Generalized Linear Mixed Models for Proportions and Percentages
Springer eBooks(2023)
摘要
Abstract In this chapter, we will review generalized linear mixed models (GLMMs) whose response can be either a proportion or a percentage. For proportion and percentage data, we refer to data whose expected value is between 0 and 1 or between 0 and 100. For the remainder of this book, we will refer to this type of data only in terms of proportion, knowing that it is possible to change it to a percentage scale only when multiplying it by 100. Proportions can be classified into two types: discrete and continuous. Discrete proportions arise when the unit of observation consists of N distinct entities, of which individuals have the attribute of interest “ y . ” N must be a nonnegative integer and “ y ” must be a positive integer; here, y ≤ N . Therefore, the observed proportion must be a discrete fraction, which can take values $$ \frac{0}{N},\frac{1}{N},\cdots, \frac{N}{N} $$ 0 N , 1 N , ⋯ , N N . A binomial distribution is the sum of a series of m independent binary trials (i.e., trials with only two possible outcomes: success or failure), where all trials have the same probability of success. For binary and binomial distributions, the target of inference is the value of the parameter such that $$ 0\le E\left(\frac{y}{N}\right)=\pi \le 1 $$ 0 ≤ E y N = π ≤ 1 . Continuous proportions (ratios) arise when the researcher measures responses such as the fraction of the area of a leaf infested with a fungus, the proportion of damaged cloth in a square meter, the fraction of a contaminated area, and so on. As with the binomial parameter π , the continuous rates (fractions) take values between 0 and 1, but, unlike the binomial, the continuous proportions do not result from a set of Bernoulli tests. Instead, the beta distribution is most often used when the response variable is in continuous proportions. In the following sections, we will first address issues in modeling when we have binary and binomial data. When the response variable is binomial, we have the option of using a linearization method (pseudo-likelihood (PL)) or the Laplace or quadrature integral approximation (Stroup 2012).
更多查看译文
关键词
linear mixed models,proportions
AI 理解论文
溯源树
样例
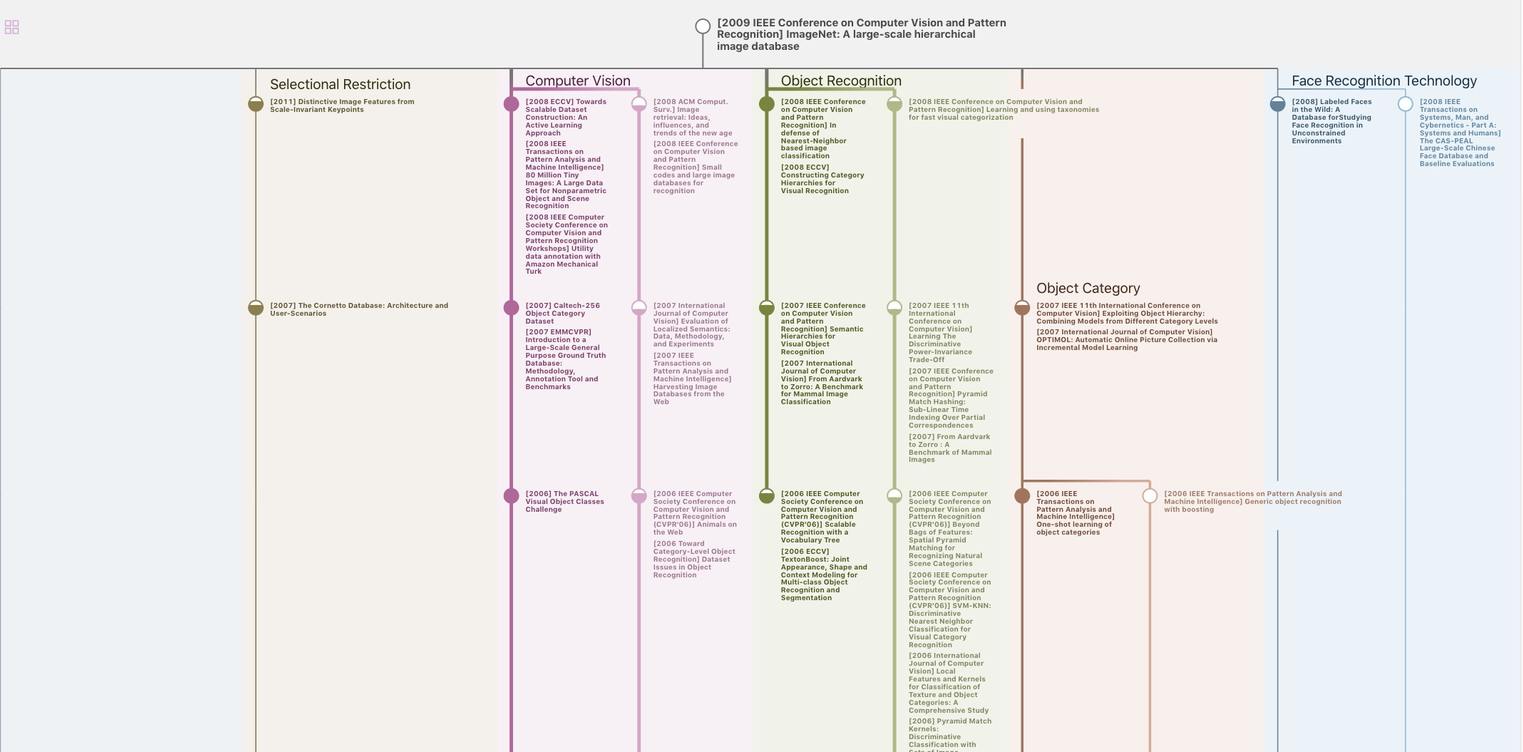
生成溯源树,研究论文发展脉络
Chat Paper
正在生成论文摘要