CPLR-SFS: Contrastive Prompt Learning to Reduce Redundancy for Scientific Faceted Summarization
Journal of physics(2023)
摘要
Abstract Scientific faceted summarization is a task to generate four summaries for a scientific article from different facets, including Purpose, Method, Findings, and Value. Existing works usually generate summary independently for each facet using pre-training or prompt-training paradigms. However, these works tend to produce duplicate content among different facets of the same scientific article, because they do not consider the relation among the aforementioned four facets. To solve the redundancy problem, we propose a novel Contrastive Prompt Learning method to Reduce redundancy for Scientific Faceted Summarization, named CPLR-SFS, to generate concise and less-overlapping faceted summaries. Specifically, CPLR-SFS receives the facet-specific prompt to guide the generation and utilizes the faceted contrastive loss for better distinguishing different faceted summaries in semantic space. Extensive experiments on the FacetSum dataset demonstrate that the proposed model can generate better faceted summaries than the state-of-the-art baselines with less redundancy.
更多查看译文
关键词
contrastive prompt learning,cplr-sfs
AI 理解论文
溯源树
样例
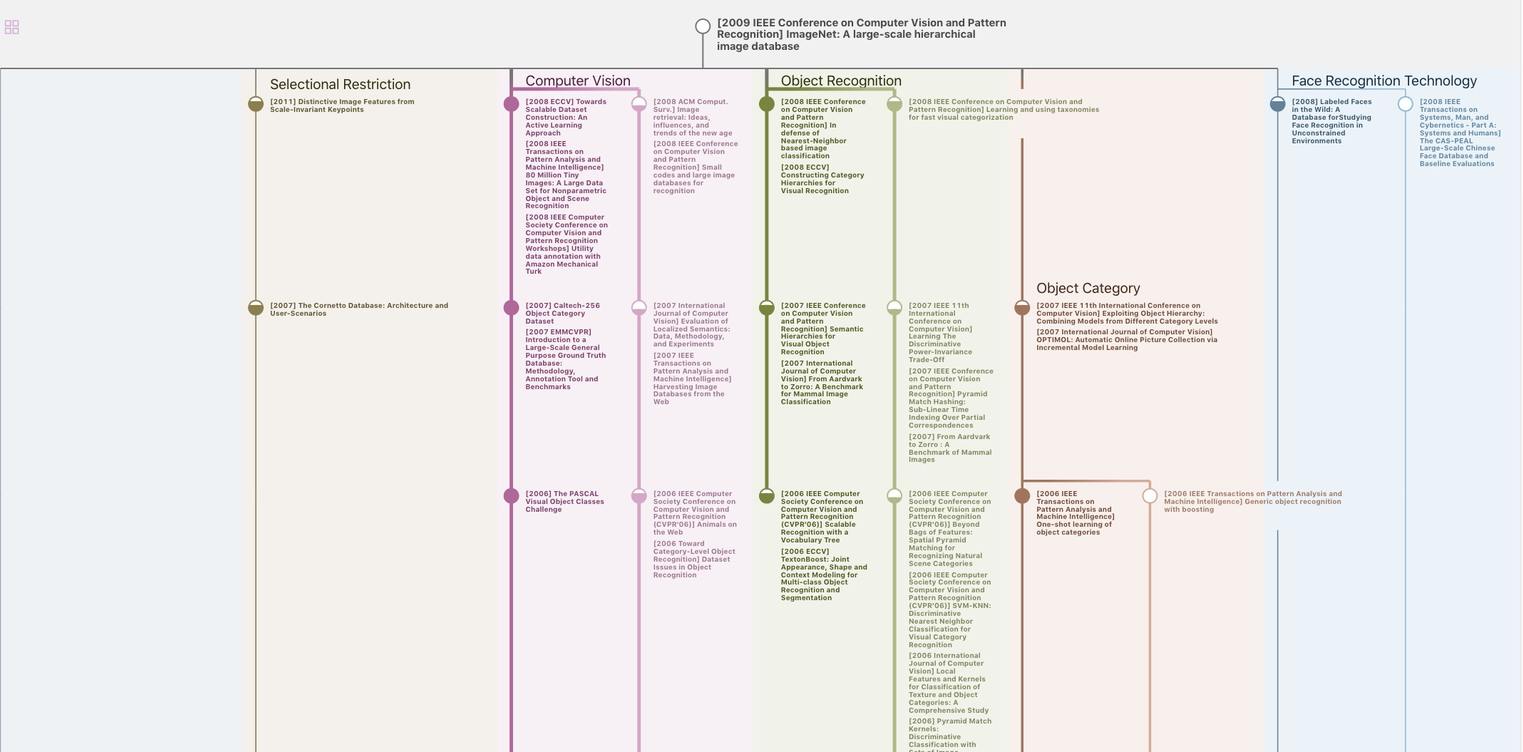
生成溯源树,研究论文发展脉络
Chat Paper
正在生成论文摘要