A Machine Learning-Based Multiple Imputation Method for the Health and Aging Brain Study-Health Disparities
INFORMATICS-BASEL(2023)
摘要
The Health and Aging Brain Study-Health Disparities (HABS-HD) project seeks to understand the biological, social, and environmental factors that impact brain aging among diverse communities. A common issue for HABS-HD is missing data. It is impossible to achieve accurate machine learning (ML) if data contain missing values. Therefore, developing a new imputation methodology has become an urgent task for HABS-HD. The three missing data assumptions, (1) missing completely at random (MCAR), (2) missing at random (MAR), and (3) missing not at random (MNAR), necessitate distinct imputation approaches for each mechanism of missingness. Several popular imputation methods, including listwise deletion, min, mean, predictive mean matching (PMM), classification and regression trees (CART), and missForest, may result in biased outcomes and reduced statistical power when applied to downstream analyses such as testing hypotheses related to clinical variables or utilizing machine learning to predict AD or MCI. Moreover, these commonly used imputation techniques can produce unreliable estimates of missing values if they do not account for the missingness mechanisms or if there is an inconsistency between the imputation method and the missing data mechanism in HABS-HD. Therefore, we proposed a three-step workflow to handle missing data in HABS-HD: (1) missing data evaluation, (2) imputation, and (3) imputation evaluation. First, we explored the missingness in HABS-HD. Then, we developed a machine learning-based multiple imputation method (MLMI) for imputing missing values. We built four ML-based imputation models (support vector machine (SVM), random forest (RF), extreme gradient boosting (XGB), and lasso and elastic-net regularized generalized linear model (GLMNET)) and adapted the four ML-based models to multiple imputations using the simple averaging method. Lastly, we evaluated and compared MLMI with other common methods. Our results showed that the three-step workflow worked well for handling missing values in HABS-HD and the ML-based multiple imputation method outperformed other common methods in terms of prediction performance and change in distribution and correlation. The choice of missing handling methodology has a significant impact on the accompanying statistical analyses of HABS-HD. The conceptual three-step workflow and the ML-based multiple imputation method perform well for our Alzheimer's disease models. They can also be applied to other disease data analyses.
更多查看译文
关键词
Alzheimer's disease,blood biomarkers,machine learning,multiple imputation,missing data
AI 理解论文
溯源树
样例
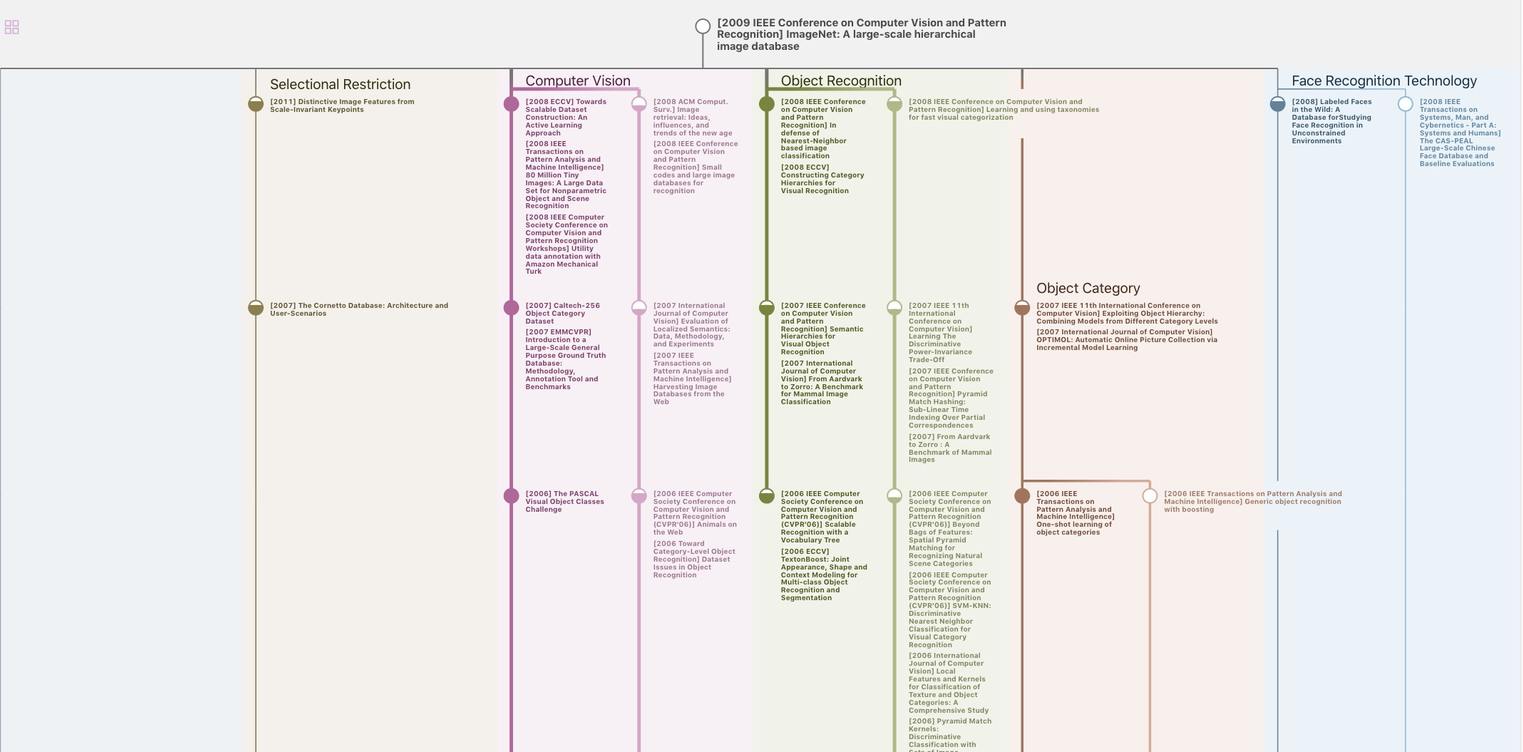
生成溯源树,研究论文发展脉络
Chat Paper
正在生成论文摘要