differential PINO-CDE
MECHANICAL SYSTEMS AND SIGNAL PROCESSING(2024)
摘要
As a fundamental mathematical tool in many engineering disciplines, coupled differential equation groups are being widely used to model complex structures containing multiple physical quantities. Engineers constantly adjust structural parameters at the design stage, which requires a highly efficient solver. The rise of deep learning technologies has offered new perspectives on this task. Unfortunately, existing black-box models suffer from poor accuracy and robustness, while the advanced methodologies of single-output operator regression cannot deal with multiple quantities simultaneously. To address these challenges, we propose PINOCDE, a deep learning framework for solving coupled differential equation groups (CDEs) along with an equation normalization algorithm for performance enhancing. Based on the theory of physics-informed neural operator (PINO), PINO-CDE uses a single network for all quantities in a CDEs, instead of training dozens, or even hundreds of networks as in the existing literature. We demonstrate the flexibility and feasibility of PINO-CDE for one toy example and two engineering applications: vehicle-track coupled dynamics (VTCD) and reliability assessment for a four-storey building (uncertainty propagation). The performance of VTCD indicates that PINO-CDE outperforms existing software and deep learning-based methods in terms of efficiency and precision, respectively. For the uncertainty propagation task, PINO-CDE provides higher resolution results in less than a quarter of the time incurred when using the probability density evolution method (PDEM). This framework integrates engineering dynamics and deep learning technologies and may reveal a new concept for CDEs solving and uncertainty propagation.
更多查看译文
关键词
Coupled differential equation group,Neural operator,Physics-informed deep learning,Uncertainty propagation
AI 理解论文
溯源树
样例
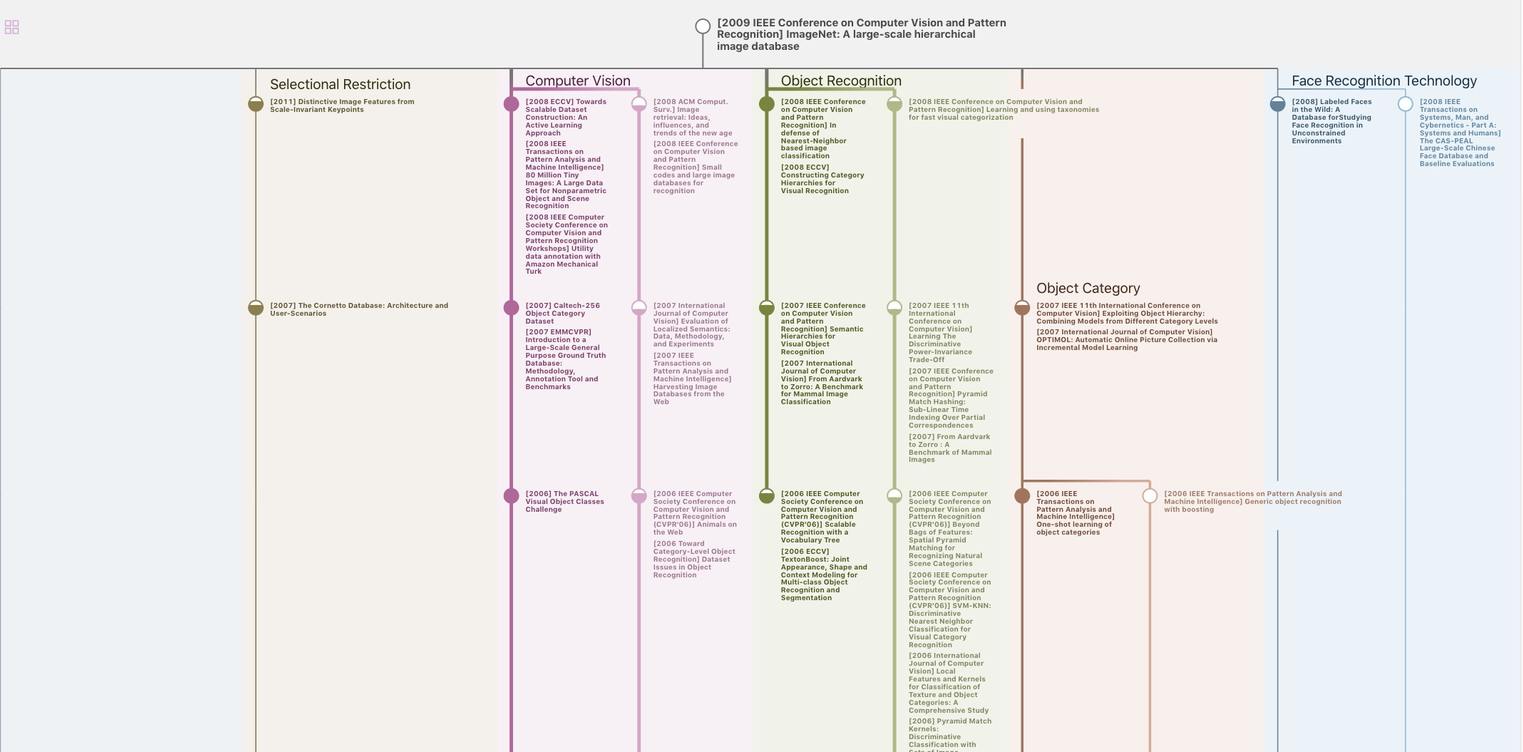
生成溯源树,研究论文发展脉络
Chat Paper
正在生成论文摘要