Stool-Derived Eukaryotic RNA Biomarkers for Predicting Therapeutic Response and Monitoring Mucosal Healing for Patients with Inflammatory Bowel Disease
The American Journal of Gastroenterology(2023)
摘要
Introduction: Inflammatory bowel disease (IBD) is a spectrum of difficult to manage diseases including ulcerative colitis (UC) and Crohn’s disease (CD). IBD can take months to make an initial diagnosis and effectively treat. Methods for diagnosis and treatment primarily include invasive tests such as colonoscopy and sigmoidoscopy. Current noninvasive options (e.g., fecal calprotectin and C-reactive protein) lack sufficient specificity and are not reliable for predicting therapeutic response or monitoring disease status during treatment. Methods: Individuals with refractory Crohn’s Disease were identified for enrollment onto the clinical trial. Stool samples were collected at baseline (T0), 6-14 weeks post treatment (T1), and 52 weeks post treatment (T2). Targeted therapies employed were based on physician recommendations. Survey data, measured using the Crohn's Disease Activity Index (CDAI), were collected at all study time points. Fecal calprotectin, C-reactive protein (CRP), endoscopy, and magnetic resonance imaging (MRI) were obtained when possible. Stool samples were subjected to total RNA extraction and next-generation sequencing. Sequencing data was compared to clinical correlates. Results: In total, 102 stool samples were derived from 68 unique individuals across various time points. Unsupervised hierarchical clustering of seRNA sequencing data identified multiple different transcripts that could be used to segregate individuals based on disease status. These transcripts were employed in a machine learning model to classify disease status based on survey data and biopsy results. The model leveraged 5-fold internal cross validation to determine classifier accuracy across all 102 samples. This model successfully classified individuals as those in remission vs having mild, or moderate/severe disease. Overall model accuracy was 87%. The model showed high classification success (94% accuracy) for moderate/severe disease relative to classification of mild disease (80% accuracy). Receiver operator characteristic (ROC) area under the curve (AUC) for the classifier was 0.86 (Figure 1). Conclusion: We demonstrate that stool-derived RNA biomarkers can be used to noninvasively identify disease status for patients with CD. This noninvasive approach could also be applied to other IBD applications, including predicting therapeutic responses and verifying the efficacy of treatment responses.Figure 1.: A) Unsupervised hierarchical clustering for all patients (n = 102) shows transcripts that were differentially expressed between patients in remission vs patients with active disease. B) Five-fold internal cross validation of a machine learning model was built to stratify patients based on disease status. The model successfully classified patients in remission with 82% accuracy (n = 50) and successfully classified patients with active disease with 90% accuracy (n = 52).
更多查看译文
关键词
eukaryotic rna biomarkers,inflammatory bowel disease,monitoring mucosal healing,therapeutic response,stool-derived
AI 理解论文
溯源树
样例
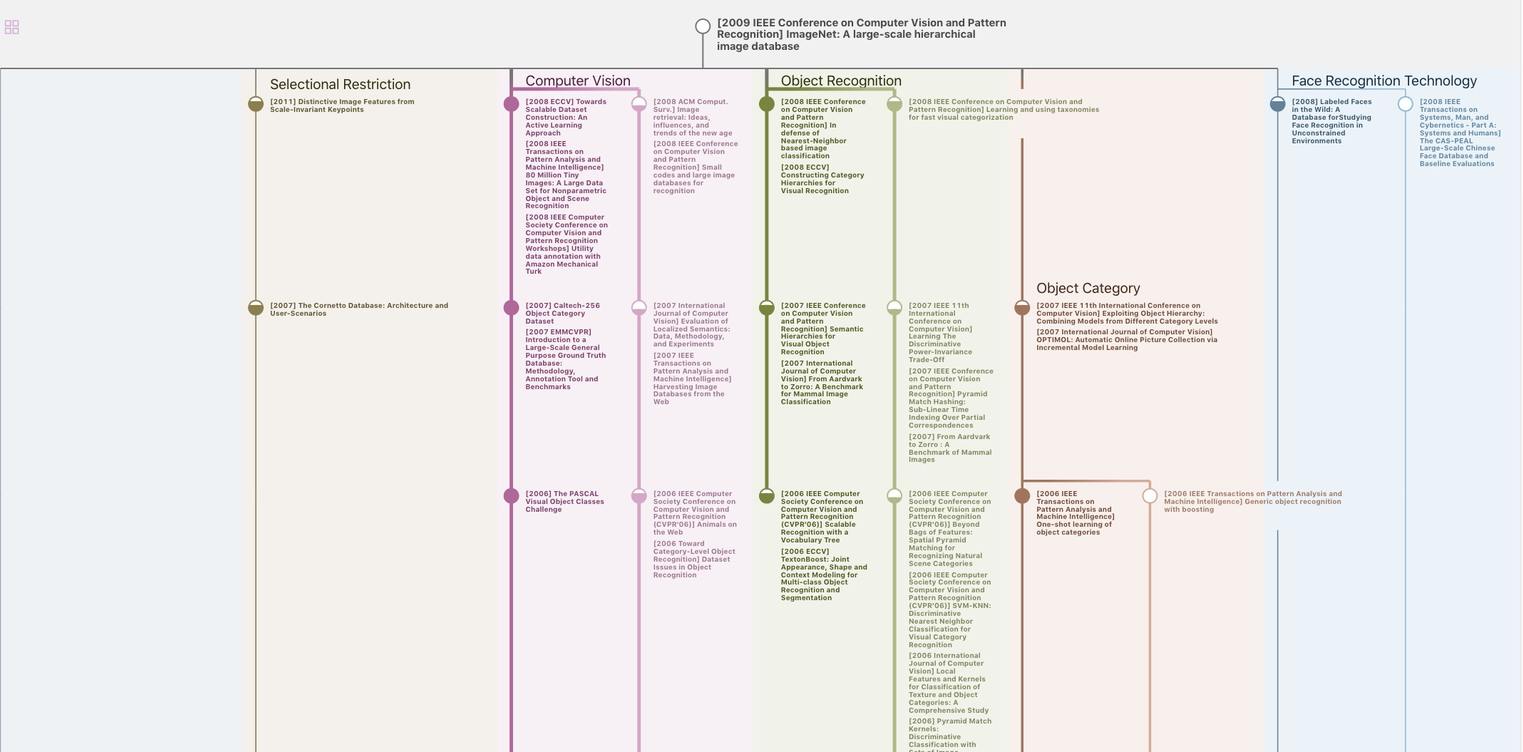
生成溯源树,研究论文发展脉络
Chat Paper
正在生成论文摘要