Information theory based clustering of cellular network usage data for the identification of representative urban areas
Digital Communications and Networks(2023)
摘要
The exponential growth of the number of network devices in recent years not only entails the need for automation of management tasks, but also leads to the increase of available network data and metadata. 5G and beyond standards already cover those requirements and also include the need to define and use machine learning techniques to take advantage of the data acquired, especially using geolocated Call Detail Record (CDR) data sets. However, this scenario requires novel cellular network analysis methodologies to exploit all these available data, especially for the network usage pattern in order to ease the management tasks. In this work, a novel method based on information theory metrics like the Kullback-Leibler divergence and data classification algorithms is proposed to identify representative urban areas in terms of the network usage pattern. Methodology validation is performed via computer analysis using the Open Big Data CDR data set in the Milan area for different scenarios. Obtained results validate the proposed methodology and also reveal its adaptability in terms of specific scenario characteristics. Network usage patterns are calculated for each representative area, paving the path to several future research lines in network management, such as network usage prediction based on this methodology and using the comportment time series.
更多查看译文
关键词
Clustering,5G,Network analysis,Multi-feature analysis,Kullback-Leibler divergence,Cellular networks
AI 理解论文
溯源树
样例
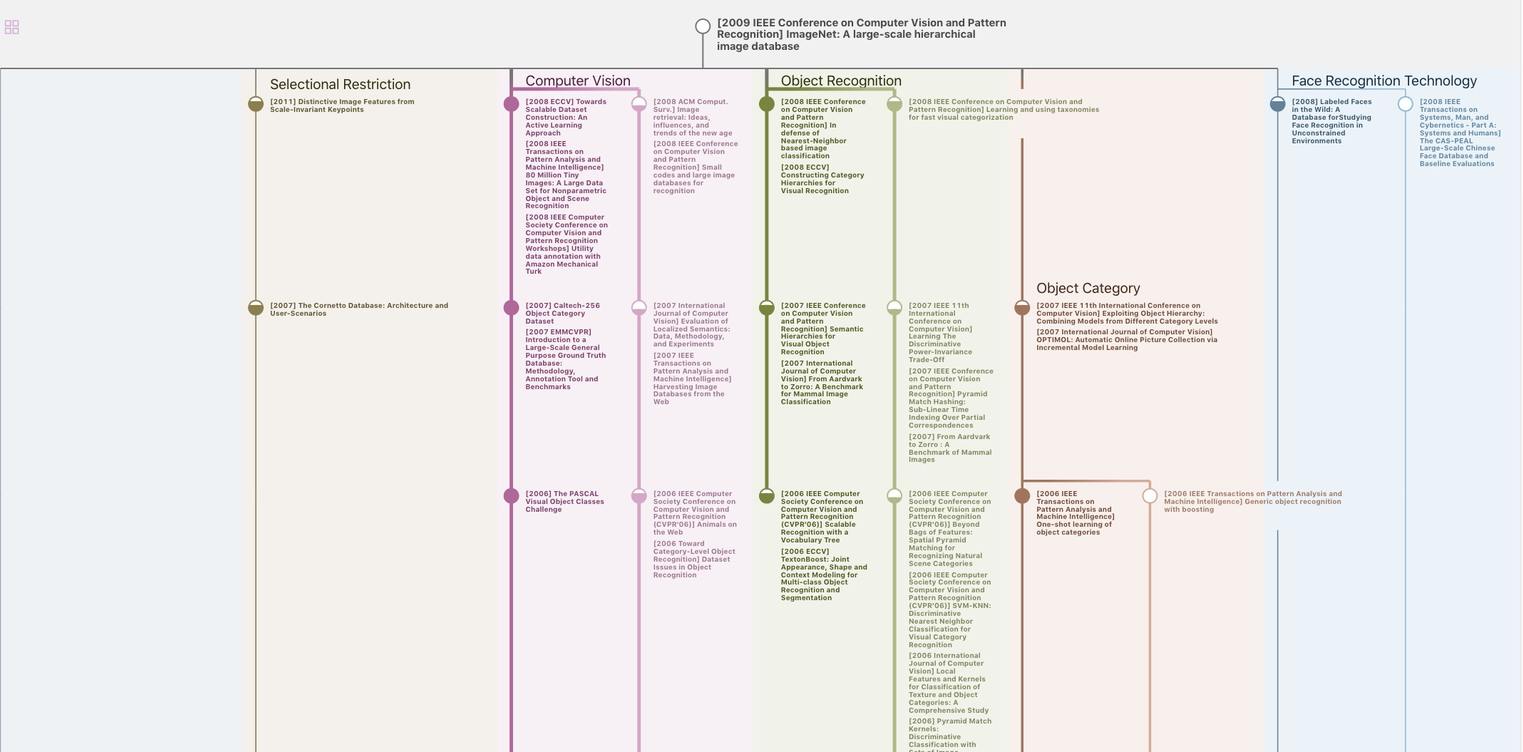
生成溯源树,研究论文发展脉络
Chat Paper
正在生成论文摘要