Secure and Private Healthcare Analytics: A Feasibility Study of Federated Deep Learning with Personal Health Train
Research Square (Research Square)(2023)
摘要
Abstract Objective In this article, we aim to present a new open-source Federated Learning infrastructure by conducting several proof-of-concept experiments. We seek to prove the reliability of the infrastructure to develop global models without sharing private patient data. Materials and Methods We applied the Personal Health Train (PHT) principles using the Vantage6 software to train a neural network to classify head and neck cancer patients’ distant metastasis using federated learning algorithms in a privacy preserving manner. Head and neck cancer patient data from four cohorts were assigned to two data stations. During each training iteration, model weights were averaged and sent back to central node. Results We compared the area under the receiver operating characteristic curves (AUCs) and model weights between the centralized and federated learning scenarios. The results showed that our federated infrastructure was able to achieve similar predicting power as in the centralized case. Different federated learning model weights aggregation methods were tested. The experiment results showed that federated learning models reached best performance when we aggregate model weights per epoch. Discussion and Conclusion PHT and FAIR data principles can efficiently calculate quality indicators in a privacy-preserving federated approach and the work can be scaled up both nationally and internationally. Despite this, application of the methodology was largely hampered by ELSI issues. However, the lessons learned from this study can provide other hospitals and researchers to adapt to the process easily and take effective measures in building quality of care infrastructures.
更多查看译文
关键词
federated deep learning,private healthcare analytics,personal healthcare train,deep learning
AI 理解论文
溯源树
样例
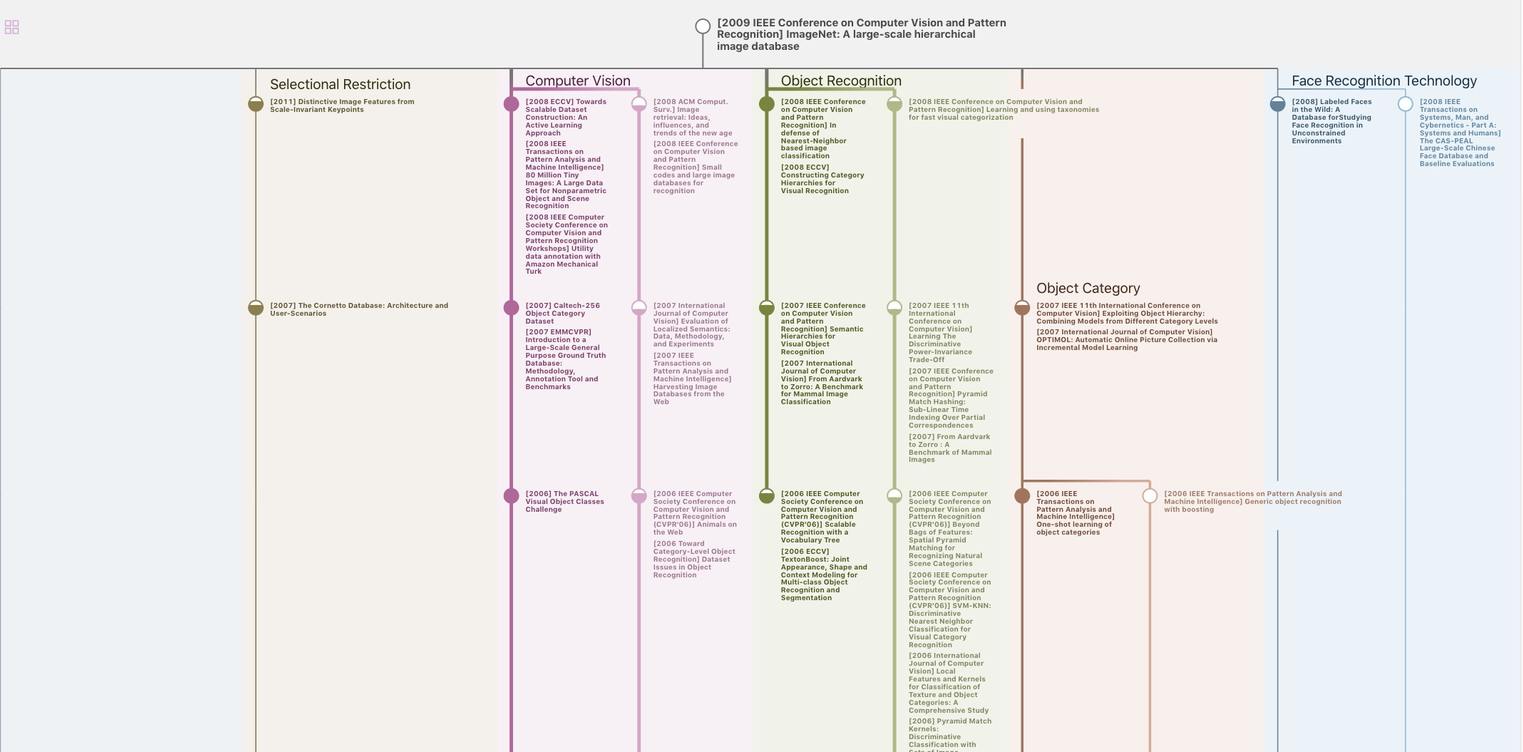
生成溯源树,研究论文发展脉络
Chat Paper
正在生成论文摘要