GenTC: Generative Transformer via Contrastive Learning for Receipt Information Extraction
ARTIFICIAL NEURAL NETWORKS AND MACHINE LEARNING, ICANN 2023, PT VI(2023)
摘要
Information Extraction from visually rich documents has attracted increasing attention due to its various advanced applications in the real world. Most existing methods employ sequence labeling models to solve this problem. However, these approaches suffer from error propagation problems, especially when dealing with noisy OCR results. For this reason, this paper proposes GenTC, a Generative Transformer enhanced by Contrastive learning for receipt information extraction. GenTC extracts structural information in a generative manner. In addition, since the optimization objective is inconsistent with the task, we use an entity-order perturbation and optimize the model with contrastive learning to mitigate the incorrect bias. GenTC is able to tolerate annotation errors in OCR results, which is vital because correct annotation of numerous documents is laborious and expensive. Extensive experiments on three public benchmark datasets demonstrate that GenTC achieves competitive performance compared with previous state-of-the-art methods, and outperforms them by a large margin, especially in realistic scenarios.
更多查看译文
关键词
Document understanding,Key information extraction,Visually rich documents,Generative transformer
AI 理解论文
溯源树
样例
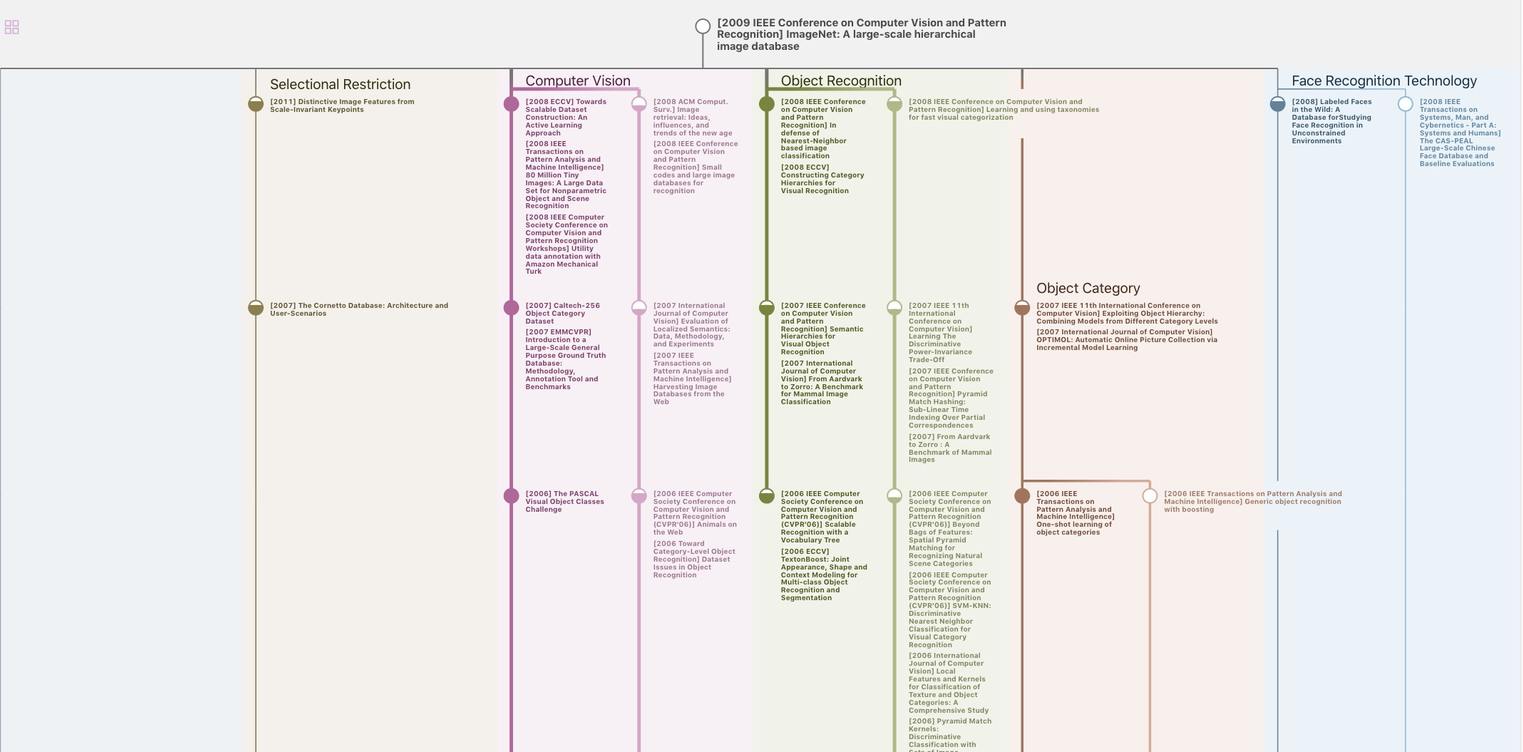
生成溯源树,研究论文发展脉络
Chat Paper
正在生成论文摘要