ALFR++: A Novel Algorithm for Learning Adversarial Fair Representations
Frontiers in artificial intelligence and applications(2023)
摘要
As more decisions in our daily life become automated, the need to have machine learning algorithms that make fair decisions increases. In fair representation learning we are tasked with finding a suitable representation of the data in which a sensitive variable is censored. Recent work aims to learn fair representations through adversarial learning. With this paper, we build upon this work by introducing a novel algorithm, ALFR++, to learn adversarial fair representations independent of the downstream task that may be encountered (supervised or unsupervised). We introduce balanced error rate modulation, to stabilize the interaction between actor and adversary. We couple it with a recently introduced censoring technique called stacking, to learn strong censored representations within a restricted hypothesis space. Results show that our algorithm improves on previous work in both censoring and reconstruction, allowing us to create fairer data without knowing the task beforehand.
更多查看译文
关键词
representations,learning,novel algorithm
AI 理解论文
溯源树
样例
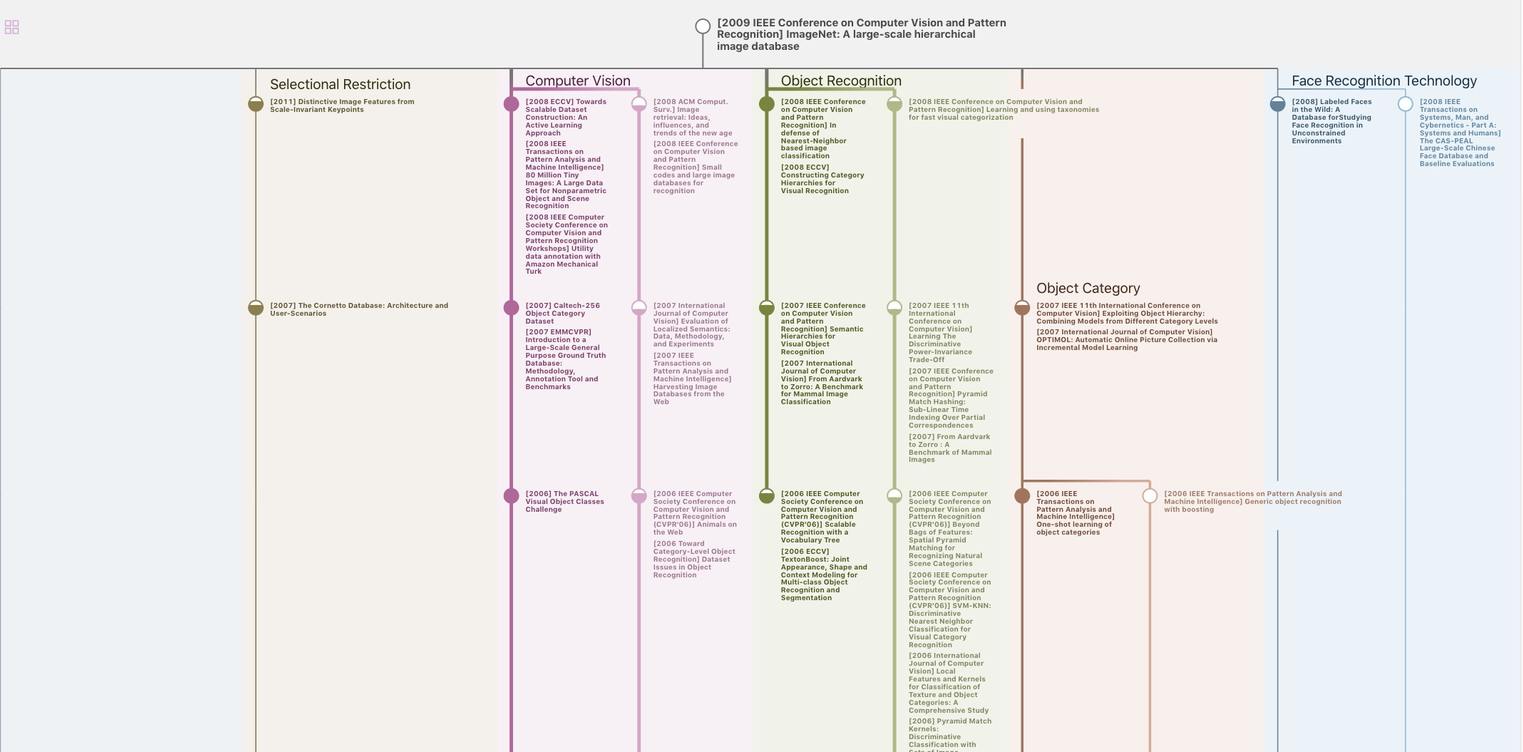
生成溯源树,研究论文发展脉络
Chat Paper
正在生成论文摘要