Reactive Power Flow Convergence Adjustment Based on Deep Reinforcement Learning
Energy Engineering(2023)
摘要
Power flow calculation is the basis of power grid planning and many system analysis tasks require convergent power flow conditions. To address the unsolvable power flow problem caused by the reactive power imbalance, a method for adjusting reactive power flow convergence based on deep reinforcement learning is proposed. The deep reinforcement learning method takes switching parallel reactive compensation as the action space and sets the reward value based on the power flow convergence and reactive power adjustment. For the non-convergence power flow, the 500 kV nodes with reactive power compensation devices on the low-voltage side are converted into PV nodes by node type switching. And the quantified reactive power non-convergence index is acquired. Then, the action space and reward value of deep reinforcement learning are reasonably designed and the adjustment strategy is obtained by taking the reactive power non-convergence index as the algorithm state space. Finally, the effectiveness of the power flow convergence adjustment algorithm is verified by an actual power grid system in a province.
更多查看译文
关键词
deep reinforcement learning,reinforcement learning,flow,power
AI 理解论文
溯源树
样例
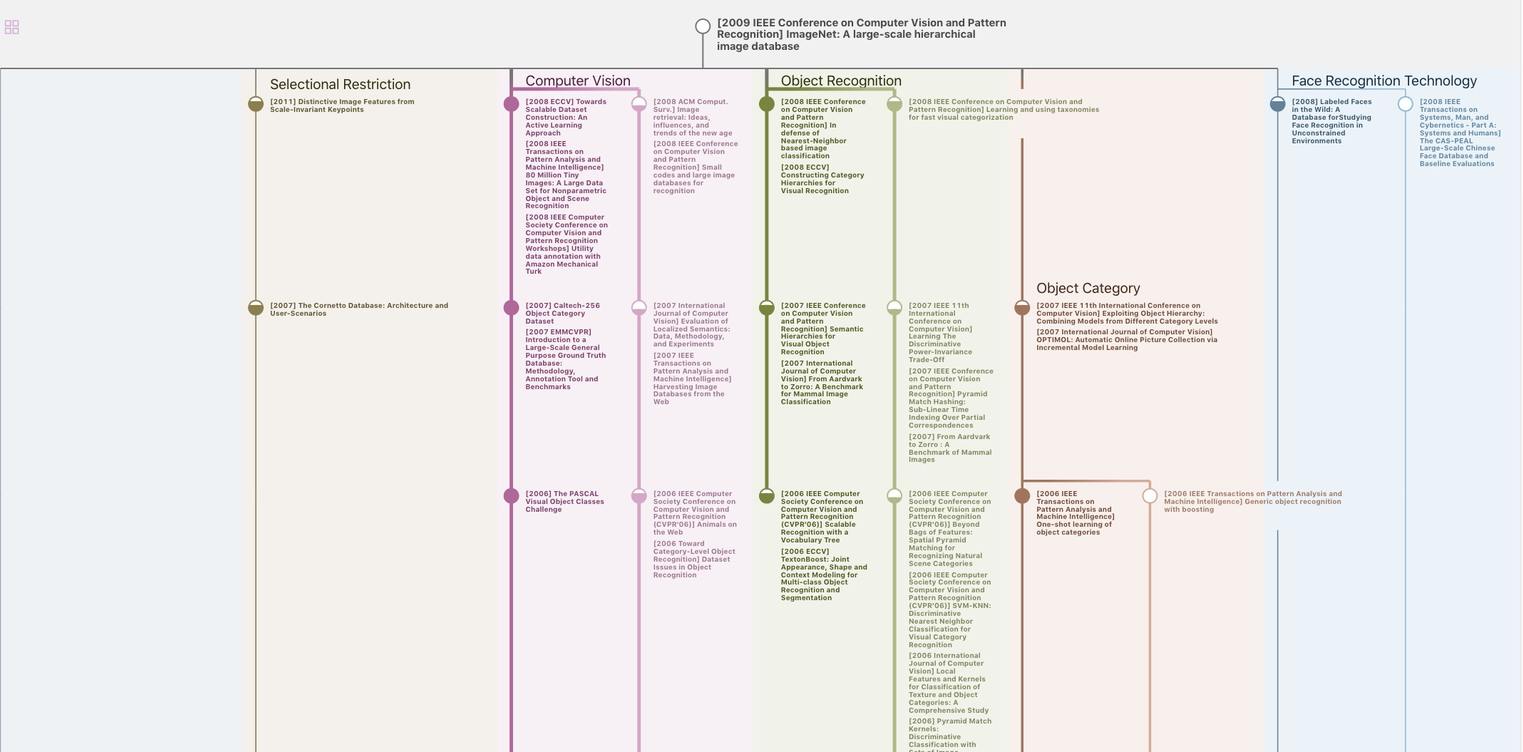
生成溯源树,研究论文发展脉络
Chat Paper
正在生成论文摘要