Successor Feature-Based Transfer Reinforcement Learning for Video Rate Adaptation With Heterogeneous QoE Preferences.
IEEE Trans. Multim.(2024)
摘要
In adaptive video streaming, the design of an adaptive bitrate (ABR) strategy is critical for the quality-of-experience (QoE) perceived by users. Though current learning-based ABR algorithms achieve state-of-the-art performance for users with a given QoE metric setting for training, they may unfortunately suffer the poor generalization issue for other users with different QoE preferences. Besides, how to quantitatively characterize the distinct QoE preference for a user has also not been extensively studied yet. In this paper, we propose STEER, a successor feature-based transfer reinforcement learning framework for fast learning the ABR strategies on heterogeneous QoE preferences. Specifically, we first develop a QoE preference analysis scheme to infer the personal QoE preference of a single user based on the user's actual viewing history. We then formulate the personalized QoE maximization problem as a reinforcement learning (RL) task, which optimizes the ABR strategy to maximize the overall QoE perceived by the user. Further, we model the QoE maximization problem for multiple users with heterogeneous QoE preferences as a multi-task RL problem, with each task distinguished by the user-distinct QoE preference. To efficiently address this problem, the proposed STEER solves for each RL-based ABR task by learning its optimal successor feature (SF) function, which can be exploited as shared knowledge across tasks to facilitate the transfer between tasks. With SF functions, STEER can quickly evaluate the optimal policies of previously learned tasks on a new task, and further use the generalized policy improvement operation to obtain a jumpstart policy. Both theoretically and empirically, we show that this jumpstart policy is a good initial policy with a performance guarantee for better generalization in the new task, and can also lead to a faster convergence to the optimal policy of the new task.
更多查看译文
关键词
Adaptive video streaming,transfer reinforcement learning,successor features,heterogeneous preferences
AI 理解论文
溯源树
样例
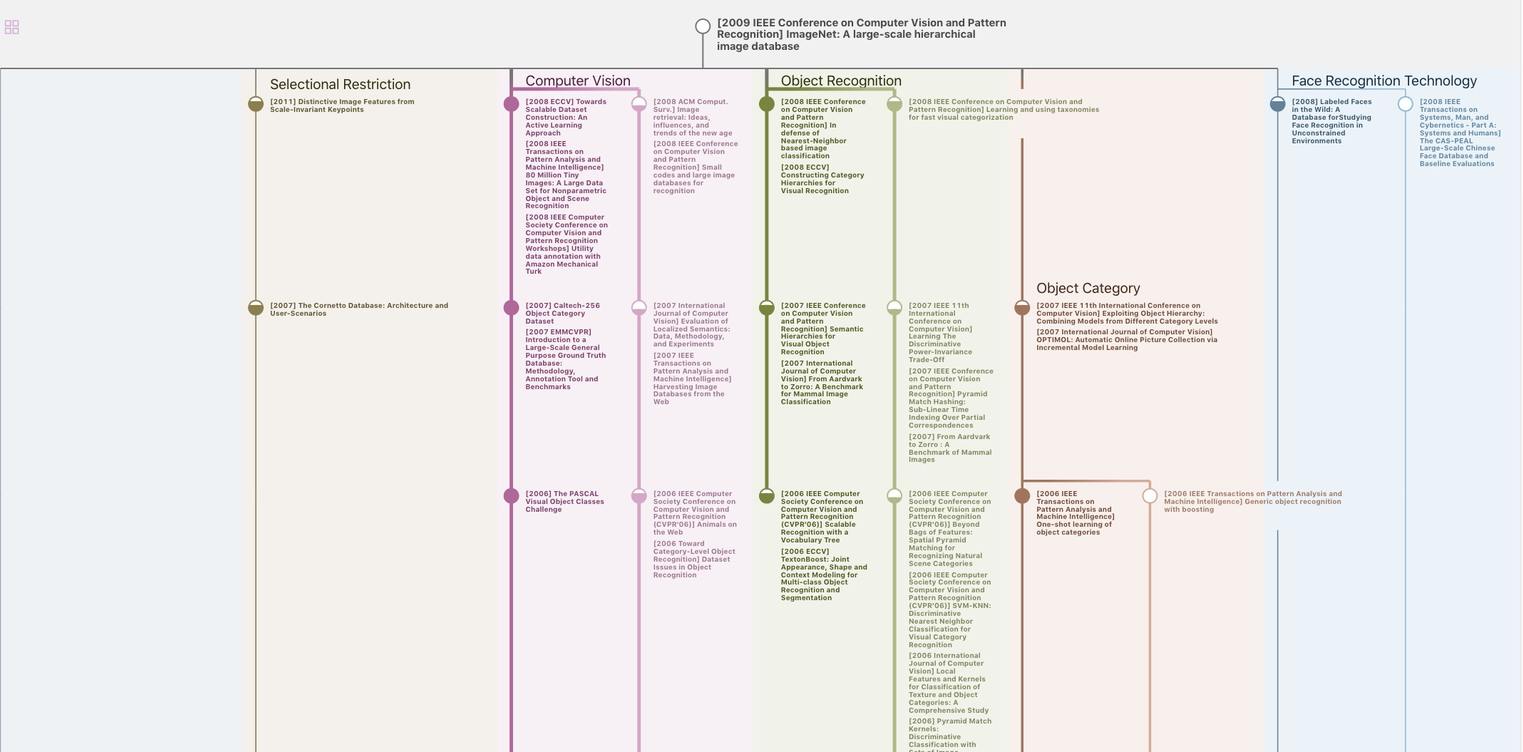
生成溯源树,研究论文发展脉络
Chat Paper
正在生成论文摘要