Reduced Order Modeling
Computational methods in engineering & the sciences(2023)
Abstract
This chapter presents an overview of the most popular reduced order models found in the approximation of partial differential equations and their connection with machine learning techniques. Although the presentation is applicable to many problems in science and engineering, the focus is first-order evolution problems in time and, more specifically, flow problems. Particular emphasis is put on the distinction between intrusive models, which make use of the physical problem being modeled, and non-intrusive models, purely designed from data using machine learning strategies. For the former, models based on proper orthogonal decomposition and Galerkin projection are described in detail, whereas alternatives are only mentioned. Likewise, some modifications that are crucial in the applications are detailed. The progressive incorporation of machine learning methods is described, yielding first hybrid formulations and ending with pure data-driven approaches. An effort has been made to include references with applications of the methods being described.
MoreTranslated text
Key words
modeling,order
AI Read Science
Must-Reading Tree
Example
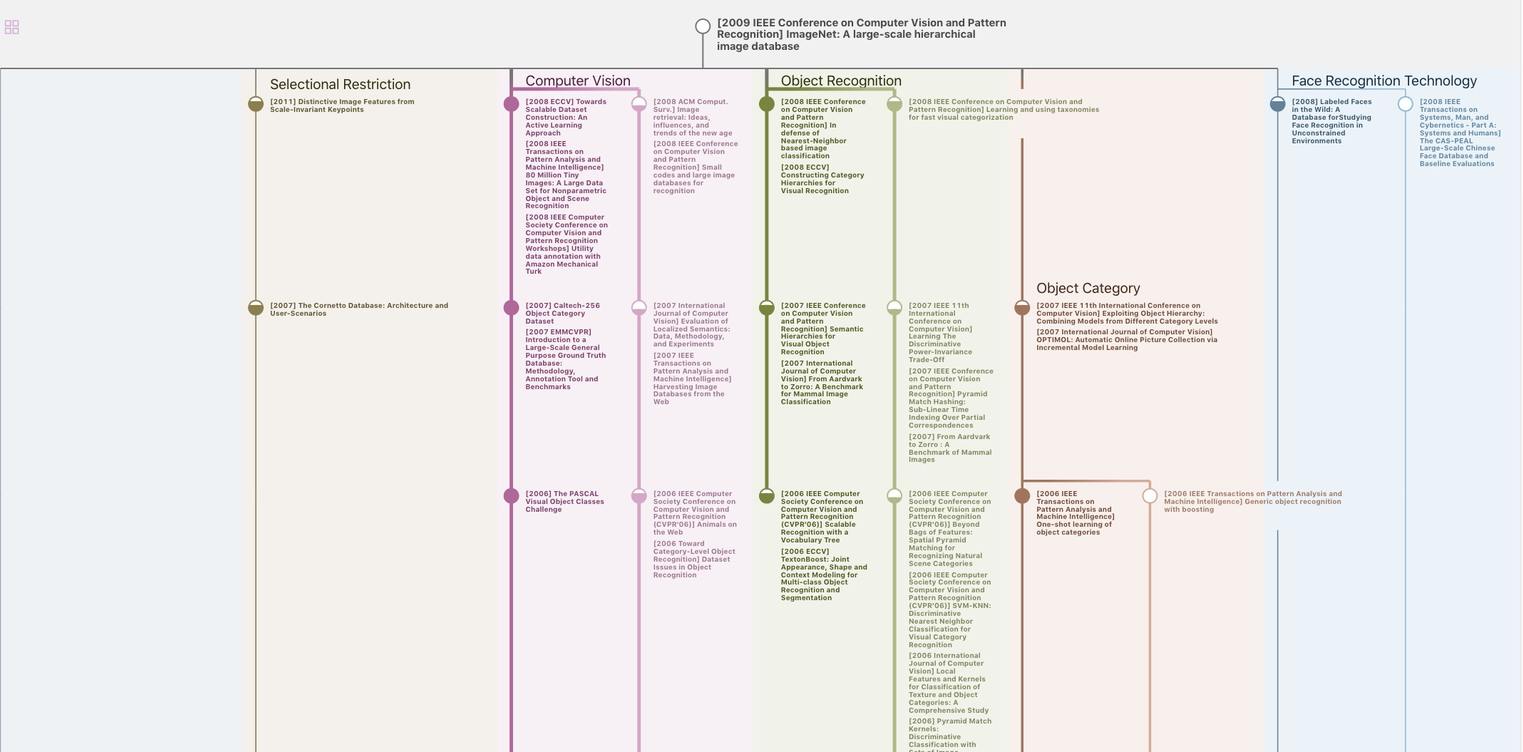
Generate MRT to find the research sequence of this paper
Chat Paper
Summary is being generated by the instructions you defined