Time to failure prediction of rotating machinery using dynamic feature extraction and gaussian process regression
The International Journal of Advanced Manufacturing Technology(2024)
Abstract
Recent advances in sensor technology and computing capabilities have enabled the creation of data-driven models that can support real-time decision making. Such a decision aid can allow for predictive maintenance (PdM) to be undertaken on a much greater scale in manufacturing plants. PdM includes data-driven prognostics and health management (PHM). A key element in developing prognostic models involves the acquisition of high-quality data, traditionally achieved through feature extraction methods to distill meaningful insights from extensive and noisy datasets. However, such methods may not handle noisy data well or address measurement errors adequately, potentially resulting in extracted features that inadequately represent the degradation process as a machine approaches failure or fault. Also, effects of sensor types on the feature extraction and prediction model have not been much explored yet. To overcome this limitation, we proposed a solution which involves dynamic feature extraction where a statistical penalty is introduced to mitigate the influence of noisy statistical features within a monotonic trend. Subsequently, the features extracted using this method are utilized to construct a health indicator (HI). Leveraging historical HI values, a probabilistic regression model may be used to forecast the time to failure (TTF) of rotating machinery with uncertainty propagation. To validate the proposed method, acceleration data were collected from rotating machinery for several run-to-failure cases. The proposed method is demonstrated to provide excellent forecasts of TTF for both accelerometer types.
MoreTranslated text
Key words
Time to failure,Dynamic feature extraction,Gaussian process,Uncertainty quantification,Rotating machinery
AI Read Science
Must-Reading Tree
Example
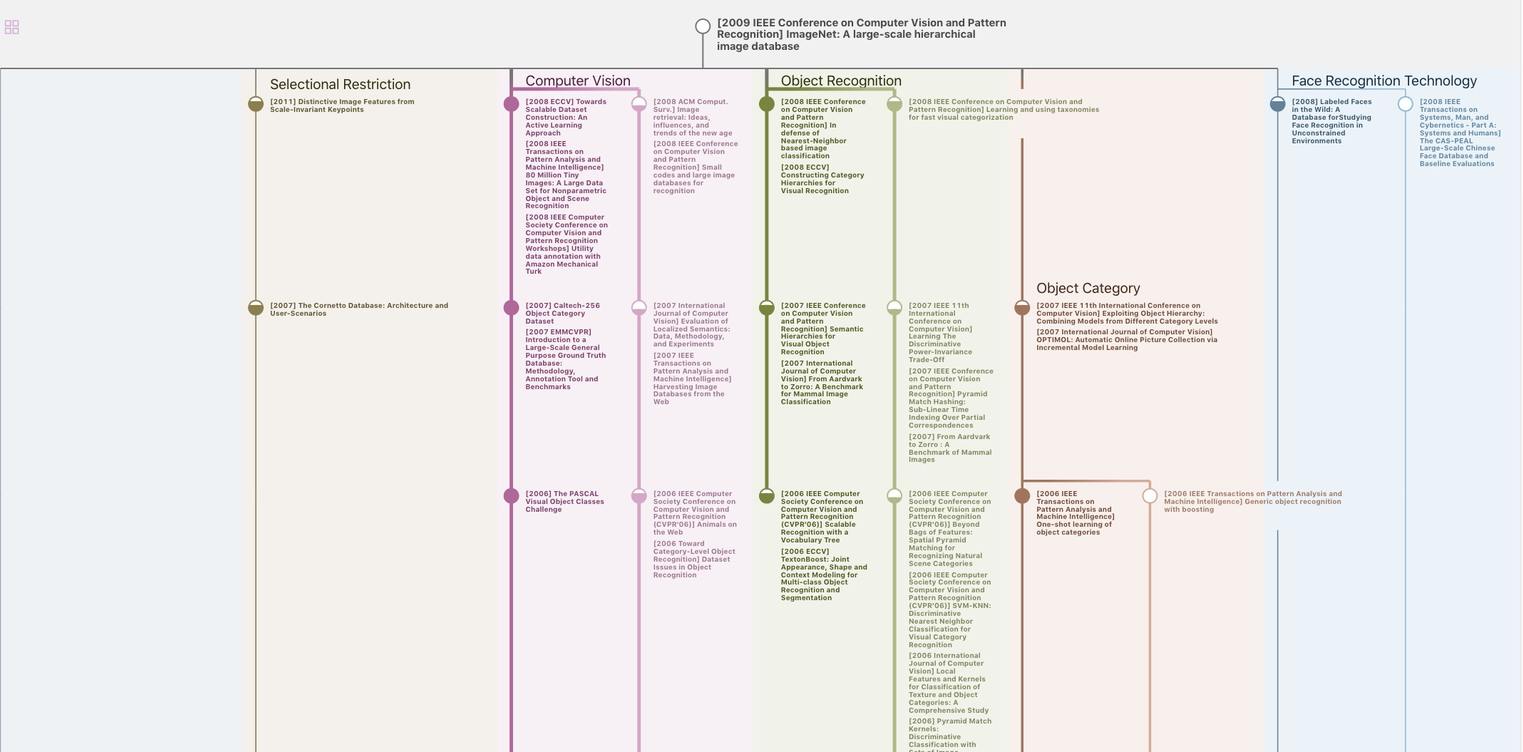
Generate MRT to find the research sequence of this paper
Chat Paper
Summary is being generated by the instructions you defined