From Pixels to Deposits: Porphyry Mineralisation With Multispectral Convolutional Neural Networks
IEEE Journal of Selected Topics in Applied Earth Observations and Remote Sensing(2023)
摘要
Mineral exploration is essential to ensure a sustainable supply of raw materials for modern living and the transition to green. It implies a series of expensive operations that aim to identify areas with natural mineral concentration in the crust of the Earth. The rapid advances in artificial intelligence and remote sensing techniques can help in significantly reducing the cost of these operations. Here, we produce a robust intelligent mineral exploration model that can fingerprint potential locations of porphyry deposits, which are the world's most important source of copper and molybdenum and major source of gold, silver, and tin. We present a deep learning pipeline for assessing multispectral imagery from the Advanced Spaceborne Thermal Emission and Reflection Radiometer (ASTER) with the objective of identifying hydrothermal alterations. Our approach leverages a Convolutional Neural Network (ConvNet) to analyze the high-resolution images, overcoming computational challenges through a patch-based strategy that involves an overlapping window for partitioning the images into fixed-size patches. Through the utilization of manually labeled patches for image classification and identification of hydrothermal alteration areas, our results demonstrate the remarkable ability of ConvNet to accurately detect hydrothermal alterations. The technique is adaptable for other ore deposit models and satellite imagery types, providing a revolution in satellite image interpretation and mineral exploration.
更多查看译文
关键词
porphyry mineralisation,convolutional neural networks,neural networks,deposits
AI 理解论文
溯源树
样例
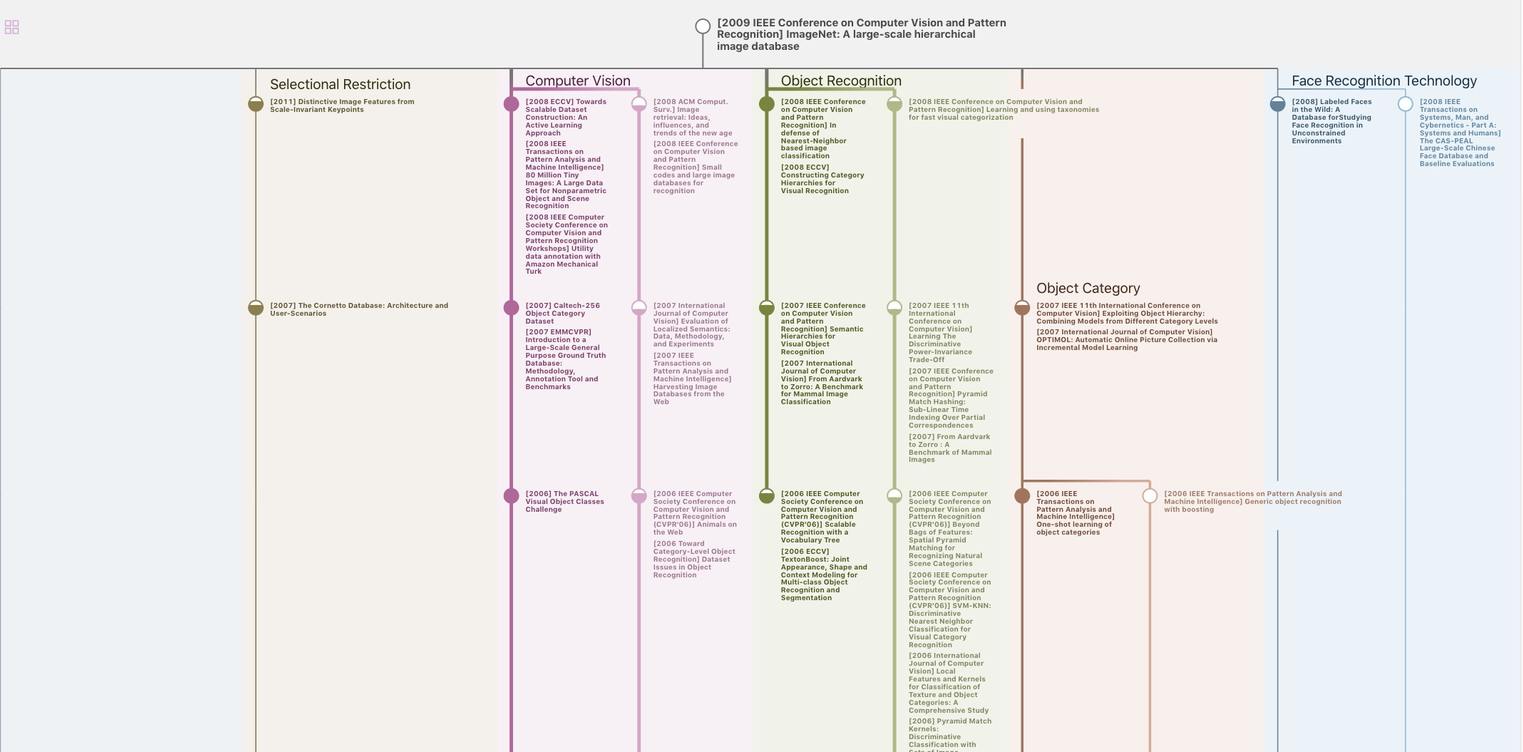
生成溯源树,研究论文发展脉络
Chat Paper
正在生成论文摘要