OncoBERT: Building an Interpretable Transfer Learning Bidirectional Encoder Representations from Transformers Framework for Longitudinal Survival Prediction of Cancer Patients
Research Square (Research Square)(2023)
摘要
Abstract Deep learning transformer models have exhibited exceptional performance in various clinical tasks, including cancer outcome prediction, when applied to electronic health records (EHR). Inspired by the success of bidirectional encoder representations from transformers (BERT) in natural language processing, we present OncoBERT, a deep transformer transfer learning framework tailored for cancer outcome prediction using unstructured clinical notes from diverse cancer sites including Glioma, Prostate, and Breast. OncoBERT adapts the BERT framework for unstructured EHR notes processing and employs a logistic regression model for binary survival prediction. Our framework analyzes aggregated EHR notes for each patient from the time of diagnosis to the initial outcome evaluation timepoint, progressively incorporating new notes for up to one year, which allows us to capture the evolving nature of cancer prognostication. In comparison to stage-based outcome models and a conventional NLP method, OncoBERT outperforms them in predicting cancer outcomes across three distinct cancer types. Furthermore, we introduce a novel interpretability pipeline that allows us to extract clinical note segments identified by the OncoBERT model as the most relevant indicators of prognosis. This pipeline enhances the interpretability of the predictive outcome, surpassing the limitations of attention weights. By providing clearer insights into the model's decision-making process, our approach improves the transparency and comprehensibility of the results, ultimately empowering clinicians and patients to make more informed decisions.
更多查看译文
关键词
longitudinal survival prediction,cancer patients,transformers framework
AI 理解论文
溯源树
样例
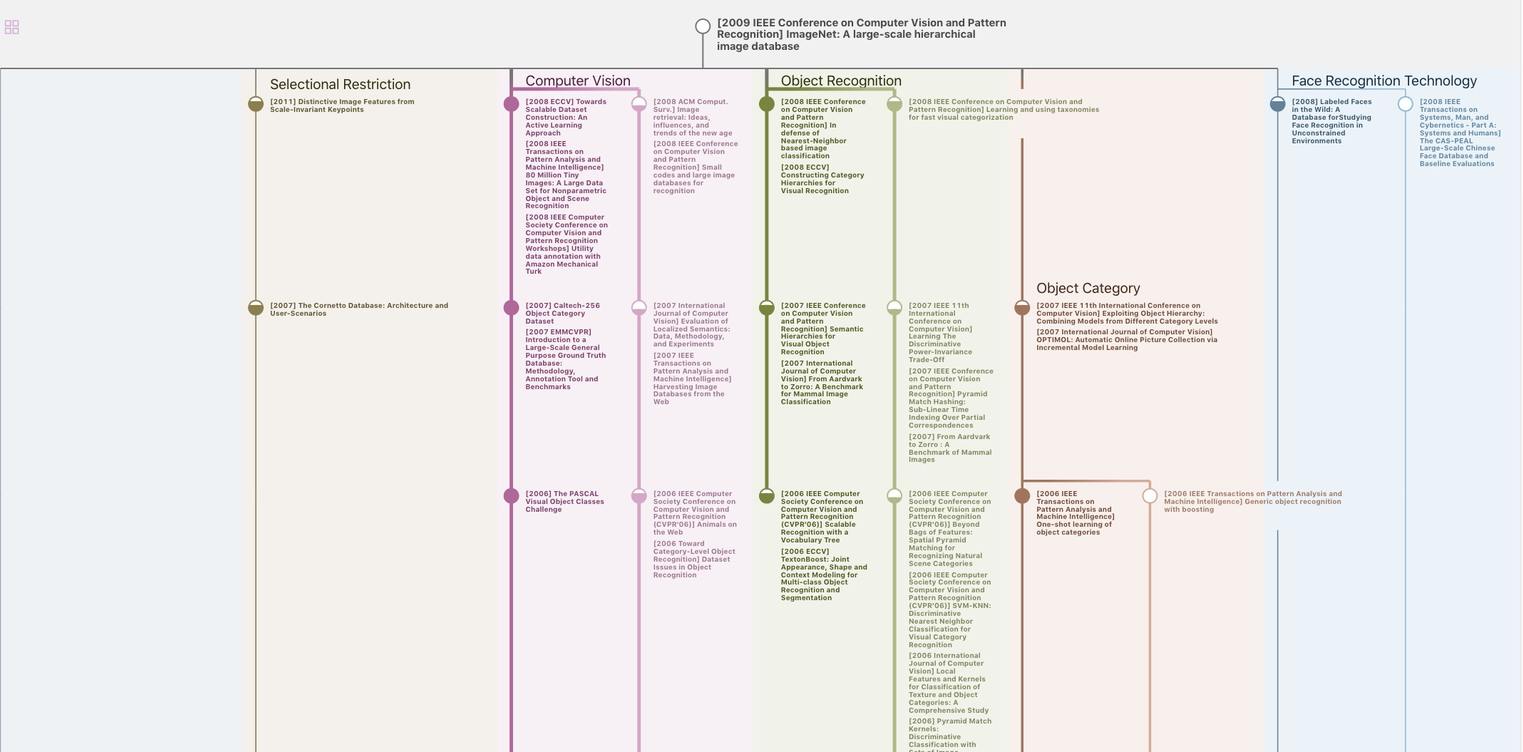
生成溯源树,研究论文发展脉络
Chat Paper
正在生成论文摘要