Deep embedded clustering generalisability and adaptation for mixed datatypes: two critical care cohorts
Research Square (Research Square)(2023)
摘要
Abstract We propose X-DEC, a novel deep clustering technique that can integrate mixed datatypes (in this study numerical and categorical variables). Deep Embedded Clustering (DEC) is a promising technique capable of managing extensive sets of variables and non-linear relationships. Nevertheless, DEC cannot adequately handle mixed datatypes. Therefore, we created X-DEC by replacing the autoencoder with an X-shaped variational autoencoder (XVAE) and optimising hyperparameters for cluster stability. We compared DEC and X-DEC by reproducing a previous study that used DEC to identify clusters in a population of intensive care patients. We assessed internal validity based on cluster stability on the development dataset. Since generalisability of clustering models has insufficiently been validated on external populations, we assessed external validity by investigating cluster generalisability onto an external validation dataset. We concluded that both DEC and X-DEC resulted in clinically recognisable and generalisable clusters, but X-DEC produced much more stable clusters.
更多查看译文
关键词
mixed datatypes,clustering,deep
AI 理解论文
溯源树
样例
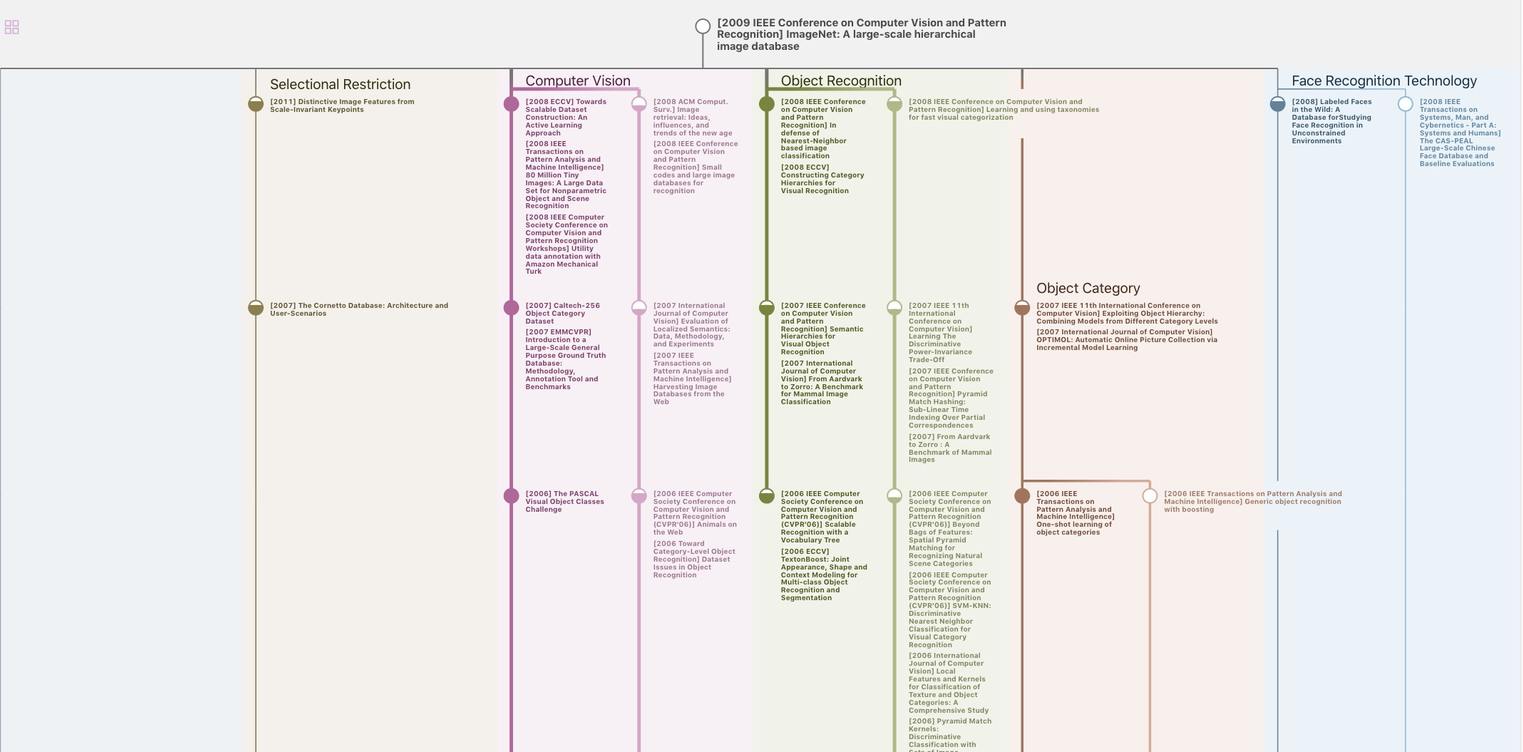
生成溯源树,研究论文发展脉络
Chat Paper
正在生成论文摘要