A Local Overfitting Alleviation Method for Data-Driven Calibration Applied in a 5-DOF Hybrid Robot
Mechanisms and machine science(2023)
摘要
In order to alleviate the local overfitting of back propagation neural network (BPNN) in data-driven calibration, this paper proposes a network optimization method based on the medboost algorithm. In the method, sample weights are introduced into the neural network to alter how different samples participate in the network training, and then numerous BPNNs with diverse learning tendencies are trained. Finally, the best learning machine (BLM) is obtained by screening and combining. The result shows that BLM's maximal loss value on the identical test set is reduced by 35.14% compared to the BPNN's. The robot's maximal position/orientation errors are reduced to 0.079 mm/0.026°, respectively, after calibration. The maximal position/orientation errors are reduced by 29.46%/31.58%, respectively, compared with the method using BPNN.
更多查看译文
关键词
local overfitting alleviation method,calibration,robot,data-driven
AI 理解论文
溯源树
样例
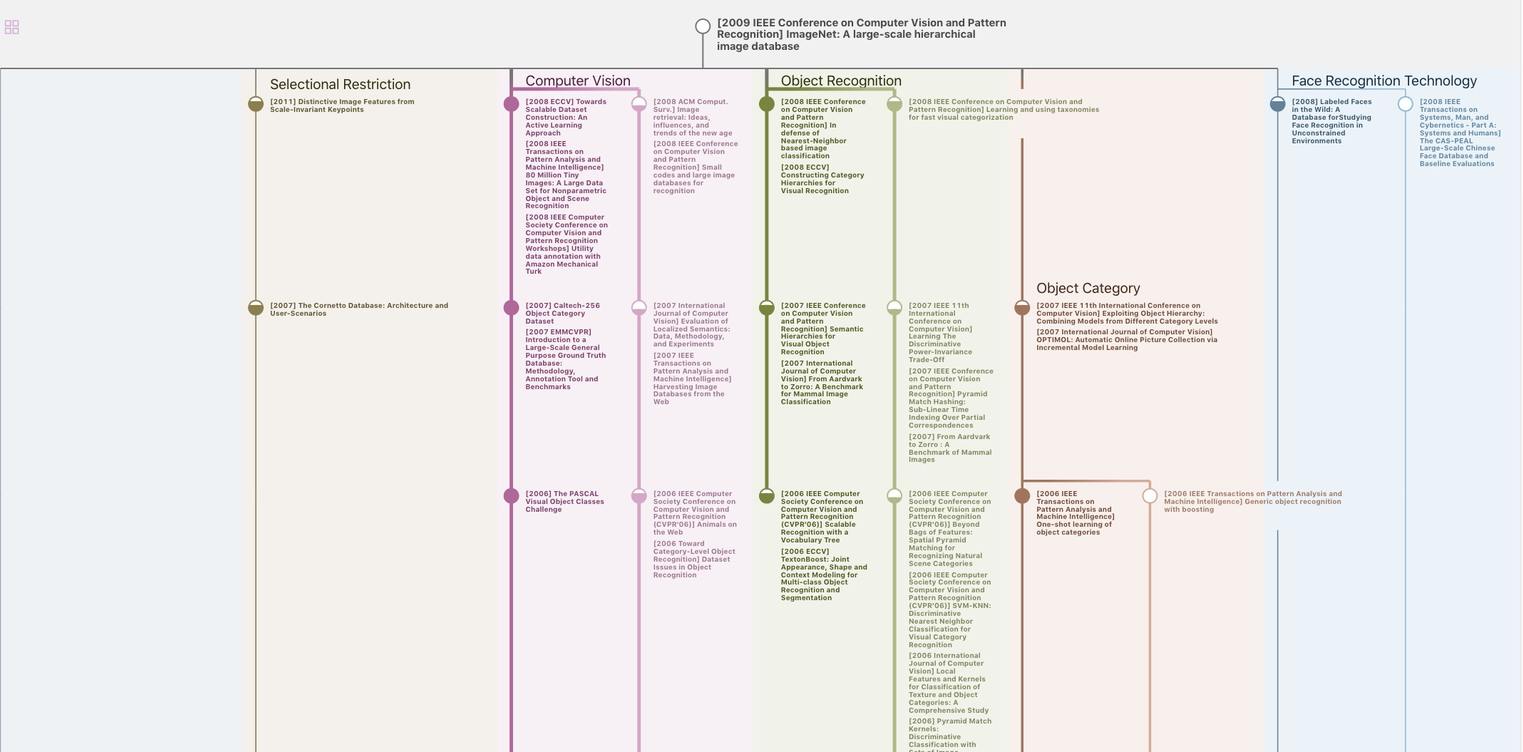
生成溯源树,研究论文发展脉络
Chat Paper
正在生成论文摘要