A hybrid Decoder-DeepONet operator regression framework for unaligned observation data
arXiv (Cornell University)(2023)
Abstract
Deep neural operators (DNOs) have been utilized to approximate nonlinear mappings between function spaces. However, DNOs face the challenge of increased dimensionality and computational cost associated with unaligned observation data. In this study, we propose a hybrid Decoder-DeepONet operator regression framework to handle unaligned data effectively. Additionally, we introduce a Multi-Decoder-DeepONet, which utilizes an average field of training data as input augmentation. The consistencies of the frameworks with the operator approximation theory are provided, on the basis of the universal approximation theorem. Two numerical experiments, Darcy problem and flow-field around an airfoil, are conducted to validate the efficiency and accuracy of the proposed methods. Results illustrate the advantages of Decoder-DeepONet and Multi-Decoder-DeepONet in handling unaligned observation data and showcase their potentials in improving prediction accuracy.
MoreTranslated text
Key words
observation data,regression,decoder-deeponet
AI Read Science
Must-Reading Tree
Example
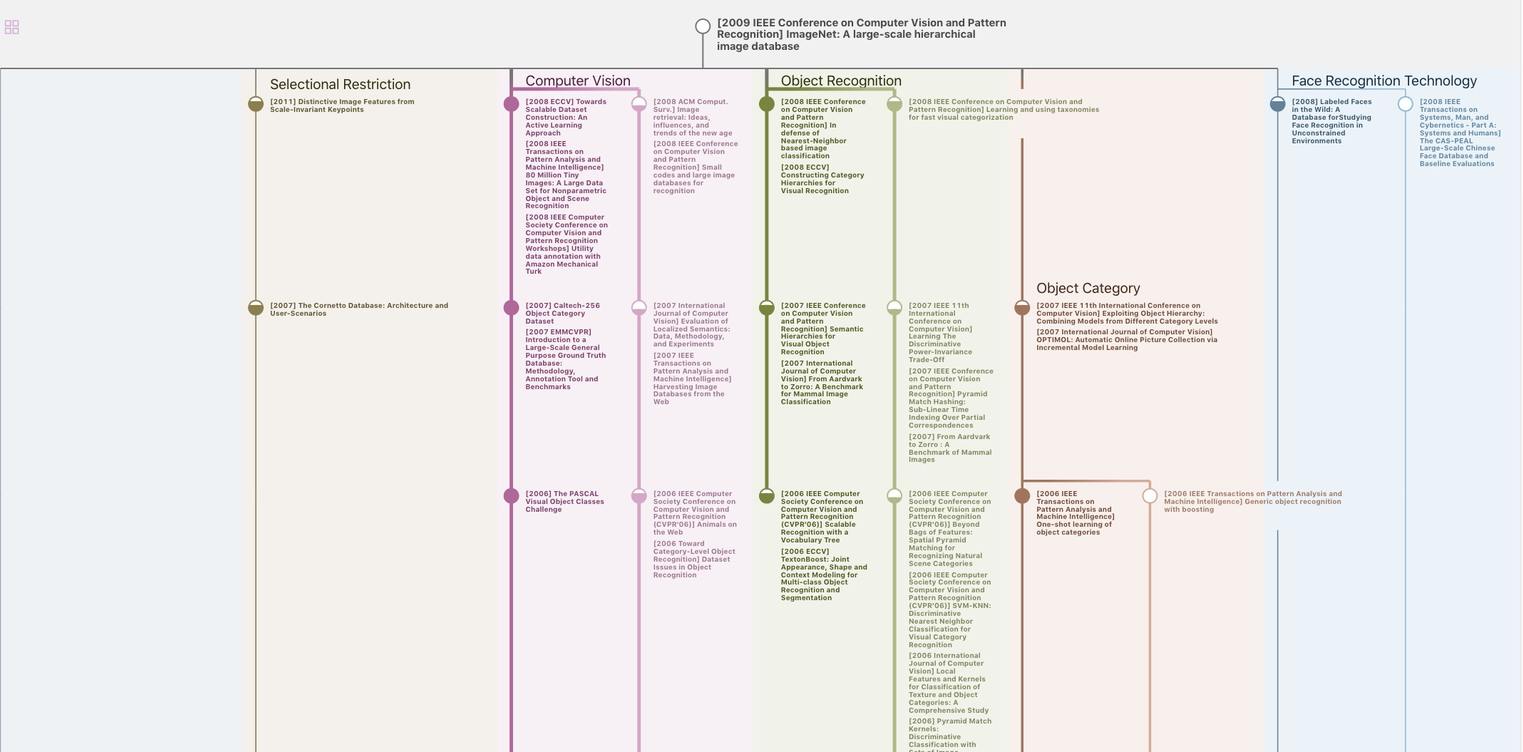
Generate MRT to find the research sequence of this paper
Chat Paper
Summary is being generated by the instructions you defined