Predicting Recurrent C. difficile Infection in IBD Patients: An Application of AutoML Tabular and Text Classification Models on Electronic Health Records and Clinical Notes
The American Journal of Gastroenterology(2023)
摘要
Introduction: The utilization of traditional machine learning models on big data demands substantial expertise. To overcome this challenge, Automated Machine Learning (AutoML) has emerged as a promising tool to automate parts of the machine learning pipeline. We evaluated AutoML to predict recurrent C difficile infection (rCDI) in Inflammatory Bowel Disease (IBD) patients. Methods: We included IBD patients with primary CDI and developed 2 models: one leveraging structured Electronic Health Record data for a supervised machine learning model, and another using clinical notes to predict recurrent CDI using natural language processing (NLP) for patient with > 5 clinical notes. Data were processed and uploaded onto a HIPPA compliant Google platform via the Mayo Clinic AI Factory. Data files were formatted to be compatible with the AutoML platform. The dataset was divided into 80/10/10 split for training, validation, and testing sets respectively. For tabular model, performance was evaluated based on the Area under the Receiver Operating Characteristic curve (AuROC), accuracy, precision, and recall and for text classification, the metrics included average precision which is Area under the Precision Recall curve (PR AUC). Results: Of 2,573 patients, 655 (25.4%) had recurrent CDI, an ML model using structured data was trained, validated, and tested on a dataset consisting of 2058, 257, and 257 patients respectively. The model demonstrated promising results to predict rCDI, achieving an AuROC 0.853 and precision and recall, both standing at 78%. (Figure 1A). For the NLP model, we utilized clinical notes from 2,100 patients, of which 508 (24.1%) had rCDI. The model was trained, validated, and tested on a dataset divided into 1680, 210, and 210 items, respectively. The NLP model performed well with PR AUC of 0.827, with both precision and recall at 77.6%. (Figure 1B). We observed similar accuracy and performance with a supervised learning model for tabular data using XGBoost with manual coding (abstract submitted separately to ACG 2023). Conclusion: Leveraging minimal coding and diverse data, we developed high-performing algorithms to predict recurrent CDI using autoML. These models demonstrate comparable performance with traditional machine learning approaches. This emphasizes a promising potential of AutoML in delivering accurate predictions while streamlining the development process.Figure 1.: Performance of Automated Machine Learning models using AutoML Tabular and Text Classification.
更多查看译文
关键词
ibd patients,infection,text classification models,automl tabular,electronic health records
AI 理解论文
溯源树
样例
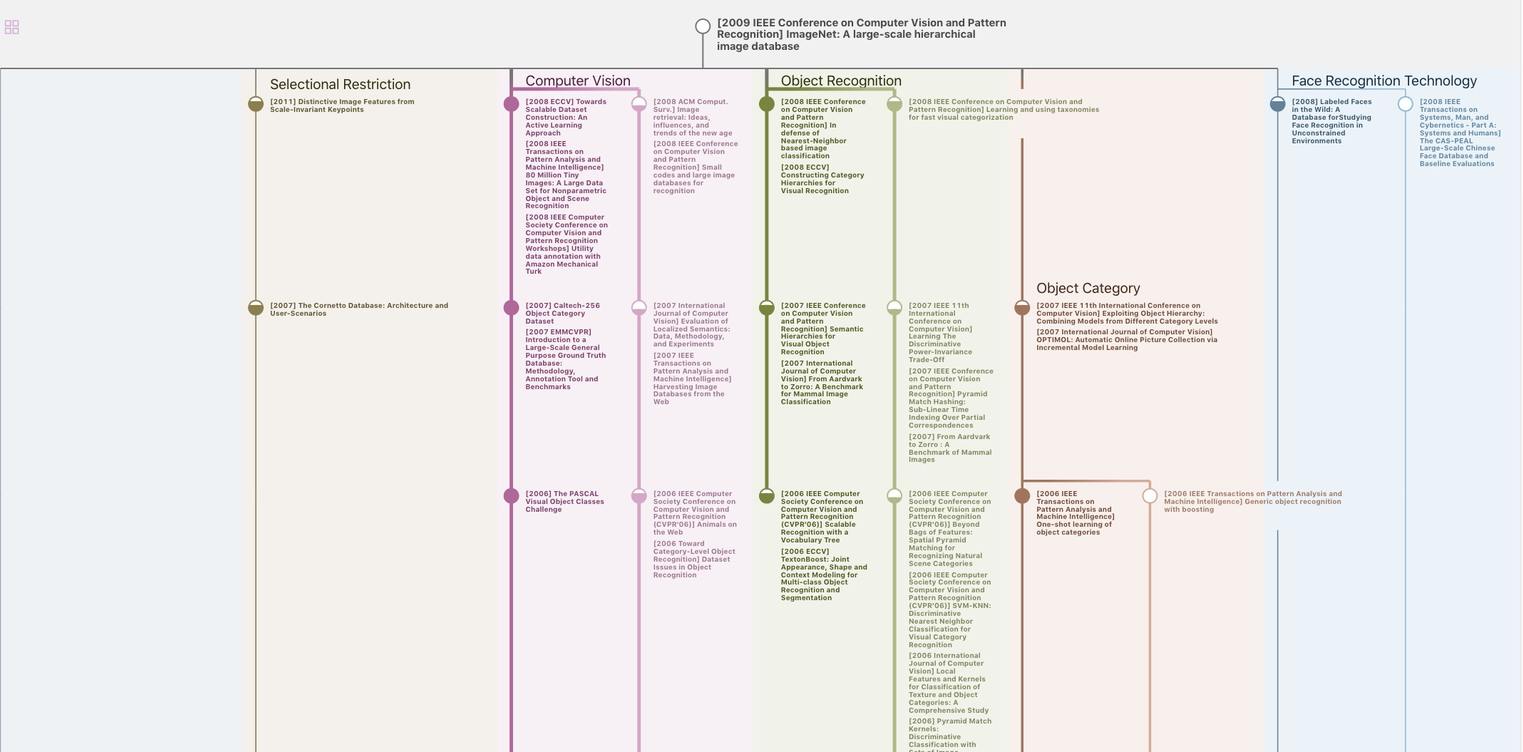
生成溯源树,研究论文发展脉络
Chat Paper
正在生成论文摘要