Self-Completed Bipartite Graph Learning for Fast Incomplete Multi-View Clustering
IEEE TRANSACTIONS ON CIRCUITS AND SYSTEMS FOR VIDEO TECHNOLOGY(2024)
摘要
Incomplete multi-view clustering (IMVC), excavating diversity and consistency from multiple incomplete views, has aroused widespread research enthusiasm. Nevertheless, most existing methods still encounter the following issues: 1) they generally concentrate on pair-wise instance correlation, which consumes at least a quadratic complexity and precludes them from applying at large scales; 2) they only concentrate on pair-wise instance relevance, whereas ignoring the discriminative correlation hidden across views. To overcome these drawbacks, we propose the Self-Completed Bipartite Graph Learning (SCBGL) method for fast IMVC, which adaptively learns a self-completed consensus bipartite graph with the guidance of global information. Specifically, SCBGL learns the consensus anchor matrix shared among diverse views and further constructs a consensus intra-view bipartite graph with missing instances to explore the diversity and complementarity underlying different views. Meanwhile, we concatenate all the multiple features with projection learning to learn global anchors that would be employed to construct an inter-view bipartite graph. Furthermore, SCBGL dexterously utilizes the abundant inter-view information to tutor the self-completion of the consensus intra-view bipartite graph. By devising an alternatively iterative strategy, we present an efficient algorithm, which enjoys a linear time complexity, to solve the proposed SCBGL model. Numerous experiments conducted on large-scale datasets substantiate the superior performance of the SCBGL beyond the state-of-the-arts.
更多查看译文
关键词
Bipartite graph,Kernel,Correlation,Task analysis,Optimization,Excavation,Time complexity,Incomplete multi-view clustering,bipartite graph learning,graph self-completion
AI 理解论文
溯源树
样例
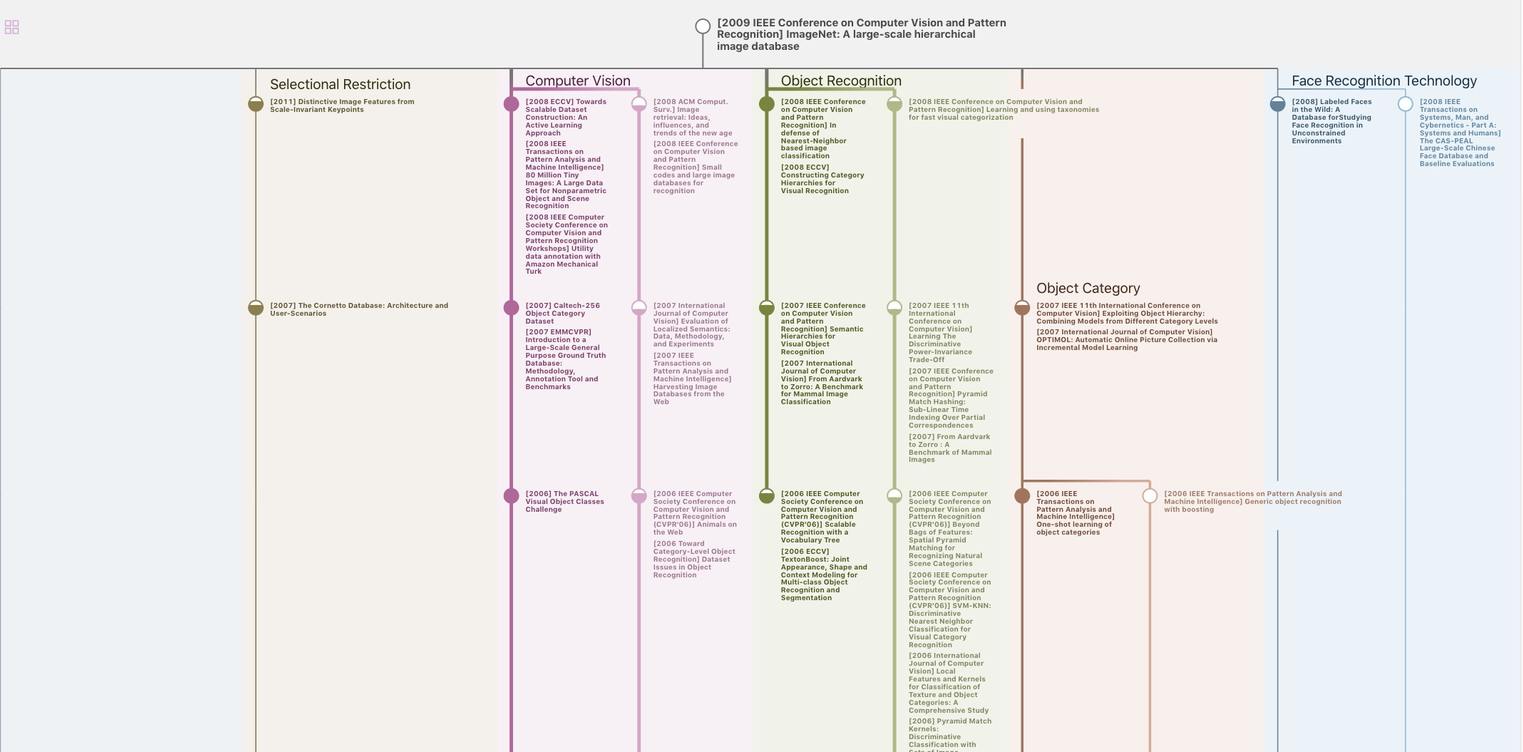
生成溯源树,研究论文发展脉络
Chat Paper
正在生成论文摘要