SpawnNet: Learning Generalizable Visuomotor Skills from Pre-trained Networks
arXiv (Cornell University)(2023)
摘要
The existing internet-scale image and video datasets cover a wide range of everyday objects and tasks, bringing the potential of learning policies that generalize in diverse scenarios. Prior works have explored visual pre-training with different self-supervised objectives. Still, the generalization capabilities of the learned policies and the advantages over well-tuned baselines remain unclear from prior studies. In this work, we present a focused study of the generalization capabilities of the pre-trained visual representations at the categorical level. We identify the key bottleneck in using a frozen pre-trained visual backbone for policy learning and then propose SpawnNet, a novel two-stream architecture that learns to fuse pre-trained multi-layer representations into a separate network to learn a robust policy. Through extensive simulated and real experiments, we show significantly better categorical generalization compared to prior approaches in imitation learning settings. Open-sourced code and videos can be found on our website: https://xingyu-lin.github.io/spawnnet.
更多查看译文
关键词
generalizable visuomotor skills,learning,networks,pre-trained
AI 理解论文
溯源树
样例
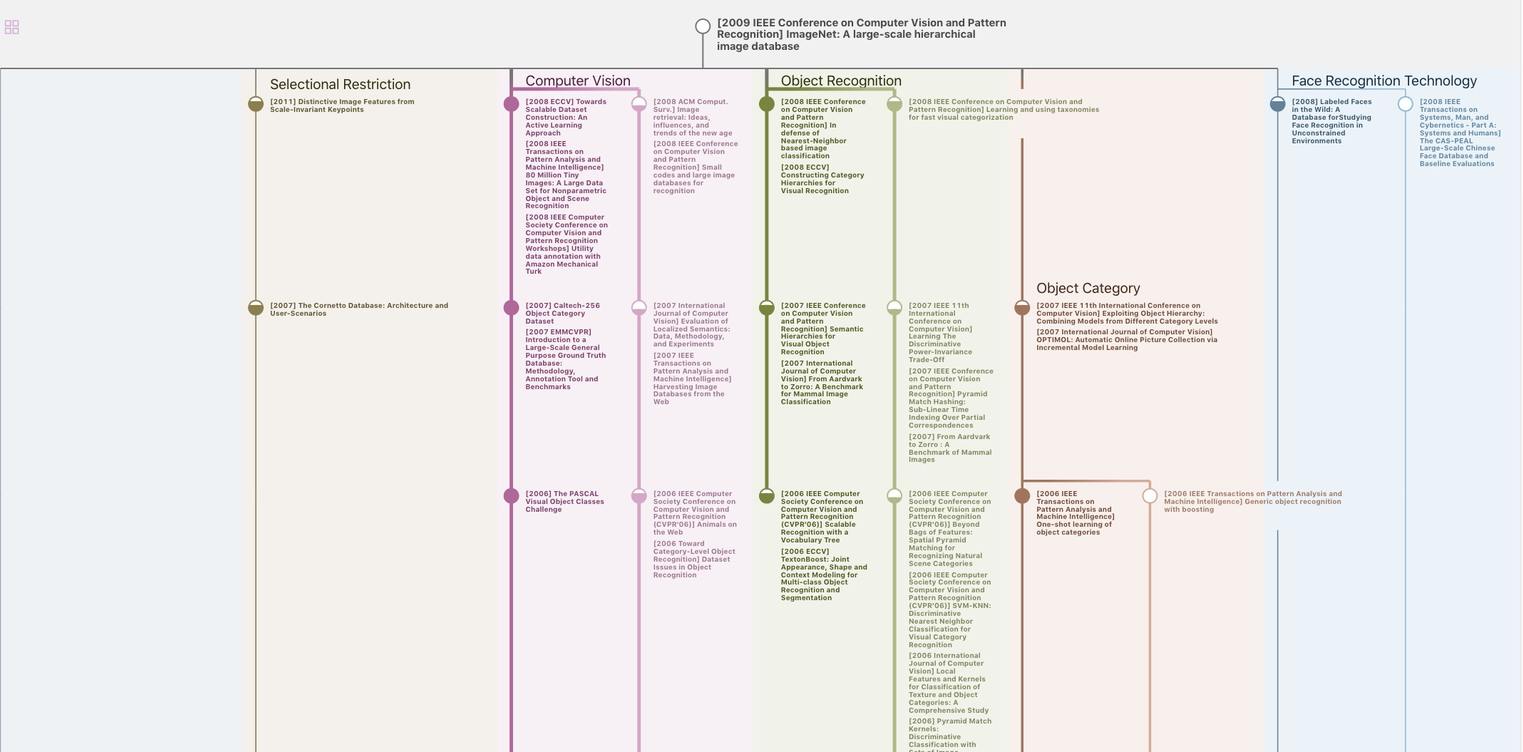
生成溯源树,研究论文发展脉络
Chat Paper
正在生成论文摘要