Towards Flexible and Adaptive Neural Process for Cold-Start Recommendation.
IEEE Trans. Knowl. Data Eng.(2024)
摘要
Recommender systems have been widely adopted in various online personal e-commerce applications for improving user experience. A long-standing challenge in recommender systems is how to provide accurate recommendation to users in cold-start situations where only a few user-item interactions can be observed. Recently, meta learning methods provide a promising solution, and most of them follow a way of parameter initialization where predictions can be fast adapted via multiple gradient descent steps. While these meta-learning recommenders promote model performance, how to derive a fundamental paradigm that enables both flexible approximations of complex user interaction distributions and effective task adaptations of global knowledge still remains a critical yet under-explored problem. To this end, we present the Flow-based Adaptive Neural Process (FANP), a new probabilistic meta-learning model where estimating the preference of each user is governed by an underlying stochastic process. Following an encoder-decoder generative framework, FANP is an effective few-shot function estimator that directly maps limited user interactions to a predictive distribution without complicated gradient updates. Through introducing a conditional normalization flow-based encoder, FANP can get rid of the model bias on latent variables and thereby derive more flexible variational distributions. Meanwhile, we propose a task-adaptive mechanism capturing the relevance of different tasks for improving adaptation ability of global knowledge. The learned task-specific and task-relevant representations are simultaneously exploited to generate the decoder parameters via a novel modulation-augmented hypernetwork. FANP is evaluated on both scenario-specific and user-specific cold-start recommendations on various real-world datasets. Extensive experimental results and detailed model analyses demonstrate that our model yields superior performance compared with multiple state-of-the-art meta-learning recommenders.
更多查看译文
关键词
Cold-start recommendation,meta learning,neural processes
AI 理解论文
溯源树
样例
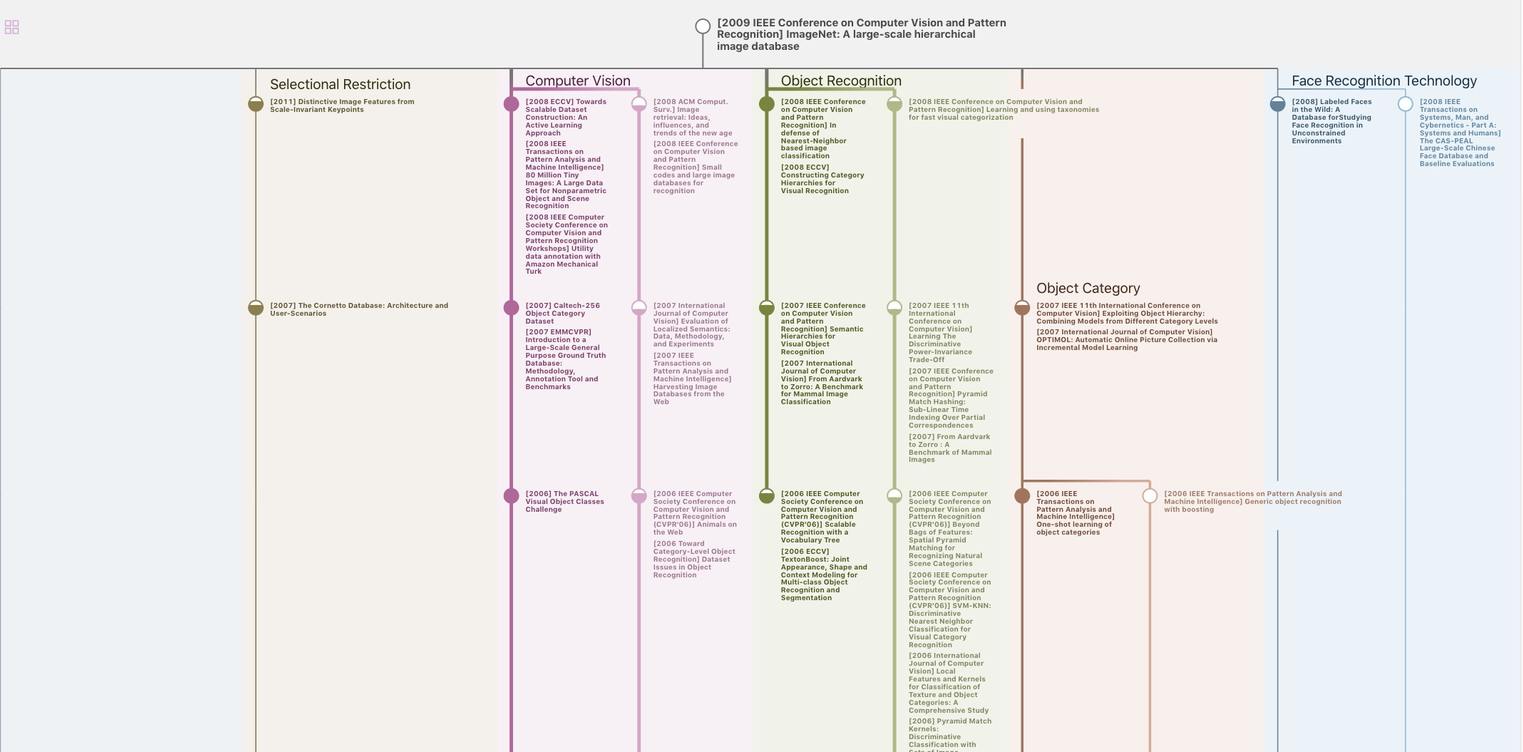
生成溯源树,研究论文发展脉络
Chat Paper
正在生成论文摘要