergence with Wasserstein-1 distance while maintaining better control over its outputs. We have shown that the CWGAN can produce optimal
generator
s and
discriminator
s irrespective of the original and input noise data distributions. We presented a detailed formulation of CWGAN and highlighted its salient features along with proper justifications. We showed the CWGAN has a wide variety of adversarial applications including preparing
fake
images through a CWGAN-based
deep generative hashing function
and generating highly accurate user mouse trajectories for fooling any underlying mouse dynamics authentications (MDAs). We conducted detailed experiments using well-known benchmark datasets in support of our claims.
更多查看译文
关键词
CGANs,WGANs,DCGANs,RD-GAN
AI 理解论文
溯源树
样例
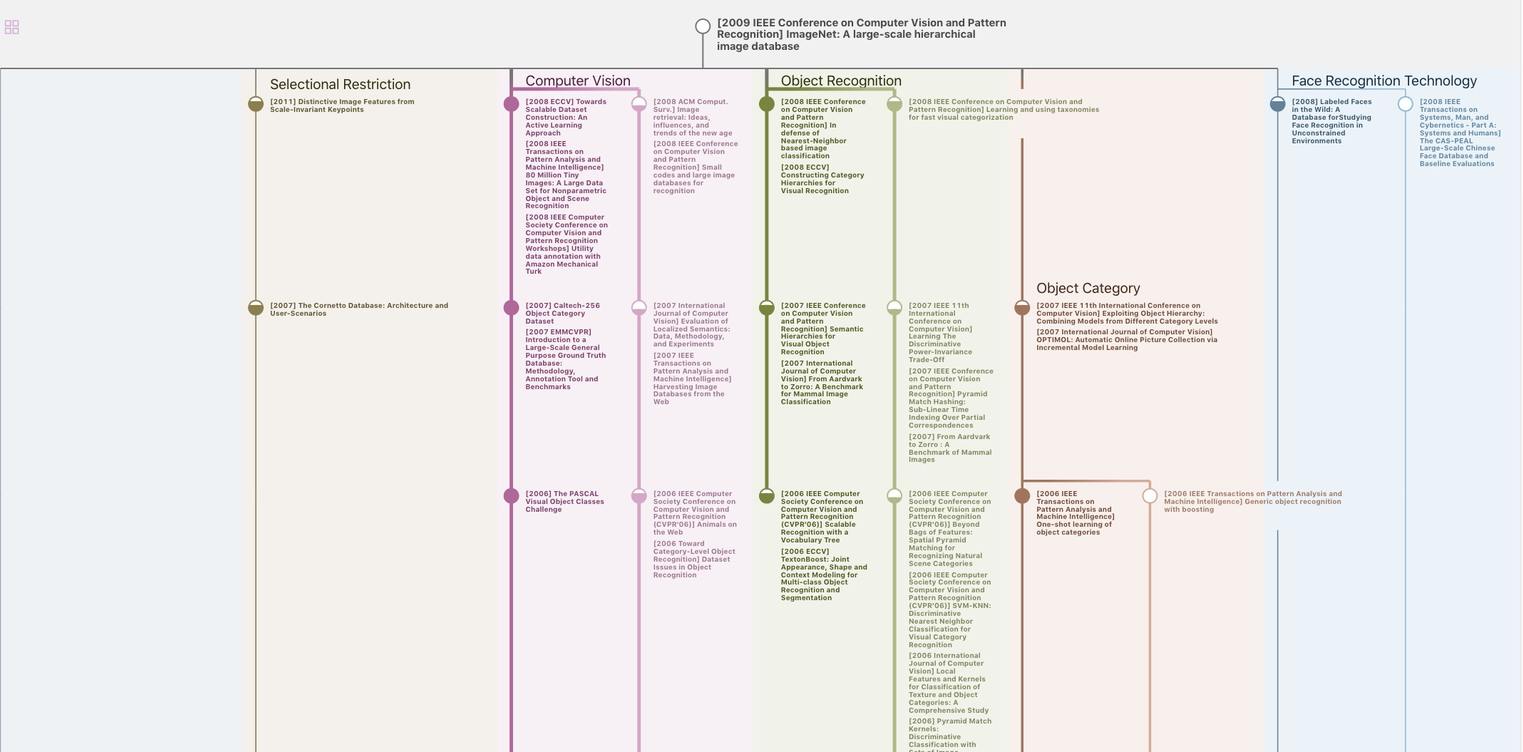
生成溯源树,研究论文发展脉络
Chat Paper
正在生成论文摘要