Dual-Balancing for Multi-Task Learning
arXiv (Cornell University)(2023)
摘要
Multi-task learning (MTL), a learning paradigm to learn multiple related tasks simultaneously, has achieved great success in various fields. However, task balancing problem remains a significant challenge in MTL, with the disparity in loss/gradient scales often leading to performance compromises. In this paper, we propose a Dual-Balancing Multi-Task Learning (DB-MTL) method to alleviate the task balancing problem from both loss and gradient perspectives. Specifically, DB-MTL ensures loss-scale balancing by performing a logarithm transformation on each task loss, and guarantees gradient-magnitude balancing via normalizing all task gradients to the same magnitude as the maximum gradient norm. Extensive experiments conducted on several benchmark datasets consistently demonstrate the state-of-the-art performance of DB-MTL.
更多查看译文
关键词
learning,dual-balancing,multi-task
AI 理解论文
溯源树
样例
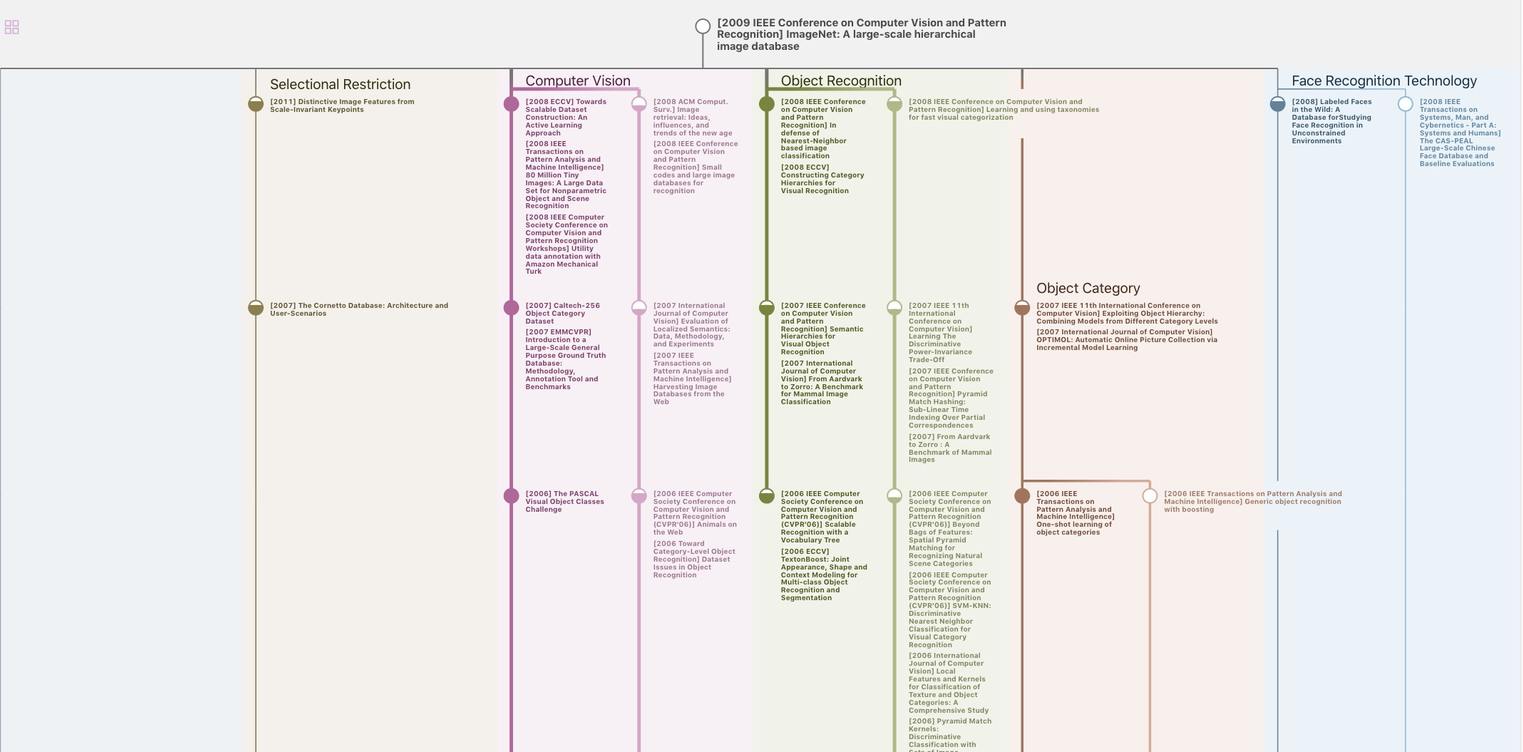
生成溯源树,研究论文发展脉络
Chat Paper
正在生成论文摘要