Prediction of Heart Disease and Survivability using Support Vector Machine and Naive Bayes Algorithm
bioRxiv (Cold Spring Harbor Laboratory)(2023)
Abstract
Purpose In the present work, we examined the outcomes and accuracy of the Support vector machine (SVM) and the Naive Bayes algorithms on a dataset, to predict whether the patient has heart disease or not, and the patient’s survival prediction status.
Method The machine learning procedures were developed using the clinically validated datasets with sixteen attributes from the University of California, Irvine’s Centre for Machine Learning, and Intelligent Systems. Confusion matrix was used to visualise the accuracy, recall, precision, and error of the models. Statistical analysis was done to prove the model accuracy using the receiver operating characteristic (ROC) curve and area under the curve (AUC).
Results The proposed method of heart disease prediction using Naïve Bayes had 87 % accuracy. The accuracy for heart survivability models using SVM and Naïve Bayes were 88 % and 93 %. The model efficiency for heart survivability using ROC curve with AUC 0.93 for Naïve Bayes and AUC 0.91 for SVM.
Conclusion Such prediction systems can help the medical sector to save energy, cost, and time by providing more efficient techniques to forecast decisions with high accuracy. This study will enable the statisticians and researchers to select more efficient and accurate machine learning algorithms to achieve better prediction of the “cardiovascular disease”.
### Competing Interest Statement
The authors have declared no competing interest.
MoreTranslated text
AI Read Science
Must-Reading Tree
Example
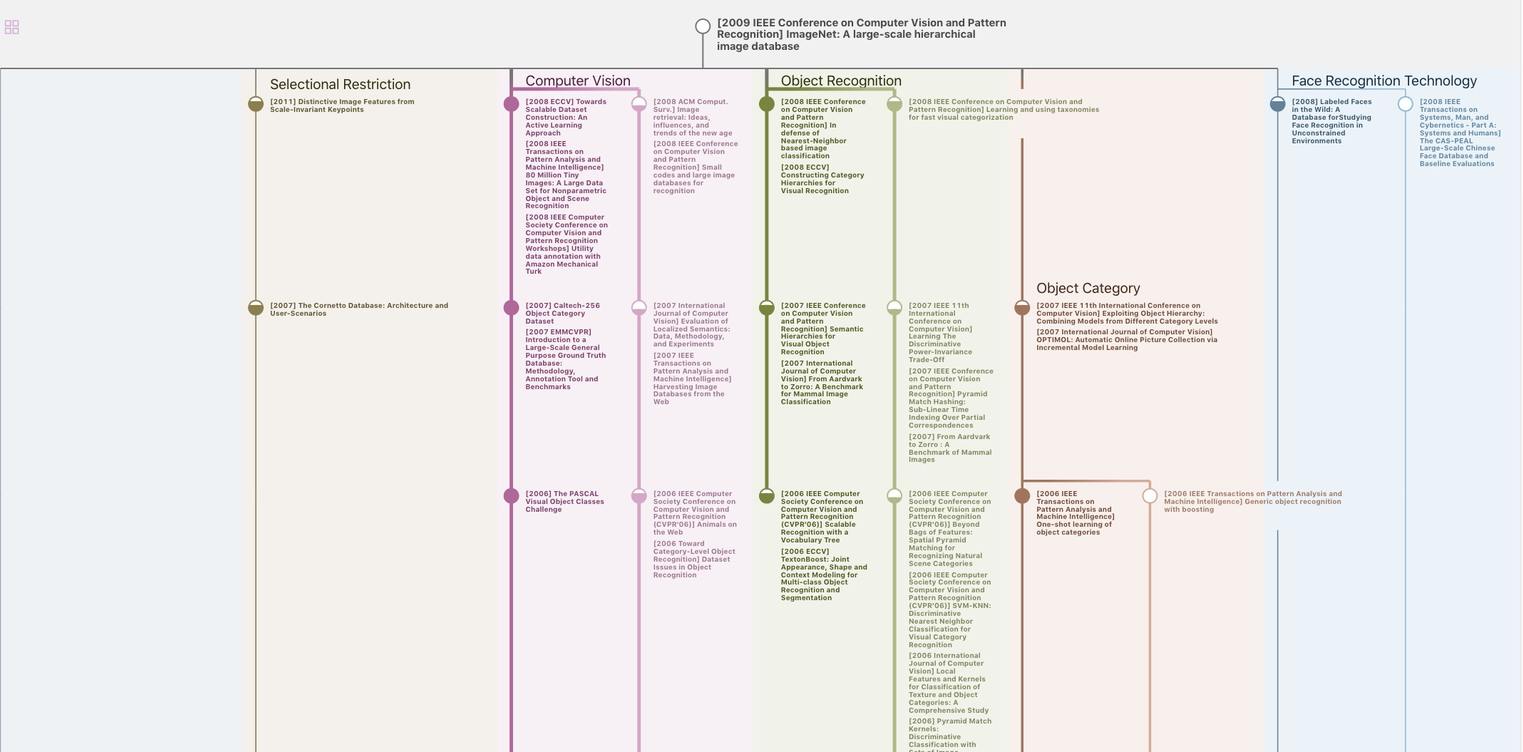
Generate MRT to find the research sequence of this paper
Chat Paper
Summary is being generated by the instructions you defined